Copyright notice
Journals
2025
161:111304 May 2025
Few-shot learning investigates how to solve novel tasks given limited labeled data. Exploiting unlabeled data along with the limited labeled has shown substantial improvement in performance. In this work we propose a novel algorithm that exploits unlabeled data in order to improve the performance of few-shot learning. We focus on transductive few-shot inference, where the entire test set is available at inference time, and semi-supervised few-shot learning where unlabeled data are available and can be exploited. Our algorithm starts by leveraging the manifold structure of the labeled and unlabeled data in order to assign accurate pseudo-labels to the unlabeled data. Iteratively, it selects the most confident pseudo-labels and treats them as labeled improving the quality of pseudo-labels at every iteration. Our method surpasses or matches the state of the art results on four benchmark datasets, namely miniImageNet, tieredImageNet, CUB and CIFAR-FS, while being robust over feature pre-processing and the quantity of available unlabeled data. Furthermore, we investigate the setting where the unlabeled data contains data from distractor classes and propose ideas to adapt our algorithm achieving new state of the art performance in the process. Specifically, we utilize the unnormalized manifold class similarities obtained from label propagation for pseudo-label cleaning and exploit the uneven pseudo-label distribution between classes to remove noisy data. The publicly available source code can be found at https://github.com/MichalisLazarou/iLPC.
@article{J34,
title = {Exploiting unlabeled data in few-shot learning},
author = {Lazarou, Michalis and Stathaki, Tania and Avrithis, Yannis},
journal = {Pattern Recognition (PR)},
volume = {161},
pages = {111304},
month = {5},
year = {2025}
}
2024
32:5010-5023 Nov 2024
Multimodal sentiment analysis (MSA) leverages heterogeneous data sources to interpret the complex nature of human sentiments. Despite significant progress in multimodal architecture design, the field lacks comprehensive regularization methods. This paper introduces PowMix, a versatile embedding space regularizer that builds upon the strengths of unimodal mixing-based regularization approaches and introduces novel algorithmic components that are specifically tailored to multimodal tasks. PowMix is integrated before the fusion stage of multimodal architectures and facilitates intra-modal mixing, such as mixing text with text, to act as a regularizer. PowMix consists of five components: 1) a varying number of generated mixed examples, 2) mixing factor reweighting, 3) anisotropic mixing, 4) dynamic mixing, and 5) cross-modal label mixing. Extensive experimentation across benchmark MSA datasets and a broad spectrum of diverse architectural designs demonstrate the efficacy of PowMix, as evidenced by consistent performance improvements over baselines and existing mixing methods. An in-depth ablation study highlights the critical contribution of each PowMix component and how they synergistically enhance performance. Furthermore, algorithmic analysis demonstrates how PowMix behaves in different scenarios, particularly comparing early versus late fusion architectures. Notably, PowMix enhances overall performance without sacrificing model robustness or magnifying text dominance. It also retains its strong performance in situations of limited data. Our findings position PowMix as a promising versatile regularization strategy for MSA.
@article{J33,
title = {{PowMix}: A Versatile Regularizer for Multimodal Sentiment Analysis},
author = {Georgiou, Efthymios and Avrithis, Yannis and Potamianos, Alexandros},
journal = {IEEE Transactions on Audio, Speech and Language Processing (TASL)},
volume = {32},
pages = {5010--5023},
month = {11},
year = {2024}
}
248:104101 Nov 2024
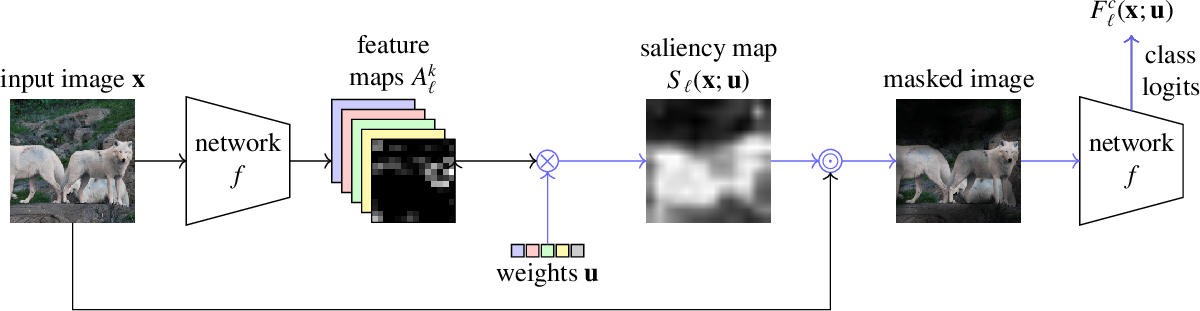
Methods based on class activation maps (CAM) provide a simple mechanism to interpret predictions of convolutional neural networks by using linear combinations of feature maps as saliency maps. By contrast, masking-based methods optimize a saliency map directly in the image space or learn it by training another network on additional data.
In this work we introduce Opti-CAM, combining ideas from CAM-based and masking-based approaches. Our saliency map is a linear combination of feature maps, where weights are optimized per image such that the logit of the masked image for a given class is maximized. We also fix a fundamental flaw in two of the most common evaluation metrics of attribution methods. On several datasets, Opti-CAM largely outperforms other CAM-based approaches according to the most relevant classification metrics. We provide empirical evidence supporting that localization and classifier interpretability are not necessarily aligned.
@article{J32,
title = {Opti-{CAM}: Optimizing saliency maps for interpretability},
author = {Zhang, Hanwei and Torres, Felipe and Sicre, Ronan and Avrithis, Yannis and Ayache, Stephane},
journal = {Computer Vision and Image Understanding (CVIU)},
volume = {248},
pages = {104101},
month = {11},
year = {2024}
}
2021
120:108-164 Dec 2021
Weakly-supervised object detection attempts to limit the amount of supervision by dispensing the need for bounding boxes, but still assumes image-level labels on the entire training set. In this work, we study the problem of training an object detector from one or few images with image-level labels and a larger set of completely unlabeled images. This is an extreme case of semi-supervised learning where the labeled data are not enough to bootstrap the learning of a detector. Our solution is to train a weakly-supervised student detector model from image-level pseudo-labels generated on the unlabeled set by a teacher classifier model, bootstrapped by region-level similarities to labeled images. Building upon the recent representative weakly-supervised pipeline PCL, our method can use more unlabeled images to achieve performance competitive or superior to many recent weakly-supervised detection solutions.
@article{J31,
title = {Training Object Detectors from Few Weakly-Labeled and Many Unlabeled Images},
author = {Yang, Zhaohui and Shi, Miaojing and Xu, Chao and Ferrari, Vittorio and Avrithis, Yannis},
journal = {Pattern Recognition (PR)},
volume = {120},
pages = {108--164},
month = {12},
year = {2021}
}
16:701-713 Sep 2021
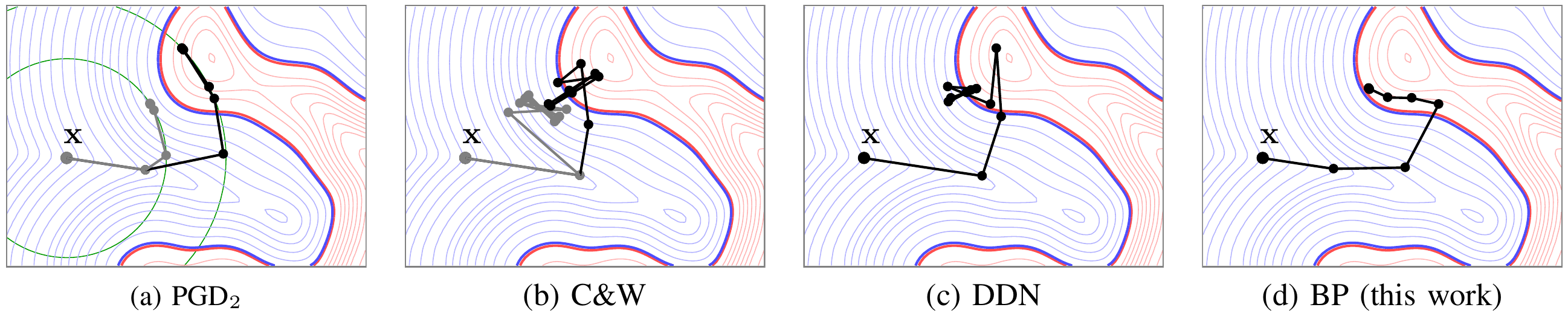
Adversarial examples of deep neural networks are receiving ever increasing attention because they help in understanding and reducing the sensitivity to their input. This is natural given the increasing applications of deep neural networks in our everyday lives. When white-box attacks are almost always successful, it is typically only the distortion of the perturbations that matters in their evaluation. In this work, we argue that speed is important as well, especially when considering that fast attacks are required by adversarial training. Given more time, iterative methods can always find better solutions. We investigate this speed-distortion trade-off in some depth and introduce a new attack called boundary projection (BP) that improves upon existing methods by a large margin. Our key idea is that the classification boundary is a manifold in the image space: we therefore quickly reach the boundary and then optimize distortion on this manifold.
@article{J30,
title = {Walking on the Edge: Fast, Low-Distortion Adversarial Examples},
author = {Zhang, Hanwei and Avrithis, Yannis and Furon, Teddy and Amsaleg, Laurent},
journal = {IEEE Transactions on Information Forensics and Security (TIFS)},
volume = {16},
pages = {701--713},
month = {9},
year = {2021}
}
2020
2020:15-26 Nov 2020
This paper investigates the visual quality of the adversarial examples. Recent papers propose to smooth the perturbations to get rid of high frequency artefacts. In this work, smoothing has a different meaning as it perceptually shapes the perturbation according to the visual content of the image to be attacked. The perturbation becomes locally smooth on the flat areas of the input image, but it may be noisy on its textured areas and sharp across its edges.
This operation relies on Laplacian smoothing, well-known in graph signal processing, which we integrate in the attack pipeline. We benchmark several attacks with and without smoothing under a white-box scenario and evaluate their transferability. Despite the additional constraint of smoothness, our attack has the same probability of success at lower distortion.
@article{J29,
title = {Smooth Adversarial Examples},
author = {Zhang, Hanwei and Avrithis, Yannis and Furon, Teddy and Amsaleg, Laurent},
journal = {EURASIP Journal on Information Security (JIS)},
volume = {2020},
pages = {15--26},
month = {11},
year = {2020}
}
2019
30(2):243-254 Mar 2019
Severe background clutter is challenging in many computer vision tasks, including large-scale image retrieval. Global descriptors, that are popular due to their memory and search efficiency, are especially prone to corruption by such a clutter. Eliminating the impact of the clutter on the image descriptor increases the chance of retrieving relevant images and prevents topic drift due to actually retrieving the clutter in the case of query expansion. In this work, we propose a novel salient region detection method. It captures, in an unsupervised manner, patterns that are both discriminative and common in the dataset. Saliency is based on a centrality measure of a nearest neighbor graph constructed from regional CNN representations of dataset images. The proposed method exploits recent CNN architectures trained for object retrieval to construct the image representation from the salient regions. We improve particular object retrieval on challenging datasets containing small objects.
@article{J28,
title = {Graph-based Particular Object Discovery},
author = {Sim\'eoni, Oriane and Iscen, Ahmet and Tolias, Giorgos and Avrithis, Yannis and Chum, Ond\v{r}ej},
journal = {Machine Vision and Applications (MVA)},
volume = {30},
number = {2},
month = {3},
pages = {243--254},
year = {2019}
}
179:66-78 Feb 2019
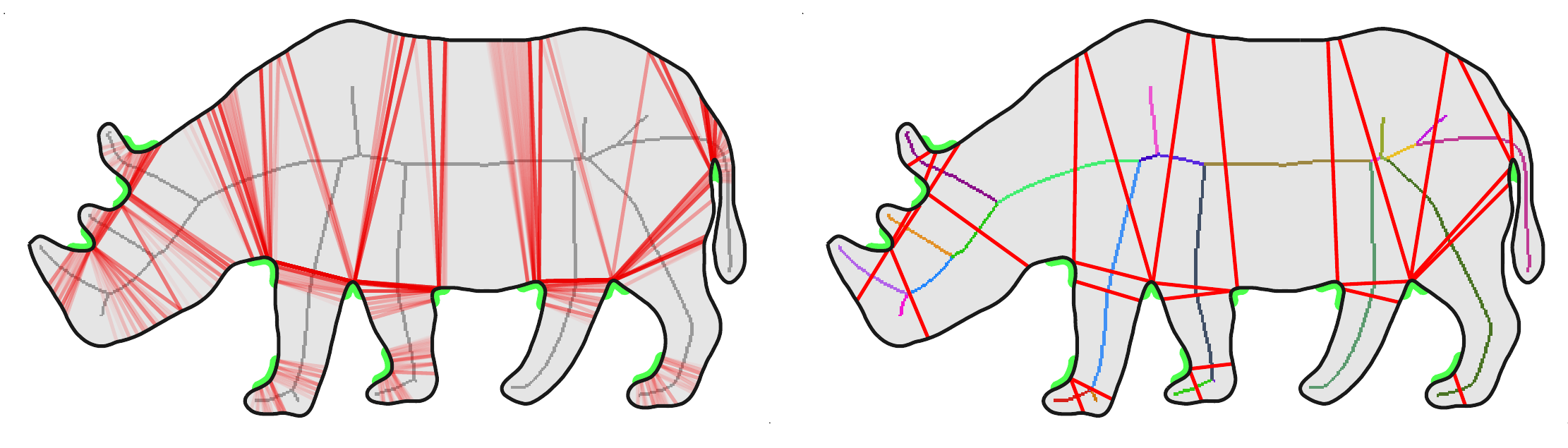
We present a simple computational model for planar shape decomposition that naturally captures most of the rules and salience measures suggested by psychophysical studies, including the minima and short-cut rules, convexity, and symmetry. It is based on a medial axis representation in ways that have not been explored before and sheds more light into the connection between existing rules like minima and convexity. In particular, vertices of the exterior medial axis directly provide the position and extent of negative minima of curvature, while a traversal of the interior medial axis directly provides a small set of candidate endpoints for part-cuts. The final selection follows a prioritized processing of candidate part-cuts according to a local convexity rule that can incorporate arbitrary salience measures. Neither global optimization nor differentiation is involved. We provide qualitative and quantitative evaluation and comparisons on ground-truth data from psychophysical experiments. With our single computational model, we outperform even an ensemble method on several other competing models.
@article{J27,
title = {Revisiting the Medial Axis for Planar Shape Decomposition},
author = {Papanelopoulos, Nikos and Avrithis, Yannis and Kollias, Stefanos},
journal = {Computer Vision and Image Understanding (CVIU)},
volume = {179},
month = {2},
pages = {66--78},
year = {2019}
}
2016
50(1):56-73 Feb 2016
Local image features are routinely used in state-of-the-art methods to solve many computer vision problems like image retrieval, classification, or 3D registration. As the applications become more complex, the research for better visual features is still active. In this paper we present a feature detector that exploits the inherent geometry of sampled image edges using α-shapes. We propose a novel edge sampling scheme that exploits local shape and investigate different triangulations of sampled points. We also introduce a novel approach to represent the anisotropy in a triangulation along with different feature selection methods. Our detector provides a small number of distinctive features that is ideal for large scale applications, while achieving competitive performance in a series of matching and retrieval experiments.
@article{J26,
title = {$\alpha$-shapes for local feature detection},
author = {Varytimidis, Christos and Rapantzikos, Konstantinos and Avrithis, Yannis and Kollias, Stefanos},
journal = {Pattern Recognition (PR)},
volume = {50},
number = {1},
month = {2},
pages = {56--73},
year = {2016}
}
116(3):247-261 Feb 2016
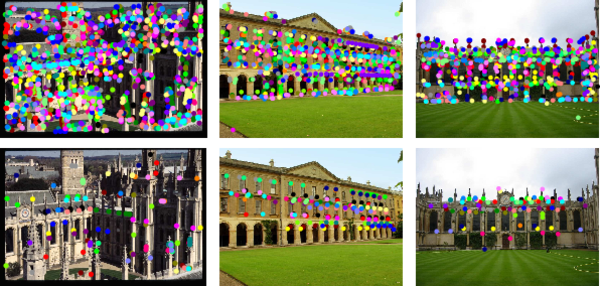
This paper considers a family of metrics to compare images based on their local descriptors. It encompasses the VLAD descriptor and matching techniques such as Hamming Embedding. Making the bridge between these approaches leads us to propose a match kernel that takes the best of existing techniques by combining an aggregation procedure with a selective match kernel. The representation underpinning this kernel is approximated, providing a large scale image search both precise and scalable, as shown by our experiments on several benchmarks.
We show that the same aggregation procedure, originally applied per image, can effectively operate on groups of similar features found across multiple images. This method implicitly performs feature set augmentation, while enjoying savings in memory requirements at the same time. Finally, the proposed method is shown effective for place recognition, outperforming state of the art methods on a large scale landmark recognition benchmark.
@article{J25,
title = {Image search with selective match kernels: aggregation across single and multiple images},
author = {Tolias, Giorgos and Avrithis, Yannis and J\'egou, Herv\'e},
journal = {International Journal of Computer Vision (IJCV)},
volume = {116},
number = {3},
month = {2},
pages = {247--261},
year = {2016}
}
2015
7(1):189-200 Dec 2015
Local feature detection has been an essential part of many methods for computer vision applications like large scale image retrieval, object detection, or tracking. Recently, structure-guided feature detectors have been proposed, exploiting image edges to accurately capture local shape. Among them, the WαSH detector [Varytimidis et al., 2012] starts from sampling binary edges and exploits α-shapes, a computational geometry representation that describes local shape in different scales. In this work, we propose a novel image sampling method, based on dithering smooth image functions other than intensity. Samples are extracted on image contours representing the underlying shapes, with sampling density determined by image functions like the gradient or Hessian response, rather than being fixed. We thoroughly evaluate the parameters of the method, and achieve state-of-the-art performance on a series of matching and retrieval experiments.
@article{J24,
title = {Dithering-based Sampling and Weighted $\alpha$-shapes for Local Feature Detection},
author = {Varytimidis, Christos and Rapantzikos, Konstantinos and Avrithis, Yannis and Kollias, Stefanos},
journal = {IPSJ Transactions on Computer Vision and Applications (CVA)},
publisher = {Information Processing Society of Japan},
volume = {7},
number = {1},
month = {12},
pages = {189--200},
year = {2015}
}
2014
120:31-45 Mar 2014
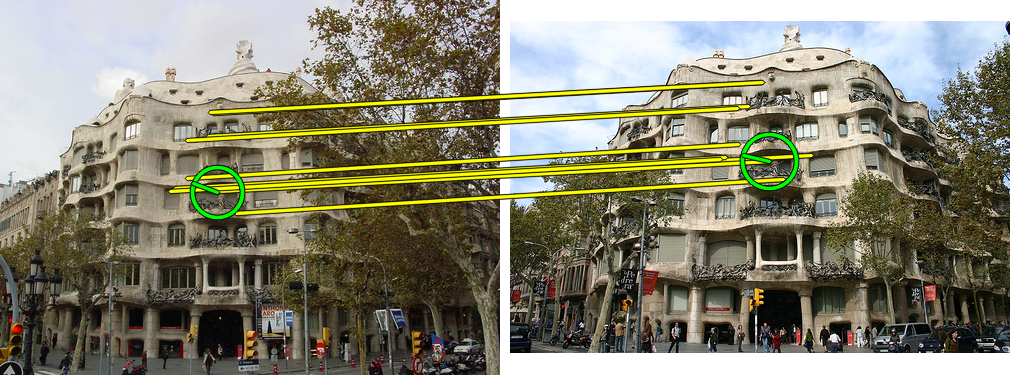
We present a new approach to image indexing and retrieval, which integrates appearance with global image geometry in the indexing process, while enjoying robustness against viewpoint change, photometric variations, occlusion, and background clutter. We exploit shape parameters of local features to estimate image alignment via a single correspondence. Then, for each feature, we construct a sparse spatial map of all remaining features, encoding their normalized position and appearance, typically vector quantized to visual word. An image is represented by a collection of such feature maps and RANSAC-like matching is reduced to a number of set intersections. The required index space is still quadratic in the number of features. To make it linear, we propose a novel feature selection model tailored to our feature map representation, replacing our earlier hashing approach. The resulting index space is comparable to baseline bag-of-words, scaling up to one million images while outperforming the state of the art on three publicly available datasets. To our knowledge, this is the first geometry indexing method to dispense with spatial verification at this scale, bringing query times down to milliseconds.
@article{J23,
title = {Towards large-scale geometry indexing by feature selection},
author = {Tolias, Giorgos and Kalantidis, Yannis and Avrithis, Yannis and Kollias, Stefanos},
journal = {Computer Vision and Image Understanding (CVIU)},
volume = {120},
pages = {31--45},
month = {03},
year = {2014}
}
107(1):1-19 Mar 2014
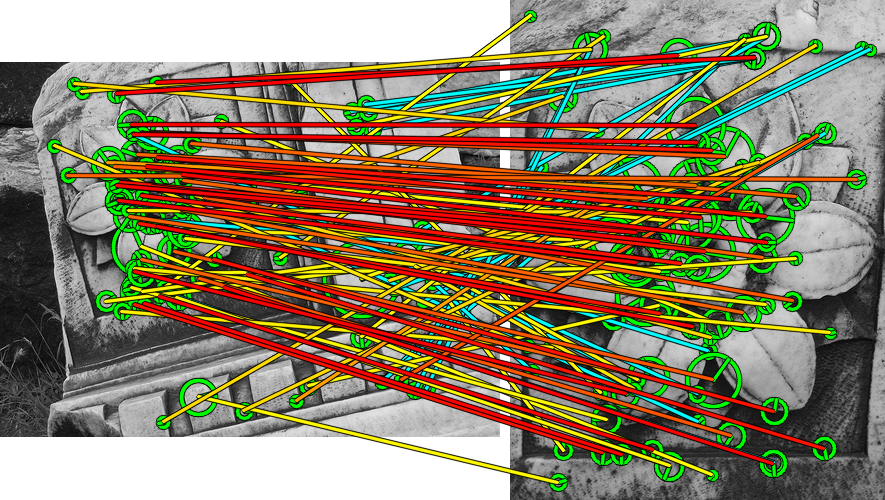
Exploiting local feature shape has made geometry indexing possible, but at a high cost of index space, while a sequential spatial verification and re-ranking stage is still indispensable for large scale image retrieval. In this work we investigate an accelerated approach for the latter problem. We develop a simple spatial matching model inspired by Hough voting in the transformation space, where votes arise from single feature correspondences. Using a histogram pyramid, we effectively compute pair-wise affinities of correspondences without ever enumerating all pairs. Our Hough pyramid matching algorithm is linear in the number of correspondences and allows for multiple matching surfaces or non-rigid objects under one-to-one mapping. We achieve re-ranking one order of magnitude more images at the same query time with superior performance compared to state of the art methods, while requiring the same index space. We show that soft assignment is compatible with this matching scheme, preserving one-to-one mapping and further increasing performance.
@article{J22,
title = {Hough Pyramid Matching: Speeded-up geometry re-ranking for large scale image retrieval},
author = {Avrithis, Yannis and Tolias, Giorgos},
journal = {International Journal of Computer Vision (IJCV)},
volume = {107},
number = {1},
month = {3},
pages = {1--19},
year = {2014}
}
2013
15(7):1553-1568 Nov 2013
Multimodal streams of sensory information are naturally parsed and integrated by humans using signal-level feature extraction and higher-level cognitive processes. Detection of attention-invoking audiovisual segments is formulated in this work on the basis of saliency models for the audio, visual and textual information conveyed in a video stream. Aural or auditory saliency is assessed by cues that quantify multifrequency waveform modulations, extracted through nonlinear operators and energy tracking. Visual saliency is measured through a spatiotemporal attention model driven by intensity, color and orientation. Textual or linguistic saliency is extracted from part-of-speech tagging on the subtitles information available with most movie distributions. The individual saliency streams, obtained from modality-depended cues, are integrated in a multimodal saliency curve, modeling the time-varying perceptual importance of the composite video stream and signifying prevailing sensory events. The multimodal saliency representation forms the basis of a generic, bottom-up video summarization algorithm. Different fusion schemes are evaluated on a movie database of multimodal saliency annotations with comparative results provided across modalities. The produced summaries, based on low-level features and content-independent fusion and selection, are of subjectively high aesthetic and informative quality.
@article{J21,
title = {Multimodal Saliency and Fusion for Movie Summarization based on Aural, Visual, and Textual Attention},
author = {Evangelopoulos, Georgios and Zlatintsi, Athanasia and Potamianos, Alexandros and Maragos, Petros and Rapantzikos, Konstantinos and Skoumas, Georgios and Avrithis, Yannis},
journal = {IEEE Transactions on Multimedia (TMM)},
volume = {15},
number = {7},
month = {11},
pages = {1553--1568},
year = {2013}
}
2011
Special issue on Saliency, Attention, Visual Search and Picture Scanning
3(1):167-184 Mar 2011
Although the mechanisms of human visual understanding remain partially unclear, computational models inspired by existing knowledge on human vision have emerged and applied to several fields. In this paper, we propose a novel method to compute visual saliency from video sequences by counting in the actual spatiotemporal nature of the video. The visual input is represented by a volume in space–time and decomposed into a set of feature volumes in multiple resolutions. Feature competition is used to produce a saliency distribution of the input implemented by constrained minimization. The proposed constraints are inspired by and associated with the Gestalt laws. There are a number of contributions in this approach, namely extending existing visual feature models to a volumetric representation, allowing competition across features, scales and voxels, and formulating constraints in accordance with perceptual principles. The resulting saliency volume is used to detect prominent spatiotemporal regions and consequently applied to action recognition and perceptually salient event detection in video sequences. Comparisons against established methods on public datasets are given and reveal the potential of the proposed model. The experiments include three action recognition scenarios and salient temporal segment detection in a movie database annotated by humans.
@article{J20,
title = {Spatiotemporal features for action recognition and salient event detection},
author = {Rapantzikos, Konstantinos and Avrithis, Yannis and Kollias, Stefanos},
journal = {Cognitive Computation (CC) (Special Issue on Saliency, Attention, Visual Search and Picture Scanning)},
editor = {J.G. Taylor and V. Cutsuridis},
volume = {3},
number = {1},
month = {3},
pages = {167--184},
year = {2011}
}
51(2):555-592 Jan 2011
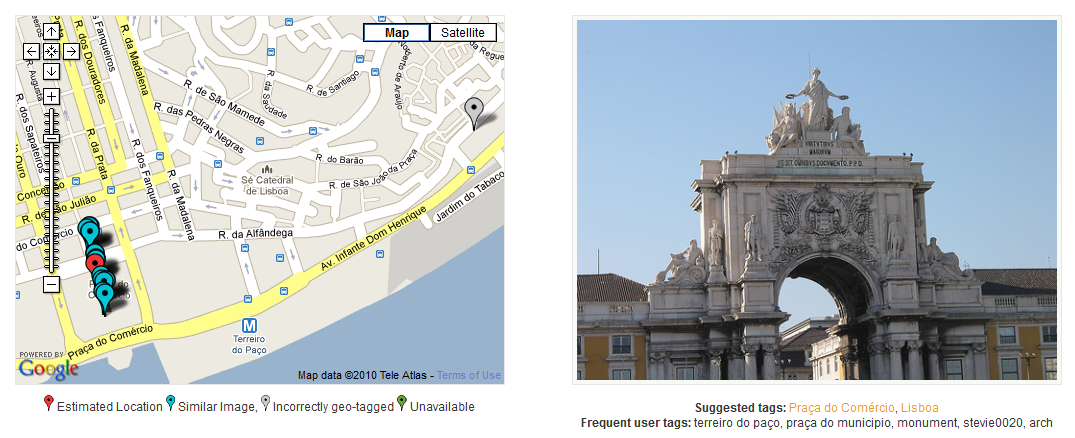
New applications are emerging every day exploiting the huge data volume in community photo collections. Most focus on popular subsets, e.g. images containing landmarks or associated to Wikipedia articles. In this work we are concerned with the problem of accurately finding the location where a photo is taken without needing any metadata, that is, solely by its visual content. We also recognize landmarks where applicable, automatically linking to Wikipedia. We show that the time is right for automating the geo-tagging process, and we show how this can work at large scale. In doing so, we do exploit redundancy of content in popular locations - but unlike most existing solutions, we do not restrict to landmarks. In other words, we can compactly represent the visual content of all thousands of images depicting e.g. the Parthenon and still retrieve any single, isolated, non-landmark image like a house or a graffiti on a wall. Starting from an existing, geo-tagged dataset, we cluster images into sets of different views of the same scene. This is a very efficient, scalable, and fully automated mining process. We then align all views in a set to one reference image and construct a 2D scene map. Our indexing scheme operates directly on scene maps. We evaluate our solution on a challenging one million urban image dataset and provide public access to our service through our application, VIRaL.
@article{J19,
title = {{VIRaL}: Visual Image Retrieval and Localization},
author = {Kalantidis, Yannis and Tolias, Giorgos and Avrithis, Yannis and Phinikettos, Marios and Spyrou, Evaggelos and Mylonas, Phivos and Kollias, Stefanos},
journal = {Multimedia Tools and Applications (MTAP)},
volume = {51},
number = {2},
month = {1},
pages = {555--592},
year = {2011}
}
2009
24(7):557-571 Aug 2009
Computer vision applications often need to process only a representative part of the visual input rather than the whole image/sequence. Considerable research has been carried out into salient region detection methods based either on models emulating human visual attention (VA) mechanisms or on computational approximations. Most of the proposed methods are bottom-up and their major goal is to filter out redundant visual information. In this paper, we propose and elaborate on a saliency detection model that treats a video sequence as a spatiotemporal volume and generates a local saliency measure for each visual unit (voxel). This computation involves an optimization process incorporating inter- and intra-feature competition at the voxel level. Perceptual decomposition of the input, spatiotemporal center-surround interactions and the integration of heterogeneous feature conspicuity values are described and an experimental framework for video classification is set up. This framework consists of a series of experiments that shows the effect of saliency in classification performance and let us draw conclusions on how well the detected salient regions represent the visual input. A comparison is attempted that shows the potential of the proposed method.
@article{J18,
title = {Spatiotemporal Saliency for Video Classification},
author = {Rapantzikos, Konstantinos and Tsapatsoulis, Nicolas and Avrithis, Yannis and Kollias, Stefanos},
journal = {Signal Processing: Image Communication (SP:IC)},
volume = {24},
number = {7},
month = {8},
pages = {557--571},
year = {2009}
}
11(11):229-243 Feb 2009
In this paper we investigate detection of high-level concepts in multimedia content through an integrated approach of visual thesaurus analysis and visual context. In the former, detection is based on model vectors that represent image composition in terms of region types, obtained through clustering over a large data set. The latter deals with two aspects, namely high-level concepts and region types of the thesaurus, employing a model of a priori specified semantic relations among concepts and automatically extracted topological relations among region types; thus it combines both conceptual and topological context. A set of algorithms is presented, which modify either the confidence values of detected concepts, or the model vectors based on which detection is performed. Visual context exploitation is evaluated on TRECVID and Corel data sets and compared to a number of related visual thesaurus approaches.
@article{J17,
title = {Using Visual Context and Region Semantics for High-Level Concept Detection},
author = {Mylonas, Phivos and Spyrou, Evaggelos and Avrithis, Yannis and Kollias, Stefanos},
journal = {IEEE Transactions on Multimedia (TMM)},
volume = {11},
number = {11},
month = {2},
pages = {229--243},
year = {2009}
}
41(3):337-373 Feb 2009
This paper presents a video analysis approach based on concept detection and keyframe extraction employing a visual thesaurus representation. Color and texture descriptors are extracted from coarse regions of each frame and a visual thesaurus is constructed after clustering regions. The clusters, called region types, are used as basis for representing local material information through the construction of a model vector for each frame, which reflects the composition of the image in terms of region types. Model vector representation is used for keyframe selection either in each video shot or across an entire sequence. The selection process ensures that all region types are represented. A number of high-level concept detectors is then trained using global annotation and Latent Semantic Analysis is applied. To enhance detection performance per shot, detection is employed on the selected keyframes of each shot, and a framework is proposed for working on very large data sets.
@article{J16,
title = {Concept detection and keyframe extraction using a visual thesaurus},
author = {Spyrou, Evaggelos and Tolias, Giorgos and Mylonas, Phivos and Avrithis, Yannis},
journal = {Multimedia Tools and Applications (MTAP)},
volume = {41},
number = {3},
month = {2},
pages = {337--373},
year = {2009}
}
2008
39(3):293-327 Sep 2008
In this paper we present a framework for unified, personalized access to heterogeneous multimedia content in distributed repositories. Focusing on semantic analysis of multimedia documents, metadata, user queries and user profiles, it contributes to the bridging of the gap between the semantic nature of user queries and raw multimedia documents. The proposed approach utilizes as input visual content analysis results, as well as analyzes and exploits associated textual annotation, in order to extract the underlying semantics, construct a semantic index and classify documents to topics, based on a unified knowledge and semantics representation model. It may then accept user queries, and, carrying out semantic interpretation and expansion, retrieve documents from the index and rank them according to user preferences, similarly to text retrieval. All processes are based on a novel semantic processing methodology, employing fuzzy algebra and principles of taxonomic knowledge representation. Part I of this work presented in this paper deals with data and knowledge models, manipulation of multimedia content annotations and semantic indexing, while Part II will continue on the use of the extracted semantic information for personalized retrieval.
@article{J15,
title = {Semantic Representation of Multimedia Content: Knowledge Representation and Semantic Indexing},
author = {Mylonas, Phivos and Athanasiadis, Thanos and Wallace, Manolis and Avrithis, Yannis and Kollias, Stefanos},
journal = {Multimedia Tools and Applications (MTAP)},
publisher = {Springer},
volume = {39},
number = {3},
month = {9},
pages = {293--327},
year = {2008}
}
23(1):73-100 Mar 2008
Context modeling has been long acknowledged as a key aspect in a wide variety of problem domains. In this paper we focus on the combination of contextualization and personalization methods to improve the performance of personalized information retrieval. The key aspects in our proposed approach are a) the explicit distinction between historic user context and live user context, b) the use of ontology-driven representations of the domain of discourse, as a common, enriched representational ground for content meaning, user interests, and contextual conditions, enabling the definition of effective means to relate the three of them, and c) the introduction of fuzzy representations as an instrument to properly handle the uncertainty and imprecision involved in the automatic interpretation of meanings, user attention, and user wishes. Based on a formal grounding at the representational level, we propose methods for the automatic extraction of persistent semantic user preferences, and live, ad-hoc user interests, which are combined in order to improve the accuracy and reliability of personalization for retrieval.
@article{J14,
title = {Personalized information retrieval based on context and ontological knowledge},
author = {Mylonas, Phivos and Vallet, David and Castells, Pablo and Fern\'andez, Miriam and Avrithis, Yannis},
journal = {Knowledge Engineering Review (KER)},
volume = {23},
number = {1},
month = {3},
pages = {73--100},
year = {2008}
}
2007
17(3):298-312 Mar 2007
In this paper we present a framework for simultaneous image segmentation and object labeling leading to automatic image annotation. Focusing on semantic analysis of images, it contributes to knowledge-assisted multimedia analysis and the bridging of the gap between its semantics and low level visual features. The proposed framework operates at semantic level using possible semantic labels, formally defined as fuzzy sets, to make decisions on handling image regions instead of visual features used traditionally. In order to stress its independence of a specific image segmentation approach we have modified two well known region growing algorithms, i.e. watershed and recursive shortest spanning tree, and compared them with their traditional counterparts. Additionally, a visual context representation and analysis approach is presented, blending global knowledge in interpreting each object locally. Contextual information is based on a novel semantic processing methodology, employing fuzzy algebra and ontological taxonomic knowledge representation. In this process, utilization of contextual knowledge re-adjusts semantic region growing labeling results appropriately, by means of fine-tuning the membership degrees of detected concepts. The performance of the overall methodology is demonstrated on a real-life still image dataset from two popular domains.
@article{J13,
title = {Semantic Image Segmentation and Object Labeling},
author = {Athanasiadis, Thanos and Mylonas, Phivos and Avrithis, Yannis and Kollias, Stefanos},
journal = {IEEE Transactions on Circuits and Systems for Video Technology (CSVT)},
volume = {17},
number = {3},
month = {3},
pages = {298--312},
year = {2007}
}
17(3):336-346 Mar 2007
Personalized content retrieval aims at improving the retrieval process by taking into account the particular interests of individual users. However, not all user preferences are relevant in all situations. It is well known that human preferences are complex, multiple, heterogeneous, changing, even contradictory, and should be understood in context with the user goals and tasks at hand. In this paper we propose a method to build a dynamic representation of the semantic context of ongoing retrieval tasks, which is used to activate different subsets of user interests at runtime, in a way that out–of-context preferences are discarded. Our approach is based on an ontology-driven representation of the domain of discourse, providing enriched descriptions of the semantics involved in retrieval actions and preferences, and enabling the definition of effective means to relate preferences and context.
@article{J12,
title = {Personalized Content Retrieval in Context Using Ontological Knowledge},
author = {Vallet, David and Castells, Pablo and Fern\'andez, Miriam and Mylonas, Phivos and Avrithis, Yannis},
journal = {IEEE Transactions on Circuits and Systems for Video Technology (CSVT)},
volume = {17},
number = {3},
month = {3},
pages = {336--346},
year = {2007}
}
1(2):237-248 Jun 2007
A video analysis framework based on spatiotemporal saliency calculation is presented. We propose a novel scheme for generating saliency in video sequences by taking into account both the spatial extent and dynamic evolution of regions. Towards this goal we extend a common image-oriented computational model of saliency-based visual attention to handle spatiotemporal analysis of video in a volumetric framework. The main claim is that attention acts as an efficient preprocessing step of a video sequence in order to obtain a compact representation of its content in the form of salient events/objects. The model has been implemented and qualitative as well as quantitative examples illustrating its performance are shown.
@article{J10,
title = {Bottom-Up Spatiotemporal Visual Attention Model for Video Analysis},
author = {Rapantzikos, Konstantinos and Tsapatsoulis, Nicolas and Avrithis, Yannis and Kollias, Stefanos},
journal = {IET Image Processing (IP)},
volume = {1},
number = {2},
month = {6},
pages = {237--248},
year = {2007}
}
2006
2(3):17-36 Jul 2006
In this article, an approach to semantic image analysis is presented. Under the proposed approach, ontologies are used to capture general, spatial, and contextual knowledge of a domain, and a genetic algorithm is applied to realize the final annotation. The employed domain knowledge considers high-level information in terms of the concepts of interest of the examined domain, contextual information in the form of fuzzy ontological relations, as well as low-level information in terms of prototypical low-level visual descriptors. To account for the inherent ambiguity in visual information, uncertainty has been introduced in the spatial relations definition. First, an initial hypothesis set of graded annotations is produced for each image region, and then context is exploited to update appropriately the estimated degrees of confidence. Finally, a genetic algorithm is applied to decide the most plausible annotation by utilizing the visual and the spatial concepts definitions included in the domain ontology. Experiments with a collection of photographs belonging to two different domains demonstrate the performance of the proposed approach.
@article{J11,
title = {Knowledge-Assisted Image Analysis Based on Context and Spatial Optimization},
author = {Papadopoulos, Georgios and Mylonas, Phivos and Mezaris, Vasileios and Avrithis, Yannis and Kompatsiaris, Ioannis},
journal = {International Journal on Semantic Web and Information Systems (SWIS)},
volume = {2},
number = {3},
month = {7},
pages = {17--36},
year = {2006}
}
Special issue on Knowledge-Based Digital Media Processing
153(3):255-262 Jun 2006
Knowledge representation and annotation of multimedia documents typically have been pursued in two different directions. Previous approaches have focused either on low level descriptors, such as dominant color, or on the semantic content dimension and corresponding manual annotations, such as person or vehicle. In this paper, we present a knowledge infrastructure and a experimentation platform for semantic annotation to bridge the two directions. Ontologies are being extended and enriched to include low-level audiovisual features and descriptors. Additionally, we present a tool that allows for linking low-level MPEG-7 visual descriptions to ontologies and annotations. This way we construct ontologies that include prototypical instances of high-level domain concepts together with a formal specification of the corresponding visual descriptors. This infrastructure is exploited by a knowledge-assisted analysis framework that may handle problems like segmentation, tracking, feature extraction and matching in order to classify scenes, identify and label objects, thus automatically create the associated semantic metadata.
@article{J9,
title = {Knowledge Representation and Semantic Annotation of Multimedia Content},
author = {Petridis, Kosmas and Bloehdorn, Stephan and Saathoff, Carsten and Simou, Nikolaos and Dasiopoulou, Stamatia and Tzouvaras, Vassilis and Handschuh, Siegfried and Avrithis, Yannis and Kompatsiaris, Ioannis and Staab, Steffen},
journal = {IEE Proceedings on Vision, Image and Signal Processing (VISP) (Special Issue on Knowledge-Based Digital Media Processing)},
volume = {153},
number = {3},
month = {6},
pages = {255--262},
year = {2006}
}
157(3):341-372 Feb 2006
The property of transitivity is one of the most important for fuzzy binary relations, especially in the cases when they are used for the representation of real life similarity or ordering information. As far as the algorithmic part of the actual calculation of the transitive closure of such relations is concerned, works in the literature mainly focus on crisp symmetric relations, paying little attention to the case of general fuzzy binary relations. Most works that deal with the algorithmic part of the transitive closure of fuzzy relations only focus on the case of max-min transitivity, disregarding other types of transitivity. In this paper, after formalizing the notion of sparseness and providing a representation model for sparse relations that displays both computational and storage merits, we propose an algorithm for the incremental update of fuzzy sup-t transitive relations. The incremental transitive update (ITU) algorithm achieves the re-establishment of transitivity when an already transitive relation is only locally disturbed. Based on this algorithm, we propose an extension to handle the sup-t transitive closure of any fuzzy binary relation, through a novel incremental transitive closure (ITC) algorithm. The ITU and ITC algorithms can be applied on any fuzzy binary relation and t-norm; properties such as reflexivity, symmetricity and idempotency are not a requirement. Under the specified assumptions for the average sparse relation, both of the proposed algorithms have considerably smaller computational complexity than the conventional approach; this is both established theoretically and verified via appropriate computing experiments.
@article{J8,
title = {Computationally efficient {sup-$t$} transitive closure for sparse fuzzy binary relations},
author = {Wallace, Manolis and Avrithis, Yannis and Kollias, Stefanos},
journal = {Fuzzy Sets and Systems (FSS)},
volume = {157},
number = {3},
month = {2},
pages = {341--372},
year = {2006}
}
36(1):34-52 Jan 2006
During the last few years numerous multimedia archives have made extensive use of digitized storage and annotation technologies. Still, the development of single points of access, providing common and uniform access to their data, despite the efforts and accomplishments of standardization organizations, has remained an open issue, as it involves the integration of various large scale heterogeneous and heterolingual systems. In this paper, we describe a mediator system that achieves architectural integration through an extended 3-tier architecture and content integration through semantic modeling. The described system has successfully integrated five multimedia archives, quite different in nature and content from each other, while also providing for easy and scalable inclusion of more archives in the future.
@article{J7,
title = {Integrating Multimedia Archives: The Architecture and the Content Layer},
author = {Wallace, Manolis and Athanasiadis, Thanos and Avrithis, Yannis and Delopoulos, Anastasios and Kollias, Stefanos},
journal = {IEEE Transactions on Systems, Man, and Cybernetics, Part A: Systems and Humans (SMC-A)},
volume = {36},
number = {1},
month = {1},
pages = {34--52},
year = {2006}
}
2003
9(6):510-519 Jun 2003
In this paper, an integrated information system is presented that offers enhanced search and retrieval capabilities to users of heterogeneous digital audiovisual (a/v) archives. This innovative system exploits the advances in handling a/v content and related metadata, as introduced by MPEG-4 and worked out by MPEG-7, to offer advanced services characterized by the tri-fold "semantic phrasing of the request (query)", "unified handling" and "personalized response". The proposed system is targeting the intelligent extraction of semantic information from a/v and text related data taking into account the nature of the queries that users my issue, and the context determined by user profiles. It also provides a personalization process of the response in order to provide end-users with desired information. From a technical point of view, the FAETHON system plays the role of an intermediate access server residing between the end users and multiple heterogeneous audiovisual archives organized according to the new MPEG standards.
@article{J6,
title = {Unified Access to Heterogeneous Audiovisual Archives},
author = {Avrithis, Yannis and Stamou, Giorgos and Wallace, Manolis and Marques, Ferran and Salembier, Philippe and Giro, Xavier and Haas, Werner and Vallant, Heribert and Zufferey, Michael},
journal = {Journal of Universal Computer Science (JUCS)},
volume = {9},
number = {6},
month = {6},
pages = {510--519},
year = {2003}
}
2001
Special issue on Image Indexation
4(2-3):93-107 Jun 2001
Pictures and video sequences showing human faces are of high importance in content-based retrieval systems, and consequently face detection has been established as an important tool in the framework of many multimedia applications like indexing, scene classification and news summarisation. In this work, we combine skin colour and shape features with template matching in an efficient way for the purpose of facial image indexing. We propose an adaptive two-dimensional Gaussian model of the skin colour distribution whose parameters are re-estimated based on the current image or frame, reducing generalisation problems. Masked areas obtained from skin colour detection are processed using morphological tools and assessed using global shape features. The verification stage is based on a template matching variation providing robust detection. Facial images and video sequences are indexed according to the number of included faces, their average colour components and their scale, leading to new types of content-based retrieval criteria in query-by-example frameworks. Experimental results have shown that the proposed implementation combines efficiency, robustness and speed, and could be easily embedded in generic visual information retrieval systems or video databases.
@article{J5,
title = {Facial Image Indexing in Multimedia Databases},
author = {Tsapatsoulis, Nicolas and Avrithis, Yannis and Kollias, Stefanos},
journal = {Pattern Analysis and Applications (PAA) (Special Issue on Image Indexation)},
volume = {4},
number = {2--3},
month = {6},
pages = {93--107},
year = {2001}
}
13(2):80-94 Nov 2001
A novel method for two-dimensional curve normalization with respect to affine transformations is presented in this paper, which allows an affine-invariant curve representation to be obtained without any actual loss of information on the original curve. It can be applied as a preprocessing step to any shape representation, classification, recognition, or retrieval technique, since it effectively decouples the problem of affine-invariant description from feature extraction and pattern matching. Curves estimated from object contours are first modeled by cubic B-splines and then normalized in several steps in order to eliminate translation, scaling, skew, starting point, rotation, and reflection transformations, based on a combination of curve features including moments and Fourier descriptors.
@article{J4,
title = {Affine-Invariant Curve Normalization for Object Shape Representation, Classification, and Retrieval},
author = {Avrithis, Yannis and Xirouhakis, Yiannis and Kollias, Stefanos},
journal = {Machine Vision and Applications (MVA)},
volume = {13},
number = {2},
month = {11},
pages = {80--94},
year = {2001}
}
2000
Special issue on Fuzzy Logic in Signal Processing
80(6):1049-1067 Jun 2000
In this paper, a fuzzy representation of visual content is proposed, which is useful for the new emerging multimedia applications, such as content-based image indexing and retrieval, video browsing and summarization. In particular, a multidimensional fuzzy histogram is constructed for each video frame based on a collection of appropriate features, extracted using video sequence analysis techniques. This approach is then applied both for video summarization, in the context of a content-based sampling algorithm, and for content-based indexing and retrieval. In the first case, video summarization is accomplished by discarding shots or frames of similar visual content so that only a small but meaningful amount of information is retained (key-frames). In the second case, a content-based retrieval scheme is investigated, so that the most similar images to a query are extracted. Experimental results and comparison with other known methods are presented to indicate the good performance of the proposed scheme on real-life video recordings.
@article{J3,
title = {A Fuzzy Video Content Representation for Video Summarization and Content-Based Retrieval},
author = {Doulamis, Anastasios and Doulamis, Nikolaos and Avrithis, Yannis and Kollias, Stefanos},
journal = {Signal Processing (SP) (Special Issue on Fuzzy Logic in Signal Processing)},
volume = {80},
number = {6},
month = {6},
pages = {1049--1067},
year = {2000}
}
Special issue on {3D} Video Technology
10(4):501-517 Jun 2000
An efficient technique for summarization of stereoscopic video sequences is presented in this paper, which extracts a small but meaningful set of video frames using a content-based sampling algorithm. The proposed video-content representation provides the capability of browsing digital stereoscopic video sequences and performing more efficient content-based queries and indexing. Each stereoscopic video sequence is first partitioned into shots by applying a shot-cut detection algorithm so that frames (or stereo pairs) of similar visual characteristics are gathered together. Each shot is then analyzed using stereo-imaging techniques, and the disparity field, occluded areas, and depth map are estimated. A multiresolution implementation of the Recursive Shortest Spanning Tree (RSST) algorithm is applied for color and depth segmentation, while fusion of color and depth segments is employed for reliable video object extraction. In particular, color segments are projected onto depth segments so that video objects on the same depth plane are retained, while at the same time accurate object boundaries are extracted. Feature vectors are then constructed using multidimensional fuzzy classification of segment features including size, location, color, and depth. Shot selection is accomplished by clustering similar shots based on the generalized Lloyd-Max algorithm, while for a given shot, key frames are extracted using an optimization method for locating frames of minimally correlated feature vectors. For efficient implementation of the latter method, a genetic algorithm is used. Experimental results are presented, which indicate the reliable performance of the proposed scheme on real-life stereoscopic video sequences.
@article{J2,
title = {Efficient Summarization of Stereoscopic Video Sequences},
author = {Doulamis, Nikolaos and Doulamis, Anastasios and Avrithis, Yannis and Ntalianis, Klimis and Kollias, Stefanos},
journal = {IEEE Transactions on Circuits and Systems for Video Technology (CSVT) (Special Issue on {3D} Video Technology)},
volume = {10},
number = {4},
month = {6},
pages = {501--517},
year = {2000}
}
1999
Special issue on Content-Based Access of Image and Video Libraries
75(1-2):3-24 Jul 1999
A video content representation framework is proposed in this paper for extracting limited, but meaningful, information of video data, directly from the MPEG compressed domain. A hierarchical color and motion segmentation scheme is applied to each video shot, transforming the frame-based representation to a feature-based one. The scheme is based on a multiresolution implementation of the recursive shortest spanning tree (RSST) algorithm. Then, all segment features are gathered together using a fuzzy multidimensional histogram to reduce the possibility of classifying similar segments to different classes. Extraction of several key frames is performed for each shot in a content-based rate-sampling framework. Two approaches are examined for key frame extraction. The first is based on examination of the temporal variation of the feature vector trajectory; the second is based on minimization of a cross-correlation criterion of the video frames. For efficient implementation of the latter approach, a logarithmic search (along with a stochastic version) and a genetic algorithm are proposed. Experimental results are presented which illustrate the performance of the proposed techniques, using synthetic and real life MPEG video sequences.
@article{J1,
title = {A Stochastic Framework for Optimal Key Frame Extraction from {MPEG} Video Databases},
author = {Avrithis, Yannis and Doulamis, Anastasios and Doulamis, Nikolaos and Kollias, Stefanos},
journal = {Computer Vision and Image Understanding (CVIU) (Special Issue on Content-Based Access of Image and Video Libraries)},
volume = {75},
number = {1--2},
month = {7},
pages = {3--24},
year = {1999}
}
Conference proceedings
2025
Tucson, AZ, US Mar 2025
This work addresses composed image retrieval in the context of domain conversion, where the content of the query image is to be retrieved in a domain given by the query text. We show that a strong generic vision-language model already provides sufficient descriptive power and no further learning is necessary. The query image is mapped to the input text space by textual inversion. In contrast to the common practice of inverting to the continuous space of textual tokens, we opt for inversion into the discrete space of words using a text vocabulary. This distinction is empirically validated and proven to be a pivotal factor. Through inversion, we represent the image by soft assignment to the vocabulary. Such a text description is made more robust by engaging a set of images visually similar to the query image. Images are retrieved via a weighted ensemble of text queries, each composed of one of the words assigned to the query image and the domain query text. Our method outperforms prior art by a large margin on standard as well as on newly introduced benchmarks.
@conference{C138,
title = {Composed Image Retrieval for Training-Free Domain Conversion},
author = {Efthymiadis, Nikos and Psomas, Bill and Laskar, Zakaria and Karantzalos, Konstantinos and Avrithis, Yannis and Chum, Ondrej and Tolias, Giorgos},
booktitle = {Proceedings of IEEE/CVF Winter Conference on Applications of Computer Vision (WACV)},
month = {3},
address = {Tucson, AZ, US},
year = {2025}
}
2024
part of IEEE Conference on Computer Vision and Pattern Recognition
Seattle, WA, US Jun 2024
Explanations obtained from transformer-based architectures, in the form of raw attention, can be seen as a class agnostic saliency map. Additionally, attention-based pooling serves as a form of masking in feature space. Motivated by this observation, we design an attention-based pooling mechanism intended to replace global average pooling during inference. This mechanism, called Cross Attention Stream (CA-Stream), comprises a stream of cross attention blocks interacting with features at different network levels. CA-Stream enhances interpretability properties in existing image recognition models, while preserving their recognition properties.
@conference{C137,
title = {{CA}-Stream: Attention-based pooling for interpretable image recognition},
author = {Torres Figueroa, Felipe and Zhang, Hanwei and Sicre, Ronan and Ayache, Stephane and Avrithis, Yannis},
booktitle = {Proceedings of 3rd Workshop on Explainable AI for Computer Vision (XAI4CV), part of IEEE Conference on Computer Vision and Pattern Recognition (CVPR)},
month = {6},
address = {Seattle, WA, US},
year = {2024}
}
Athens, Greece Jul 2024
The surge in data volume within the field of remote sensing has necessitated efficient methods for retrieving relevant information from extensive image archives. Conventional unimodal queries, whether visual or textual, are insufficient and restrictive. To address this limitation, we introduce the task of composed image retrieval in remote sensing, allowing users to combine query images with a textual part that modifies attributes such as color, texture, context, or more, thereby enhancing the expressivity of the query.
We demonstrate that a vision-language model possesses sufficient descriptive power and, when coupled with the proposed fusion method, eliminates the necessity for further learning. We present a new evaluation benchmark focused on shape, color, density, and quantity modifications. Our work not only sets the state-of-the-art for this task, but also serves as a foundational step in addressing a gap in the field of remote sensing image retrieval.
@conference{C136,
title = {Composed Image Retrieval for Remote Sensing},
author = {Psomas, Bill and Kakogeorgiou, Ioannis and Efthymiadis, Nikos and Tolias, Giorgos and Chum, Ondrej and Avrithis, Yannis and Karantzalos, Konstantinos},
booktitle = {Proceedings of IEEE International Geoscience and Remote Sensing Symposium (IGARSS) (Oral)},
month = {7},
address = {Athens, Greece},
year = {2024}
}
Seattle, WA, US Jun 2024
How important is it for training and evaluation sets to not have class overlap in image retrieval? We revisit Google Landmarks v2 clean, the most popular training set, by identifying and removing class overlap with Revisited Oxford and Paris, the most popular evaluation set. By comparing the original and the new $\mathcal{R}$GLDv2-clean on a benchmark of reproduced state-of-the-art methods, our findings are striking. Not only is there a dramatic drop in performance, but it is inconsistent across methods, changing the ranking.
What does it take to focus on objects or interest and ignore background clutter when indexing? Do we need to train an object detector and the representation separately? Do we need location supervision? We introduce Single-stage Detect-to-Retrieve (CiDeR), an end-to-end, single-stage pipeline to detect objects of interest and extract a global image representation. We outperform previous state-of-the-art on both existing training sets and the new $\mathcal{R}$GLDv2-clean. Our dataset is available at https://github.com/dealicious-inc/RGLDv2-clean.
@conference{C135,
title = {On Train-Test Class Overlap and Detection for Image Retrieval},
author = {Song, Chull Hwan and Yoon, Jooyoung and Hwang, Taebaek and Choi, Shunghyun and Gu, Yeong Hyeon and Avrithis, Yannis},
booktitle = {Proceedings of IEEE Conference on Computer Vision and Pattern Recognition (CVPR)},
month = {6},
address = {Seattle, WA, US},
year = {2024}
}
Vienna, Austria May 2024
Self-supervised learning has unlocked the potential of scaling up pretraining to billions of images, since annotation is unnecessary. But are we making the best use of data? How more economical can we be? In this work, we attempt to answer this question by making two contributions. First, we investigate first-person videos and introduce a "Walking Tours" dataset. These videos are high-resolution, hours-long, captured in a single uninterrupted take, depicting a large number of objects and actions with natural scene transitions. They are unlabeled and uncurated, thus realistic for self-supervision and comparable with human learning.
Second, we introduce a novel self-supervised image pretraining method tailored for learning from continuous videos. Existing methods typically adapt image-based pretraining approaches to incorporate more frames. Instead, we advocate a "tracking to learn to recognize" approach. Our method called DoRA, leads to attention maps that DiscOver and tRAck objects over time in an end-to-end manner, using transformer cross-attention. We derive multiple views from the tracks and use them in a classical self-supervised distillation loss. Using our novel approach, a single Walking Tours video remarkably becomes a strong competitor to ImageNet for several image and video downstream tasks.
@conference{C134,
title = {Is ImageNet worth 1 video? Learning strong image encoders from 1 long unlabelled video},
author = {Venkataramanan, Shashanka and Rizve, Mamshad Nayeem and Carreira, Jo\~ao and Asano, Yuki M. and Avrithis, Yannis},
booktitle = {Proceedings of International Conference on Learning Representations (ICLR) (Oral). Outstanding Paper Honorable Mention},
month = {5},
address = {Vienna, Austria},
year = {2024}
}
Rome, Italy Feb 2024
This paper studies interpretability of convolutional networks by means of saliency maps. Most approaches based on Class Activation Maps (CAM) combine information from fully connected layers and gradient through variants of backpropagation. However, it is well understood that gradients are noisy and alternatives like guided backpropagation have been proposed to obtain better visualization at inference. In this work, we present a novel training approach to improve the quality of gradients for interpretability. In particular, we introduce a regularization loss such that the gradient with respect to the input image obtained by standard backpropagation is similar to the gradient obtained by guided backpropagation. We find that the resulting gradient is qualitatively less noisy and improves quantitatively the interpretability properties of different networks, using several interpretability methods.
@conference{C133,
title = {A Learning Paradigm for Interpretable Gradients},
author = {Torres Figueroa, Felipe and Zhang, Hanwei and Sicre, Ronan and Avrithis, Yannis and Ayache, Stephane},
booktitle = {Proceedings of International Conference on Computer Vision Theory and Applications (VISAPP) (Oral)},
month = {2},
address = {Rome, Italy},
year = {2024}
}
Waikoloa, HI, US Jan 2024
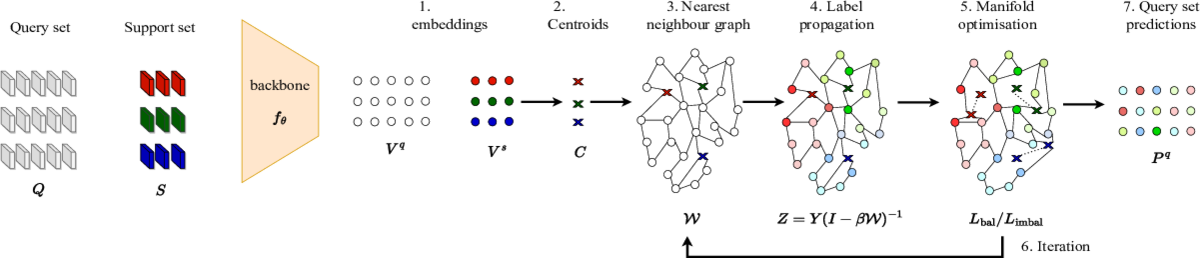
Transductive few-shot learning algorithms have showed substantially superior performance over their inductive counterparts by leveraging the unlabeled queries at inference. However, the vast majority of transductive methods are evaluated on perfectly class-balanced benchmarks. It has been shown that they undergo remarkable drop in performance under a more realistic, imbalanced setting.
To this end, we propose a novel algorithm to address imbalanced transductive few-shot learning, named Adaptive Manifold. Our algorithm exploits the underlying manifold of the labeled examples and unlabeled queries by using manifold similarity to predict the class probability distribution of every query. It is parameterized by one centroid per class and a set of manifold parameters that determine the manifold. All parameters are optimized by minimizing a loss function that can be tuned towards class-balanced or imbalanced distributions. The manifold similarity shows substantial improvement over Euclidean distance, especially in the 1-shot setting.
Our algorithm outperforms all other state of the art methods in three benchmark datasets, namely miniImageNet, tieredImageNet and CUB, and two different backbones, namely ResNet-18, WideResNet-28-10. In certain cases, our algorithm outperforms the previous state of the art by as much as 4.2%.
@conference{C132,
title = {Adaptive manifold for imbalanced transductive few-shot learning},
author = {Lazarou, Michalis and Avrithis, Yannis and Stathaki, Tania},
booktitle = {Proceedings of IEEE Winter Conference on Applications of Computer Vision (WACV)},
month = {1},
address = {Waikoloa, HI, US},
year = {2024}
}
2023
New Orleans, LA, US Dec 2023
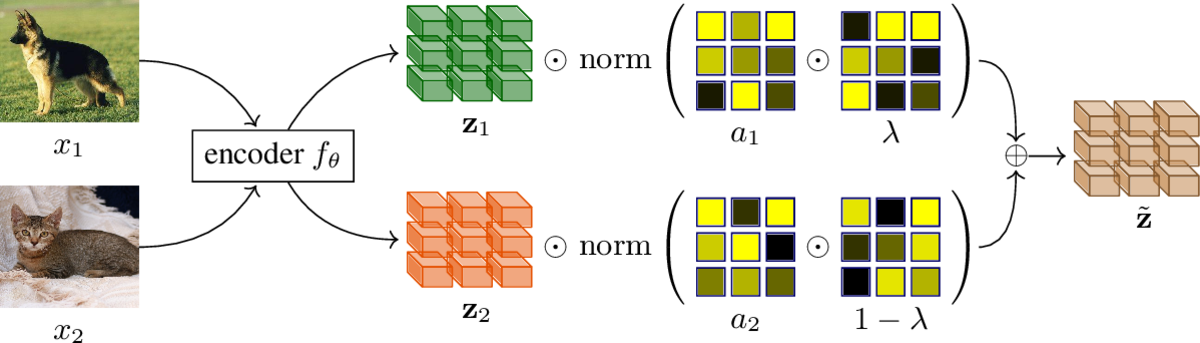
Mixup refers to interpolation-based data augmentation, originally motivated as a way to go beyond empirical risk minimization (ERM). Its extensions mostly focus on the definition of interpolation and the space (input or embedding) where it takes place, while the augmentation process itself is less studied. In most methods, the number of generated examples is limited to the mini-batch size and the number of examples being interpolated is limited to two (pairs), in the input space.
We make progress in this direction by introducing MultiMix, which generates an arbitrarily large number of interpolated examples beyond the mini-batch size, and interpolates the entire mini-batch in the embedding space. Effectively, we sample on the entire convex hull of the mini-batch rather than along linear segments between pairs of examples.
On sequence data we further extend to Dense MultiMix. We densely interpolate features and target labels at each spatial location and also apply the loss densely. To mitigate the lack of dense labels, we inherit labels from examples and weight interpolation factors by attention as a measure of confidence.
Overall, we increase the number of loss terms per mini-batch by orders of magnitude at little additional cost. This is only possible because of interpolating in the embedding space. We empirically show that our solutions yield significant improvement over state-of-the-art mixup methods on four different benchmarks, despite interpolation being only linear. By analyzing the embedding space, we show that the classes are more tightly clustered and uniformly spread over the embedding space, thereby explaining the improved behavior.
@conference{C131,
title = {Embedding Space Interpolation Beyond Mini-Batch, Beyond Pairs and Beyond Examples},
author = {Venkataramanan, Shashanka and Kijak, Ewa and Amsaleg, Laurent and Avrithis, Yannis},
booktitle = {Proceedings of Conference on Neural Information Processing Systems (NeurIPS)},
month = {12},
address = {New Orleans, LA, US},
year = {2023}
}
part of International Conference on Computer Vision
Paris, France Oct 2023
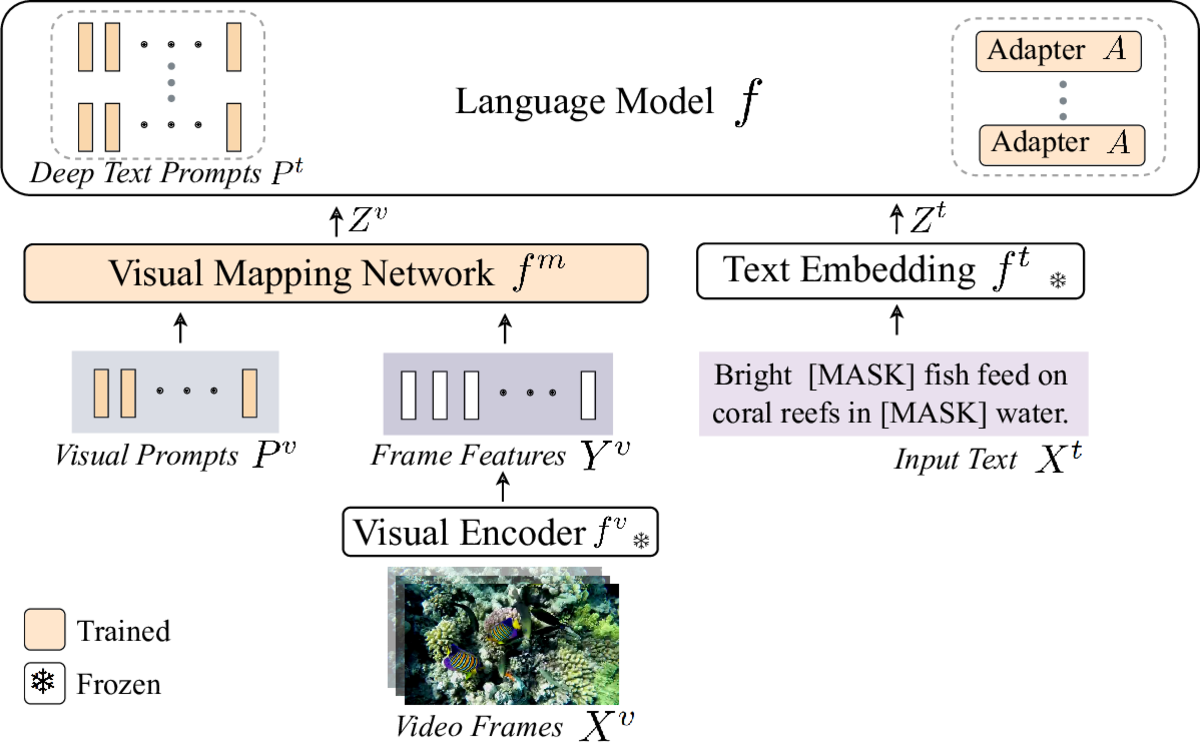
Recent vision-language models are driven by large-scale pretrained models. However, adapting pretrained models on limited data presents challenges such as overfitting, catastrophic forgetting, and the cross-modal gap between vision and language. We introduce a parameter-efficient method to address these challenges, combining multimodal prompt learning and a transformer-based mapping network, while keeping the pretrained models frozen. Our experiments on several video question answering benchmarks demonstrate the superiority of our approach in terms of performance and parameter efficiency on both zero-shot and few-shot settings. Our code is available at https://engindeniz.github.io/vitis.
@conference{C130,
title = {Zero-Shot and Few-Shot Video Question Answering with Multi-Modal Prompts},
author = {Engin, Deniz and Avrithis, Yannis},
booktitle = {Proceedings of 5th Workshop on Closing the Loop Between Vision and Language (CLVL), part of International Conference on Computer Vision (ICCV)},
month = {10},
address = {Paris, France},
year = {2023}
}
Paris, France Oct 2023
Convolutional networks and vision transformers have different forms of pairwise interactions, pooling across layers and pooling at the end of the network. Does the latter really need to be different? As a by-product of pooling, vision transformers provide spatial attention for free, but this is most often of low quality unless self-supervised, which is not well studied. Is supervision really the problem? In this work, we develop a generic pooling framework and then we formulate a number of existing methods as instantiations. By discussing the properties of each group of methods, we derive SimPool, a simple attention-based pooling mechanism as a replacement of the default one for both convolutional and transformer encoders. We find that, whether supervised or self-supervised, this improves performance on pre-training and downstream tasks and provides attention maps delineating object boundaries in all cases. One could thus call SimPool universal. To our knowledge, we are the first to obtain attention maps in supervised transformers of at least as good quality as self-supervised, without explicit losses or modifying the architecture. Code at: https://github.com/billpsomas/simpool.
@conference{C129,
title = {Keep It {SimPool}: Who Said Supervised Transformers Suffer from Attention Deficit?},
author = {Psomas, Bill and Kakogeorgiou, Ioannis and Karantzalos, Konstantinos and Avrithis, Yannis},
booktitle = {Proceedings of International Conference on Computer Vision (ICCV)},
month = {10},
address = {Paris, France},
year = {2023}
}
Kuala Lumpur, Malaysia Oct 2023
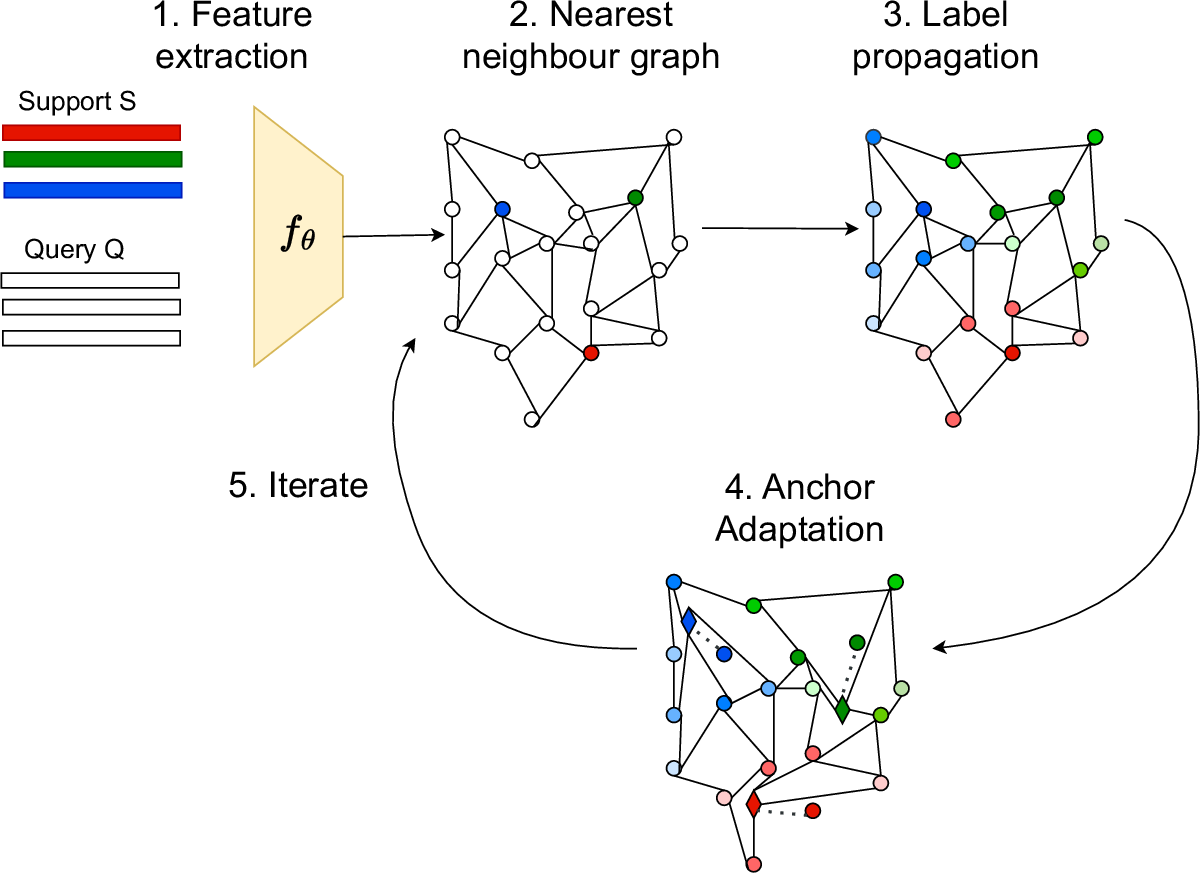
Few-shot learning addresses the issue of classifying images using limited labeled data. Exploiting unlabeled data through the use of transductive inference methods such as label propagation has been shown to improve the performance of few-shot learning significantly. Label propagation infers pseudo-labels for unlabeled data by utilizing a constructed graph that exploits the underlying manifold structure of the data. However, a limitation of the existing label propagation approaches is that the positions of all data points are fixed and might be sub-optimal so that the algorithm is not as effective as possible. In this work, we propose a novel algorithm that adapts the feature embeddings of the labeled data by minimizing a differentiable loss function optimizing their positions in the manifold in the process. Our novel algorithm, Adaptive Anchor Label Propagation, outperforms the standard label propagation algorithm by as much as 7% and 2% in the 1-shot and 5-shot settings respectively. We provide experimental results highlighting the merits of our algorithm on four widely used few-shot benchmark datasets, namely miniImageNet, tieredImageNet, CUB and CIFAR-FS and two commonly used backbones, ResNet12 and WideResNet-28-10. The source code can be found at https://github.com/MichalisLazarou/A2LP.
@conference{C128,
title = {Adaptive Anchors Label Propagation for Transductive Few-Shot Learning},
author = {Lazarou, Michalis and Avrithis, Yannis and Ren, Guangyu and Stathaki, Tania},
booktitle = {Proceedings of International Conference on Image Processing (ICIP)},
month = {10},
address = {Kuala Lumpur, Malaysia},
year = {2023}
}
Vancouver, Canada Jun 2023

Impressive progress in generative models and implicit representations gave rise to methods that can generate 3D shapes of high quality. However, being able to locally control and edit shapes is another essential property that can unlock several content creation applications. Local control can be achieved with part-aware models, but existing methods require 3D supervision and cannot produce textures. In this work, we devise PartNeRF, a novel part-aware generative model for editable 3D shape synthesis that does not require any explicit 3D supervision. Our model generates objects as a set of locally defined NeRFs, augmented with an affine transformation. This enables several editing operations such as applying transformations on parts, mixing parts from different objects etc. To ensure distinct, manipulable parts we enforce a hard assignment of rays to parts that makes sure that the color of each ray is only determined by a single NeRF. As a result, altering one part does not affect the appearance of the others. Evaluations on various ShapeNet categories demonstrate the ability of our model to generate editable 3D objects of improved fidelity, compared to previous part-based generative approaches that require 3D supervision or models relying on NeRFs.
@conference{C127,
title = {Generating Part-Aware Editable 3D Shapes Without 3D Supervision},
author = {Tertikas, Konstantinos and Paschalidou, Despoina and Pan, Boxiao and Park, Jeong Joon and Uy, Mikaela Angelina and Emiris, Ioannis and Avrithis, Yannis and Guibas, Leonidas},
booktitle = {Proceedings of IEEE Conference on Computer Vision and Pattern Recognition (CVPR)},
month = {6},
address = {Vancouver, Canada},
year = {2023}
}
Waikoloa, HI, US Jan 2023
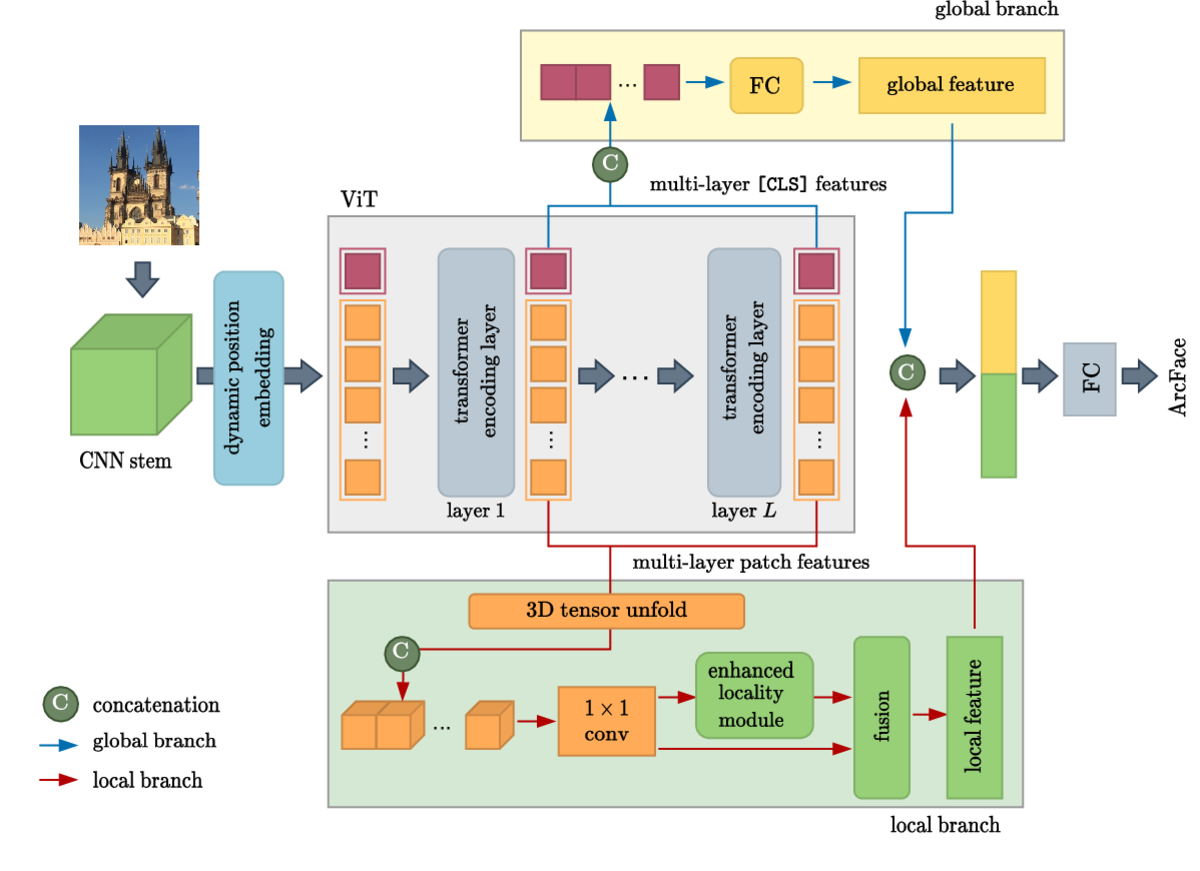
Vision transformers have achieved remarkable progress in vision tasks such as image classification and detection. However, in instance-level image retrieval, transformers have not yet shown good performance compared to convolutional networks. We propose a number of improvements that make transformers outperform the state of the art for the first time. (1) We show that a hybrid architecture is more effective than plain transformers, by a large margin. (2) We introduce two branches collecting global (classification token) and local (patch tokens) information, from which we form a global image representation. (3) In each branch, we collect multi-layer features from the transformer encoder, corresponding to skip connections across distant layers. (4) We enhance locality of interactions at the deeper layers of the encoder, which is the relative weakness of vision transformers. We train our model on all commonly used training sets and, for the first time, we make fair comparisons separately per training set. In all cases, we outperform previous models based on global representation. Public code is available at https://github.com/dealicious-inc/DToP.
@conference{C126,
title = {Boosting vision transformers for image retrieval},
author = {Song, Chull Hwan and Yoon, Jooyoung and Choi, Shunghyun and Avrithis, Yannis},
booktitle = {Proceedings of IEEE Winter Conference on Applications of Computer Vision (WACV)},
month = {1},
address = {Waikoloa, HI, US},
year = {2023}
}
2022
Tel Aviv, Isreal Oct 2022
Transformers and masked language modeling are quickly being adopted and explored in computer vision as vision transformers and masked image modeling (MIM). In this work, we argue that image token masking differs from token masking in text, due to the amount and correlation of tokens in an image. In particular, to generate a challenging pretext task for MIM, we advocate a shift from random masking to informed masking. We develop and exhibit this idea in the context of distillation-based MIM, where a teacher transformer encoder generates an attention map, which we use to guide masking for the student.
We thus introduce a novel masking strategy, called attention-guided masking (AttMask), and we demonstrate its effectiveness over random masking for dense distillation-based MIM as well as plain distillation-based self-supervised learning on classification tokens. We confirm that AttMask accelerates the learning process and improves the performance on a variety of downstream tasks. We provide the implementation code at https://github.com/gkakogeorgiou/attmask.
@conference{C125,
title = {What to Hide from Your Students: Attention-Guided Masked Image Modeling},
author = {Kakogeorgiou, Ioannis and Gidaris, Spyros and Psomas, Bill and Avrithis, Yannis and Bursuc, Andrei and Karantzalos, Konstantinos and Komodakis, Nikos},
booktitle = {Proceedings of European Conference on Computer Vision (ECCV)},
month = {10},
address = {Tel Aviv, Isreal},
year = {2022}
}
New Orleans, LA, US Jun 2022
Mixup is a powerful data augmentation method that interpolates between two or more examples in the input or feature space and between the corresponding target labels. Many recent mixup methods focus on cutting and pasting two or more objects into one image, which is more about efficient processing than interpolation. However, how to best interpolate images is not well defined. In this sense, mixup has been connected to autoencoders, because often autoencoders "interpolate well", for instance generating an image that continuously deforms into another.
In this work, we revisit mixup from the interpolation perspective and introduce AlignMix, where we geometrically align two images in the feature space. The correspondences allow us to interpolate between two sets of features, while keeping the locations of one set. Interestingly, this gives rise to a situation where mixup retains mostly the geometry or pose of one image and the texture of the other, connecting it to style transfer. More than that, we show that an autoencoder can still improve representation learning under mixup, without the classifier ever seeing decoded images. AlignMix outperforms state-of-the-art mixup methods on five different benchmarks.
@conference{C124,
title = {{AlignMixup}: Improving representations by interpolating aligned features},
author = {Venkataramanan, Shashanka and Kijak, Ewa and Amsaleg, Laurent and Avrithis, Yannis},
booktitle = {Proceedings of IEEE Conference on Computer Vision and Pattern Recognition (CVPR)},
month = {6},
address = {New Orleans, LA, US},
year = {2022}
}
Virtual Apr 2022
Metric learning involves learning a discriminative representation such that embeddings of similar classes are encouraged to be close, while embeddings of dissimilar classes are pushed far apart. State-of-the-art methods focus mostly on sophisticated loss functions or mining strategies. On the one hand, metric learning losses consider two or more examples at a time. On the other hand, modern data augmentation methods for classification consider two or more examples at a time. The combination of the two ideas is under-studied.
In this work, we aim to bridge this gap and improve representations using mixup, which is a powerful data augmentation approach interpolating two or more examples and corresponding target labels at a time. This task is challenging because, unlike classification, the loss functions used in metric learning are not additive over examples, so the idea of interpolating target labels is not straightforward. To the best of our knowledge, we are the first to investigate mixing both examples and target labels for deep metric learning. We develop a generalized formulation that encompasses existing metric learning loss functions and modify it to accommodate for mixup, introducing Metric Mix, or Metrix. We also introduce a new metric---utilization---to demonstrate that by mixing examples during training, we are exploring areas of the embedding space beyond the training classes, thereby improving representations. To validate the effect of improved representations, we show that mixing inputs, intermediate representations or embeddings along with target labels significantly outperforms state-of-the-art metric learning methods on four benchmark deep metric learning datasets.
@conference{C123,
title = {It Takes Two to Tango: Mixup for Deep Metric Learning},
author = {Venkataramanan, Shashanka and Psomas, Bill and Kijak, Ewa and Amsaleg, Laurent and Karantzalos, Konstantinos and Avrithis, Yannis},
booktitle = {Proceedings of International Conference on Learning Representations (ICLR)},
month = {4},
address = {Virtual},
year = {2022}
}
Waikoloa, HI, US Jan 2022
We address representation learning for large-scale instance-level image retrieval. Apart from backbone, training pipelines and loss functions, popular approaches have focused on different spatial pooling and attention mechanisms, which are at the core of learning a powerful global image representation. There are different forms of attention according to the interaction of elements of the feature tensor (local and global) and the dimensions where it is applied (spatial and channel). Unfortunately, each study addresses only one or two forms of attention and applies it to different problems like classification, detection or retrieval.
We present global-local attention module (GLAM), which is attached at the end of a backbone network and incorporates all four forms of attention: local and global, spatial and channel. We obtain a new feature tensor and, by spatial pooling, we learn a powerful embedding for image retrieval. Focusing on global descriptors, we provide empirical evidence of the interaction of all forms of attention and improve the state of the art on standard benchmarks.
@conference{C122,
title = {All the attention you need: Global-local, spatial-channel attention for image retrieval},
author = {Song, Chull Hwan and Han, Hye Joo and Avrithis, Yannis},
booktitle = {Proceedings of IEEE Winter Conference on Applications of Computer Vision (WACV)},
month = {1},
address = {Waikoloa, HI, US},
year = {2022}
}
Waikoloa, HI, US Jan 2022
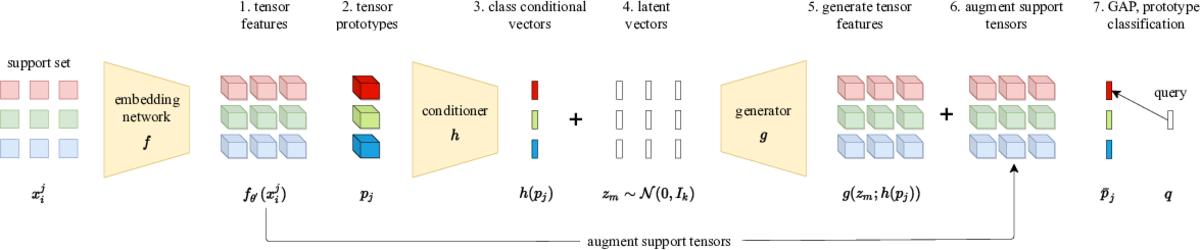
Few-shot learning addresses the challenge of learning how to address novel tasks given not just limited supervision but limited data as well. An attractive solution is synthetic data generation. However, most such methods are overly sophisticated, focusing on high-quality, realistic data in the input space. It is unclear whether adapting them to the few-shot regime and using them for the downstream task of classification is the right approach. Previous works on synthetic data generation for few-shot classification focus on exploiting complex models, e.g. a Wasserstein GAN with multiple regularizers or a network that transfers latent diversities from known to novel classes.
We follow a different approach and investigate how a simple and straightforward synthetic data generation method can be used effectively. We make two contributions, namely we show that: (1) using a simple loss function is more than enough for training a feature generator in the few-shot setting; and (2) learning to generate tensor features instead of vector features is superior. Extensive experiments on miniImagenet, CUB and CIFAR-FS datasets show that our method sets a new state of the art, outperforming more sophisticated few-shot data augmentation methods. The source code can be found at https://github.com/MichalisLazarou/TFH_fewshot.
@conference{C121,
title = {Tensor feature hallucination for few-shot learning},
author = {Lazarou, Michalis and Stathaki, Tania and Avrithis, Yannis},
booktitle = {Proceedings of IEEE Winter Conference on Applications of Computer Vision (WACV)},
month = {1},
address = {Waikoloa, HI, US},
year = {2022}
}
2021
part of ACM Multimedia Conference
Chengdu, China Oct 2021
Deep Neural Networks (DNNs) are robust against intra-class variability of images, pose variations and random noise, but vulnerable to imperceptible adversarial perturbations that are well-crafted precisely to mislead. While random noise even of relatively large magnitude can hardly affect predictions, adversarial perturbations of very small magnitude can make a classifier fail completely.
To enhance robustness, we introduce a new adversarial defense called patch replacement, which transforms both the input images and their intermediate features at early layers to make adversarial perturbations behave similarly to random noise. We decompose images/features into small patches and quantize them according to a codebook learned from legitimate training images. This maintains the semantic information of legitimate images, while removing as much as possible the effect of adversarial perturbations.
Experiments show that patch replacement improves robustness against both white-box and gray-box attacks, compared with other transformation-based defenses. It has a low computational cost since it does not need training or fine-tuning the network. Importantly, in the white-box scenario, it increases the robustness, while other transformation-based defenses do not.
@conference{C120,
title = {Patch replacement: A transformation-based method to improve robustness against adversarial attacks},
author = {Zhang, Hanwei and Avrithis, Yannis and Furon, Teddy and Amsaleg, Laurent},
booktitle = {Proceedings of International Workshop on Trustworthy AI for Multimedia Computing (TAI), part of ACM Multimedia Conference (ACM-MM)},
month = {10},
address = {Chengdu, China},
year = {2021}
}
Virtual Oct 2021
High-level understanding of stories in video such as movies and TV shows from raw data is extremely challenging. Modern video question answering (VideoQA) systems often use additional human-made sources like plot synopses, scripts, video descriptions or knowledge bases. In this work, we present a new approach to understand the whole story without such external sources. The secret lies in the dialog: unlike any prior work, we treat dialog as a noisy source to be converted into text description via dialog summarization, much like recent methods treat video. The input of each modality is encoded by transformers independently, and a simple fusion method combines all modalities, using soft temporal attention for localization over long inputs. Our model outperforms the state of the art on the KnowIT VQA dataset by a large margin, without using question-specific human annotation or human-made plot summaries. It even outperforms human evaluators who have never watched any whole episode before.
@conference{C119,
title = {On the hidden treasure of dialog in video question answering},
author = {Engin, Deniz and Duong, Ngoc Q. K. and Schnitzler, Fran\c{c}ois and Avrithis, Yannis},
booktitle = {Proceedings of International Conference on Computer Vision (ICCV)},
month = {10},
address = {Virtual},
year = {2021}
}
Virtual Oct 2021
Few-shot learning amounts to learning representations and acquiring knowledge such that novel tasks may be solved with both supervision and data being limited. Improved performance is possible by transductive inference, where the entire test set is available concurrently, and semi-supervised learning, where more unlabeled data is available. These problems are closely related because there is little or no adaptation of the representation in novel tasks.
Focusing on these two settings, we introduce a new algorithm that leverages the manifold structure of the labeled and unlabeled data distribution to predict pseudo-labels, while balancing over classes and using the loss value distribution of a limited-capacity classifier to select the cleanest labels, iterately improving the quality of pseudo-labels. Our solution sets new state of the art results on four benchmark datasets, namely miniImageNet, tieredImageNet, CUB and CIFAR-FS, while being robust over feature space pre-processing and the quantity of available data.
@conference{C118,
title = {Iterative label cleaning for transductive and semi-supervised few-shot learning},
author = {Lazarou, Michalis and Stathaki, Tania and Avrithis, Yannis},
booktitle = {Proceedings of International Conference on Computer Vision (ICCV)},
month = {10},
address = {Virtual},
year = {2021}
}
Virtual Jun 2021
Knowledge transfer from large teacher models to smaller student models has recently been studied for metric learning, focusing on fine-grained classification. In this work, focusing on instance-level image retrieval, we study an asymmetric testing task, where the database is represented by the teacher and queries by the student. Inspired by this task, we introduce asymmetric metric learning, a novel paradigm of using asymmetric representations at training. This acts as a simple combination of knowledge transfer with the original metric learning task.
We systematically evaluate different teacher and student models, metric learning and knowledge transfer loss functions on the new asymmetric testing as well as the standard symmetric testing task, where database and queries are represented by the same model. We find that plain regression is surprisingly effective compared to more complex knowledge transfer mechanisms, working best in asymmetric testing. Interestingly, our asymmetric metric learning approach works best in symmetric testing, allowing the student to even outperform the teacher.
@conference{C117,
title = {Asymmetric metric learning for knowledge transfer},
author = {Budnik, Mateusz and Avrithis, Yannis},
booktitle = {Proceedings of IEEE Conference on Computer Vision and Pattern Recognition (CVPR)},
month = {6},
address = {Virtual},
year = {2021}
}
2020
Virtual Dec 2020
The challenge in few-shot learning is that available data is not enough to capture the underlying distribution. To mitigate this, two emerging directions are (a) using local image representations, essentially multiplying the amount of data by a constant factor, and (b) using more unlabeled data, for instance by transductive inference, jointly on a number of queries. In this work, we bring these two ideas together, introducing local propagation. We treat local image features as independent examples, we build a graph on them and we use it to propagate both the features themselves and the labels, known and unknown. Interestingly, since there is a number of features per image, even a single query gives rise to transductive inference. As a result, we provide a universally safe choice for few-shot inference under both non-transductive and transductive settings, improving accuracy over corresponding methods. This is in contrast to existing solutions, where one needs to choose the method depending on the quantity of available data.
@conference{C116,
title = {Local Propagation for Few-Shot Learning},
author = {Lifchitz, Yann and Avrithis, Yannis and Picard, Sylvaine},
booktitle = {Proceedings of International Conference on Pattern Recognition (ICPR)},
month = {12},
address = {Virtual},
year = {2020}
}
Virtual Dec 2020
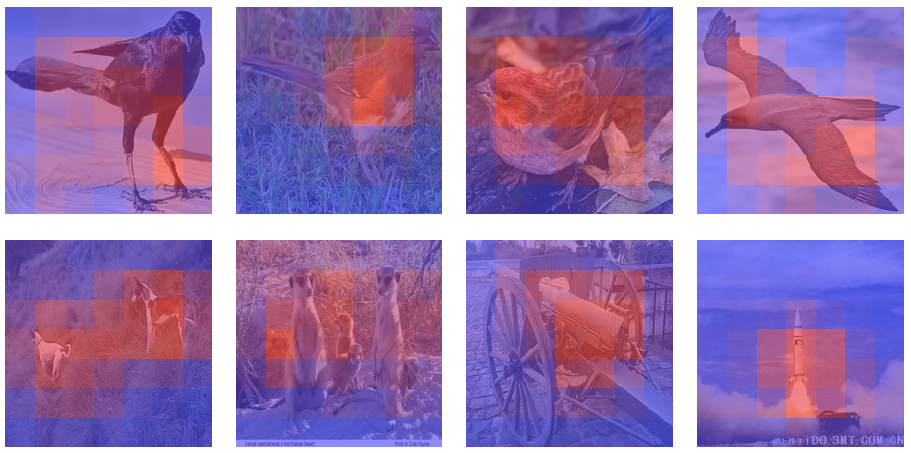
Few-shot learning is often motivated by the ability of humans to learn new tasks from few examples. However, standard few-shot classification benchmarks assume that the representation is learned on a limited amount of base class data, ignoring the amount of prior knowledge that a human may have accumulated before learning new tasks. At the same time, even if a powerful representation is available, it may happen in some domain that base class data are limited or non-existent. This motivates us to study a problem where the representation is obtained from a classifier pre-trained on a large-scale dataset of a different domain, assuming no access to its training process, while the base class data are limited to few examples per class and their role is to adapt the representation to the domain at hand rather than learn from scratch. We adapt the representation in two stages, namely on the few base class data if available and on the even fewer data of new tasks. In doing so, we obtain from the pre-trained classifier a spatial attention map that allows focusing on objects and suppressing background clutter. This is important in the new problem, because when base class data are few, the network cannot learn where to focus implicitly. We also show that a pre-trained network may be easily adapted to novel classes, without meta-learning.
@conference{C115,
title = {Few-Shot Few-Shot Learning and the role of Spatial Attention},
author = {Lifchitz, Yann and Avrithis, Yannis and Picard, Sylvaine},
booktitle = {Proceedings of International Conference on Pattern Recognition (ICPR)},
month = {12},
address = {Virtual},
year = {2020}
}
Virtual Aug 2020
In this work we consider the problem of learning a classifier from noisy labels when a few clean labeled examples are given. The structure of clean and noisy data is modeled by a graph per class and Graph Convolutional Networks (GCN) are used to predict class relevance of noisy examples. For each class, the GCN is treated as a binary classifier, which learns to discriminate clean from noisy examples using a weighted binary cross-entropy loss function. The GCN-inferred "clean" probability is then exploited as a relevance measure. Each noisy example is weighted by its relevance when learning a classifier for the end task. We evaluate our method on an extended version of a few-shot learning problem, where the few clean examples of novel classes are supplemented with additional noisy data. Experimental results show that our GCN-based cleaning process significantly improves the classification accuracy over not cleaning the noisy data, as well as standard few-shot classification where only few clean examples are used.
@conference{C114,
title = {Graph convolutional networks for learning with few clean and many noisy labels},
author = {Iscen, Ahmet and Tolias, Giorgos and Avrithis, Yannis and Chum, Ond\v{r}ej and Schmid, Cordelia},
booktitle = {Proceedings of European Conference on Computer Vision (ECCV)},
month = {8},
address = {Virtual},
year = {2020}
}
Virtual Dec 2020
Active learning typically focuses on training a model on few labeled examples alone, while unlabeled ones are only used for acquisition. In this work we depart from this setting by using both labeled and unlabeled data during model training across active learning cycles. We do so by using unsupervised feature learning at the beginning of the active learning pipeline and semi-supervised learning at every active learning cycle, on all available data. The former has not been investigated before in active learning, while the study of latter in the context of deep learning is scarce and recent findings are not conclusive with respect to its benefit. Our idea is orthogonal to acquisition strategies by using more data, much like ensemble methods use more models. By systematically evaluating on a number of popular acquisition strategies and datasets, we find that the use of unlabeled data during model training brings a spectacular accuracy improvement in image classification, compared to the differences between acquisition strategies. We thus explore smaller label budgets, even one label per class.
@conference{C113,
title = {Rethinking deep active learning: Using unlabeled data at model training},
author = {Sim\'eoni, Oriane and Budnik, Mateusz and Avrithis, Yannis and Gravier, Guillaume},
booktitle = {Proceedings of International Conference on Pattern Recognition (ICPR)},
month = {12},
address = {Virtual},
year = {2020}
}
2019
Long Beach, CA, US Jun 2019
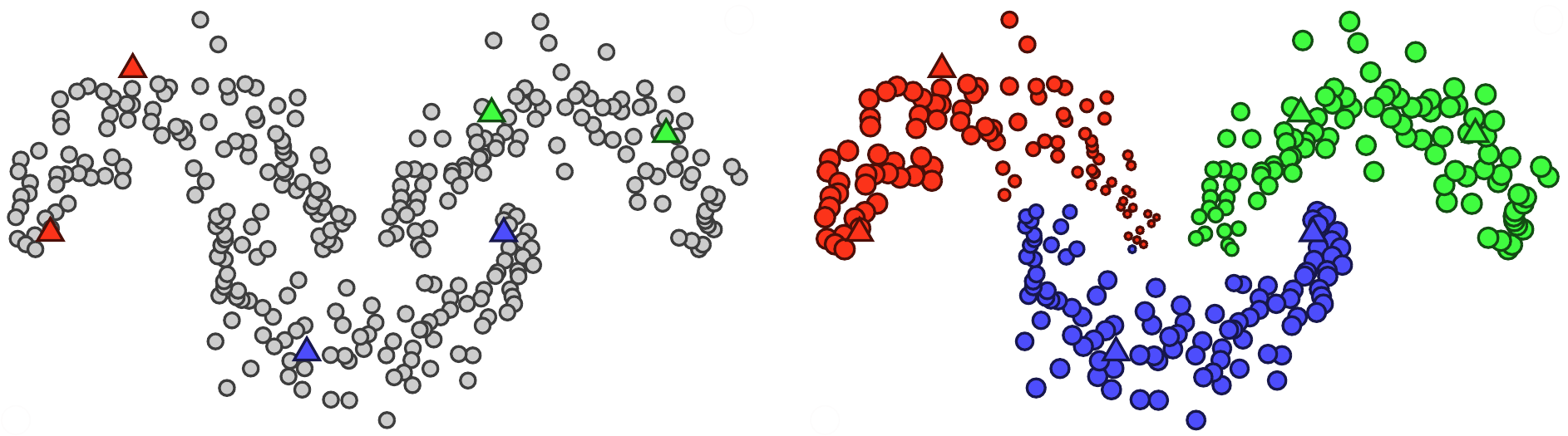
Semi-supervised learning is becoming increasingly important because it can combine data carefully labeled by humans with abundant unlabeled data to train deep neural networks. Classic works on semi-supervised learning that have focused on transductive learning have not been fully exploited in the inductive framework followed by modern deep learning. The same holds for the manifold assumption--that similar examples should get the same prediction. In this work, we employ a transductive method that is based on the manifold assumption to make predictions on the entire dataset and use these predictions to generate pseudo-labels for the unlabeled data and train a deep neural network. In doing so, a nearest neighbor graph of the dataset is created based on the embeddings of the same network. Therefore our learning process iterates between these two steps. We improve performance on several datasets especially in the few labels regime and show that our work is complementary to current state of the art.
@conference{C112,
title = {Label propagation for Deep Semi-supervised Learning},
author = {Iscen, Ahmet and Tolias, Giorgos and Avrithis, Yannis and Chum, Ond\v{r}ej},
booktitle = {Proceedings of IEEE Conference on Computer Vision and Pattern Recognition (CVPR)},
month = {6},
address = {Long Beach, CA, US},
year = {2019}
}
Long Beach, CA, US Jun 2019
Training deep neural networks from few examples is a highly challenging and key problem for many computer vision tasks. In this context, we are targeting knowledge transfer from a set with abundant data to other sets with few available examples. We propose two simple and effective solutions: (i) dense classification over feature maps, which for the first time studies local activations in the domain of few-shot learning, and (ii) implanting, that is, attaching new neurons to a previously trained network to learn new, task-specific features. On miniImageNet, we improve the prior state-of-the-art on few-shot classification, i.e., we achieve 62.5%, 79.8% and 83.8% on 5-way 1-shot, 5-shot and 10-shot settings respectively.
@conference{C111,
title = {Dense Classification and Implanting for Few-shot Learning},
author = {Lifchitz, Yann and Avrithis, Yannis and Picard, Sylvaine and Bursuc, Andrei},
booktitle = {Proceedings of IEEE Conference on Computer Vision and Pattern Recognition (CVPR)},
month = {6},
address = {Long Beach, CA, US},
year = {2019}
}
Long Beach, CA, US Jun 2019
We propose a novel method of spatial verification for image retrieval. Initial ranking is based on image descriptors extracted from convolutional neural network activations by global pooling, as in recent state-of-the-art work. However, the same sparse 3D activation tensor is also approximated by a collection of local features. These local features are then robustly matched to approximate the optimal alignment of the tensors. This happens without any network modification, additional layers or training. No local feature detection happens on the original image; no local feature descriptors and no visual vocabulary are needed throughout the whole process.
We experimentally show that the proposed method achieves the state-of-the-art performance on standard benchmarks across different network architectures and different global pooling methods. Advantages of combining efficient nearest neighbor retrieval with global descriptors and spatial verification is even more pronounced by spatially verified diffusion.
@conference{C110,
title = {Local Features and Visual Words Emerge in Activations},
author = {Sim\'eoni, Oriane and Avrithis, Yannis and Chum, Ond\v{r}ej},
booktitle = {Proceedings of IEEE Conference on Computer Vision and Pattern Recognition (CVPR)},
month = {6},
address = {Long Beach, CA, US},
year = {2019}
}
2018
Perth, Western Australia Dec 2018
State of the art image retrieval performance is achieved with CNN features and manifold ranking using a k-NN similarity graph that is pre-computed off-line. The two most successful existing approaches are temporal filtering, where manifold ranking amounts to solving a sparse linear system online, and spectral filtering, where eigen-decomposition of the adjacency matrix is performed off-line and then manifold ranking amounts to dot-product search online. The former suffers from expensive queries and the latter from significant space overhead. Here we introduce a novel, theoretically well-founded hybrid filtering approach allowing full control of the space-time trade-off between these two extremes. Experimentally, we verify that our hybrid method delivers results on par with the state of the art, with lower memory demands compared to spectral filtering approaches and faster compared to temporal filtering.
@conference{C109,
title = {Hybrid Diffusion: Spectral-Temporal Graph Filtering for Manifold Ranking},
author = {Iscen, Ahmet and Avrithis, Yannis and Tolias, Giorgos and Furon, Teddy and Chum, Ond\v{r}ej},
booktitle = {Proceedings of Asian Conference on Computer Vision (ACCV)},
month = {12},
address = {Perth, Western Australia},
year = {2018}
}
Salt Lake City, UT, US Jun 2018
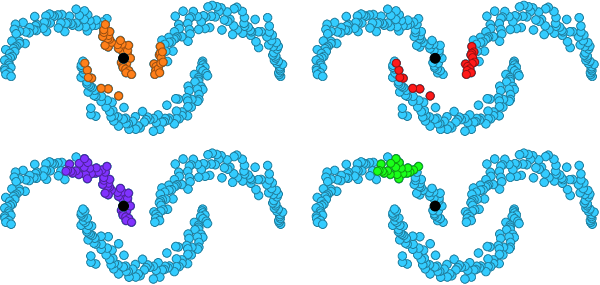
In this work we present a novel unsupervised framework for hard training example mining. The only input to the method is a collection of images relevant to the target application and a meaningful initial representation, provided e.g. by pre-trained CNN. Positive examples are distant points on a single manifold, while negative examples are nearby points on different manifolds. Both types of examples are revealed by disagreements between Euclidean and manifold similarities. The discovered examples can be used in training with any discriminative loss.
The method is applied to unsupervised fine-tuning of pre-trained networks for fine-grained classification and particular object retrieval. Our models are on par or are outperforming prior models that are fully or partially supervised.
@conference{C108,
title = {Mining on Manifolds: Metric Learning without Labels},
author = {Iscen, Ahmet and Tolias, Giorgos and Avrithis, Yannis and Chum, Ond\v{r}ej},
booktitle = {Proceedings of IEEE Conference on Computer Vision and Pattern Recognition (CVPR)},
month = {6},
address = {Salt Lake City, UT, US},
year = {2018}
}
Salt Lake City, UT, US Jun 2018
In this paper we address issues with image retrieval benchmarking on standard and popular Oxford 5k and Paris 6k datasets. In particular, annotation errors, the size of the dataset, and the level of challenge are addressed: new annotation for both datasets is created with an extra attention to the reliability of the ground truth. Three new protocols of varying difficulty are introduced. The protocols allow fair comparison between different methods, including those using a dataset pre-processing stage. For each dataset, 15 new challenging queries are introduced. Finally, a new set of 1M hard, semi-automatically cleaned distractors is selected.
An extensive comparison of the state-of-the-art methods is performed on the new benchmark. Different types of methods are evaluated, ranging from local-feature-based to modern CNN based methods. The best results are achieved by taking the best of the two worlds. Most importantly, image retrieval appears far from being solved.
@conference{C107,
title = {Revisiting {Oxford} and {Paris}: Large-Scale Image Retrieval Benchmarking},
author = {Radenovi\'c, Filip and Iscen, Ahmet and Tolias, Giorgos and Avrithis, Yannis and Chum, Ond\v{r}ej},
booktitle = {Proceedings of IEEE Conference on Computer Vision and Pattern Recognition (CVPR)},
month = {6},
address = {Salt Lake City, UT, US},
year = {2018}
}
Salt Lake City, UT, US Jun 2018
Despite the success of deep learning on representing images for particular object retrieval, recent studies show that the learned representations still lie on manifolds in a high dimensional space. This makes the Euclidean nearest neighbor search biased for this task. Exploring the manifolds online remains expensive even if a nearest neighbor graph has been computed offline.
This work introduces an explicit embedding reducing manifold search to Euclidean search followed by dot product similarity search. This is equivalent to linear graph filtering of a sparse signal in the frequency domain. To speed up online search, we compute an approximate Fourier basis of the graph offline. We improve the state of art on particular object retrieval datasets including the challenging Instre dataset containing small objects. At a scale of 10^5 images, the offline cost is only a few hours, while query time is comparable to standard similarity search.
@conference{C106,
title = {Fast Spectral Ranking for Similarity Search},
author = {Iscen, Ahmet and Avrithis, Yannis and Tolias, Giorgos and Furon, Teddy and Chum, Ond\v{r}ej},
booktitle = {Proceedings of IEEE Conference on Computer Vision and Pattern Recognition (CVPR)},
month = {6},
address = {Salt Lake City, UT, US},
year = {2018}
}
Lake Tahoe, NV/CA, US Mar 2018
Severe background clutter is challenging in many computer vision tasks, including large-scale image retrieval. Global descriptors, that are popular due to their memory and search efficiency, are especially prone to corruption by such a clutter. Eliminating the impact of the clutter on the image descriptor increases the chance of retrieving relevant images and prevents topic drift due to actually retrieving the clutter in the case of query expansion. In this work, we propose a novel salient region detection method. It captures, in an unsupervised manner, patterns that are both discriminative and common in the dataset. Saliency is based on a centrality measure of a nearest neighbor graph constructed from regional CNN representations of dataset images. The descriptors derived from the salient regions improve particular object retrieval, most noticeably in a large collections containing small objects.
@conference{C105,
title = {Unsupervised object discovery for instance recognition},
author = {Sim\'eoni, Oriane and Iscen, Ahmet and Tolias, Giorgos and Avrithis, Yannis and Chum, Ond\v{r}ej},
booktitle = {Proceedings of IEEE Winter Conference on Applications of Computer Vision (WACV)},
month = {3},
address = {Lake Tahoe, NV/CA, US},
year = {2018}
}
2017
part of International Conference on Computer Vision
Venice, Italy Oct 2017
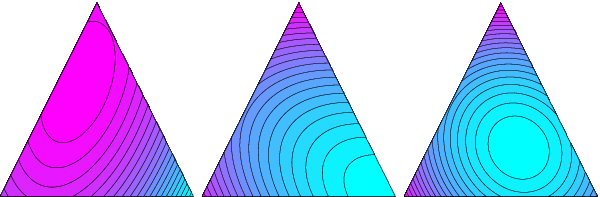
Part-based image classification consists in representing categories by small sets of discriminative parts upon which a representation of the images is built. This paper addresses the question of how to automatically learn such parts from a set of labeled training images. We propose to cast the training of parts as a quadratic assignment problem in which optimal correspondences between image regions and parts are automatically learned. The paper analyses different assignment strategies and thoroughly evaluates them on two public datasets: Willow actions and MIT 67 scenes.
@conference{C104,
title = {Automatic discovery of discriminative parts as a quadratic assignment problem},
author = {Sicre, Ronan and Rabin, Julien and Avrithis, Yannis and Furon, Teddy and Jurie, Fr\'ed\'eric and Kijak, Ewa},
booktitle = {Proceedings of International Workshop on Compact and Efficient Feature Representation and Learning (CEFRL), part of International Conference on Computer Vision (ICCV)},
month = {10},
address = {Venice, Italy},
year = {2017}
}
Honolulu, Hawaii, US Jul 2017
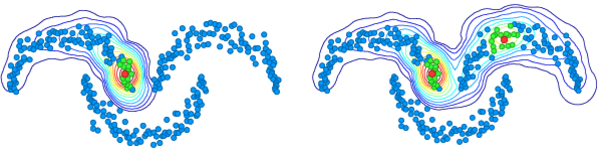
Query expansion is a popular method to improve the quality of image retrieval with both conventional and CNN representations. It has been so far limited to global image similarity. This work focuses on diffusion, a mechanism that captures the image manifold in the feature space. The diffusion is carried out on descriptors of overlapping image regions rather than on a global image descriptor like in previous approaches. An efficient off-line stage allows optional reduction in the number of stored regions. In the on-line stage, the proposed handling of unseen queries in the indexing stage removes additional computation to adjust the precomputed data. A novel way to perform diffusion through a sparse linear system solver yields practical query times well below one second. Experimentally, we observe a significant boost in performance of image retrieval with compact CNN descriptors on standard benchmarks, especially when the query object covers only a small part of the image. Small objects have been a common failure case of CNN-based retrieval.
@conference{C103,
title = {Efficient Diffusion on Region Manifolds: Recovering Small Objects with Compact {CNN} Representations},
author = {Iscen, Ahmet and Tolias, Giorgos and Avrithis, Yannis and Furon, Teddy and Chum, Ond\v{r}ej},
booktitle = {Proceedings of IEEE Conference on Computer Vision and Pattern Recognition (CVPR)},
month = {7},
address = {Honolulu, Hawaii, US},
year = {2017}
}
Honolulu, Hawaii, US Jul 2017
Part-based image classification aims at representing categories by small sets of learned discriminative parts, upon which an image representation is built. Considered as a promising avenue a decade ago, this direction has been neglected since the advent of deep neural networks. In this context, this paper brings two contributions: first, this work proceeds one step further compared to recent part-based models (PBM), focusing on how to learn parts without using any labeled data. Instead of learning a set of parts per class, as generally performed in the PBM literature, the proposed approach both constructs a partition of a given set of images into visually similar groups, and subsequently learns a set of discriminative parts per group in a fully unsupervised fashion. This strategy opens the door to the use of PBM in new applications where labeled data are typically not available, such as instance-based image retrieval. Second, this paper shows that despite the recent success of end-to-end models, explicit part learning can still boost classification performance. We experimentally show that our learned parts can help building efficient image representations, which outperform state-of-the art Deep Convolutional Neural Networks (DCNN) on both classification and retrieval tasks.
@conference{C102,
title = {Unsupervised part learning for visual recognition},
author = {Sicre, Ronan and Avrithis, Yannis and Kijak, Ewa and Jurie, Fr\'ed\'eric},
booktitle = {Proceedings of IEEE Conference on Computer Vision and Pattern Recognition (CVPR)},
month = {7},
address = {Honolulu, Hawaii, US},
year = {2017}
}
Bucharest, Romania Jun 2017
Location recognition is commonly treated as visual instance retrieval on "street view" imagery. The dataset items and queries are panoramic views, i.e. groups of images taken at a single location. This work introduces a novel panorama-to-panorama matching process, either by aggregating features of individual images in a group or by explicitly constructing a larger panorama. In either case, multiple views are used as queries. We reach near perfect location recognition on a standard benchmark with only four query views.
@conference{C101,
title = {Panorama to Panorama Matching for Location Recognition},
author = {Iscen, Ahmet and Tolias, Giorgos and Avrithis, Yannis and Furon, Teddy and Chum, Ond\v{r}ej},
booktitle = {Proceedings of ACM International Conference on Multimedia Retrieval (ICMR)},
month = {6},
address = {Bucharest, Romania},
year = {2017}
}
2016
Heraklion, Greece Jun 2016
We propose a new data-structure, the generalized randomized k-d forest, or k-d GeRaF, for approximate nearest neighbor searching in high dimensions. In particular, we introduce new randomization techniques to specify a set of independently constructed trees where search is performed simultaneously, hence increasing accuracy. We omit backtracking, and we optimize distance computations, thus accelerating queries. We release public domain software GeRaF and we compare it to existing implementations of state-of-the-art methods including BBD-trees, Locality Sensitive Hashing, randomized k-d forests, and product quantization. Experimental results indicate that our method would be the method of choice in dimensions around 1,000, and probably up to 10,000, and pointsets of cardinality up to a few hundred thousands or even one million; this range of inputs is encountered in many critical applications today. For instance, we handle a real dataset of 10^6 images represented in 960 dimensions with a query time of less than 1sec on average and 90% responses being true nearest neighbors.
@conference{C100,
title = {High-Dimensional Visual Similarity Search: {k-d} Generalized Randomized Forests},
author = {Avrithis, Yannis and Emiris, Ioannis and Samaras, Georgios},
booktitle = {Proceedings of the 33rd Computer Graphics International (CGI)},
month = {6},
address = {Heraklion, Greece},
year = {2016},
organization = {ACM},
pages = {25--28}
}
2015
Santiago, Chile Dec 2015
Large scale duplicate detection, clustering and mining of documents or images has been conventionally treated with seed detection via hashing, followed by seed growing heuristics using fast search. Principled clustering methods, especially kernelized and spectral ones, have higher complexity and are difficult to scale above millions. Under the assumption of documents or images embedded in Euclidean space, we revisit recent advances in approximate k-means variants, and borrow their best ingredients to introduce a new one, inverted-quantized k-means (IQ-means). Key underlying concepts are quantization of data points and multi-index based inverted search from centroids to cells. Its quantization is a form of hashing and analogous to seed detection, while its updates are analogous to seed growing, yet principled in the sense of distortion minimization. We further design a dynamic variant that is able to determine the number of clusters k in a single run at nearly zero additional cost. Combined with powerful deep learned representations, we achieve clustering of a 100 million image collection on a single machine in less than one hour.
@conference{C99,
title = {Web-scale image clustering revisited},
author = {Avrithis, Yannis and Kalantidis, Yannis and Anagnostopoulos, Evangelos and Emiris, Ioannis},
booktitle = {Proceedings of International Conference on Computer Vision (ICCV) (Oral)},
month = {12},
address = {Santiago, Chile},
year = {2015}
}
Swansea, UK Sep 2015
We present a very simple computational model for planar shape decomposition that naturally captures most of the rules and salience measures suggested by psychophysical studies, including the minima and short-cut rules, convexity, and symmetry. It is based on a medial axis representation in ways that have not been explored before and sheds more light into the connection between existing rules like minima and convexity. In particular, vertices of the exterior medial axis directly provide the position and extent of negative minima of curvature, while a traversal of the interior medial axis directly provides a small set of candidate endpoints for part-cuts. The final selection follows a simple local convexity rule that can incorporate arbitrary salience measures. Neither global optimization nor differentiation is involved. We provide qualitative and quantitative evaluation and comparisons on ground-truth data from psychophysical experiments.
@conference{C98,
title = {Planar shape decomposition made simple},
author = {Papanelopoulos, Nikos and Avrithis, Yannis},
booktitle = {Proceedings of British Machine Vision Conference (BMVC)},
month = {9},
address = {Swansea, UK},
year = {2015}
}
Boston, MA, US Jun 2015
Recent works show that image comparison based on local descriptors is corrupted by visual bursts, which tend to dominate the image similarity. The existing strategies, like power-law normalization, improve the results by discounting the contribution of visual bursts to the image similarity.
In this paper, we propose to explicitly detect the visual bursts in an image at an early stage. We compare several detection strategies jointly taking into account feature similarity and geometrical quantities. The bursty groups are merged into meta-features, which are used as input to state-of-the-art image search systems such as VLAD or the selective match kernel. Then, we show the interest of using this strategy in an asymmetrical manner, with only the database features being aggregated but not those of the query.
Extensive experiments performed on public benchmarks for visual retrieval show the benefits of our method, which achieves performance on par with the state of the art but with a significantly reduced complexity, thanks to the lower number of features fed to the indexing system.
@conference{C97,
title = {Early burst detection for memory-efficient image retrieval},
author = {Shi, Miaojing and Avrithis, Yannis and J\'egou, Herv\'e},
booktitle = {Proceedings of IEEE Conference on Computer Vision and Pattern Recognition (CVPR)},
month = {6},
address = {Boston, MA, US},
year = {2015}
}
2014
Singapore Nov 2014
The recent trend of structure-guided feature detectors, as opposed to blob and corner detectors, has led to a family of methods that exploit image edges to accurately capture local shape. Among them, the WaSH detector combines binary edge sampling with gradient strength and computational geometry representations towards distinctive and repeatable local features. In this work, we provide alternative, variable-density sampling schemes on smooth functions of image intensity based on dithering. These methods are parameter-free and more invariant to geometric transformations than uniform sampling. The resulting detectors compare well to the state-of-the-art, while achieving higher performance in a series of matching and retrieval experiments.
@conference{C96,
title = {Improving local features by dithering-based image sampling},
author = {Varytimidis, Christos and Rapantzikos, Konstantinos and Avrithis, Yannis and Kollias, Stefanos},
booktitle = {Proceedings of Asian Conference on Computer Vision (ACCV)},
month = {11},
address = {Singapore},
year = {2014}
}
Columbus, OH, US Jun 2014
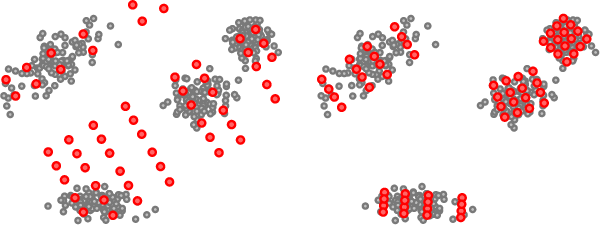
We present a simple vector quantizer that combines low distortion with fast search and apply it to approximate nearest neighbor (ANN) search in high dimensional spaces. Leveraging the very same data structure that is used to provide non-exhaustive search, i.e. inverted lists or a multi-index, the idea is to locally optimize an individual product quantizer (PQ) per cell and use it to encode residuals. Local optimization is over rotation and space decomposition; interestingly, we apply a parametric solution that assumes a normal distribution and is extremely fast to train. With a reasonable space and time overhead that is constant in the data size, we set a new state-of-the-art on several public datasets, including a billion-scale one.
@conference{C95,
title = {Locally Optimized Product Quantization for Approximate Nearest Neighbor Search},
author = {Kalantidis, Yannis and Avrithis, Yannis},
booktitle = {Proceedings of IEEE Conference on Computer Vision and Pattern Recognition (CVPR)},
month = {6},
address = {Columbus, OH, US},
year = {2014}
}
2013
Sydney, Australia Dec 2013
Inspired by the close relation between nearest neighbor search and clustering in high-dimensional spaces as well as the success of one helping to solve the other, we introduce a new paradigm where both problems are solved simultaneously. Our solution is recursive, not in the size of input data but in the number of dimensions. One result is a clustering algorithm that is tuned to small codebooks but does not need all data in memory at the same time and is practically constant in the data size. As a by-product, a tree structure performs either exact or approximate quantization on trained centroids, the latter being not very precise but extremely fast. A lesser contribution is a new indexing scheme for image retrieval that exploits multiple small codebooks to provide an arbitrarily fine partition of the descriptor space. Large scale experiments on public datasets exhibit state of the art performance and remarkable generalization.
@conference{C94,
title = {Quantize and Conquer: A dimensionality-recursive solution to clustering, vector quantization, and image retrieval},
author = {Avrithis, Yannis},
booktitle = {Proceedings of International Conference on Computer Vision (ICCV)},
month = {12},
address = {Sydney, Australia},
year = {2013}
}
Sydney, Australia Dec 2013
This paper considers a family of metrics to compare images based on their local descriptors. It encompasses the VLAD descriptor and matching techniques such as Hamming Embedding. Making the bridge between these approaches leads us to propose a match kernel that takes the best of existing techniques by combining an aggregation procedure with a selective match kernel. Finally, the representation underpinning this kernel is approximated, providing a large scale image search both precise and scalable, as shown by our experiments on several benchmarks.
@conference{C93,
title = {To aggregate or not to aggregate: selective match kernels for image search},
author = {Tolias, Giorgos and Avrithis, Yannis and J\'egou, Herv\'e},
booktitle = {Proceedings of International Conference on Computer Vision (ICCV) (Oral)},
month = {12},
address = {Sydney, Australia},
year = {2013}
}
2012
Florence, Italy Oct 2012
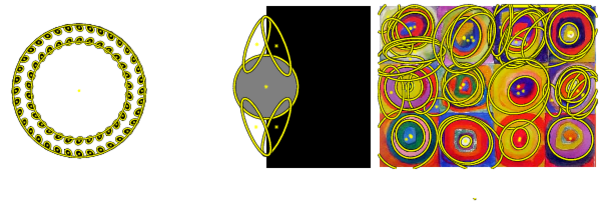
Depending on the application, local feature detectors should comply to properties that are often contradictory, e.g. distinctiveness vs robustness. Providing a good balance is a standing problem in the field. In this direction, we propose a novel approach for local feature detection starting from sampled edges and based on shape stability measures across the weighted alpha-filtration, a computational geometry construction that captures the shape of a non-uniform set of points. Detected features are blob-like and include non-extremal regions as well as regions determined by cavities of boundary shape. The detector provides distinctive regions, while achieving high robustness in terms of repeatability and matching score, as well as competitive performance in a large scale image retrieval application.
@conference{C92,
title = {W$\alpha$SH: Weighted $\alpha$-Shapes for Local Feature Detection},
author = {Varytimidis, Christos and Rapantzikos, Konstantinos and Avrithis, Yannis},
booktitle = {Proceedings of European Conference on Computer Vision (ECCV)},
month = {10},
address = {Florence, Italy},
year = {2012}
}
Florence, Italy Oct 2012
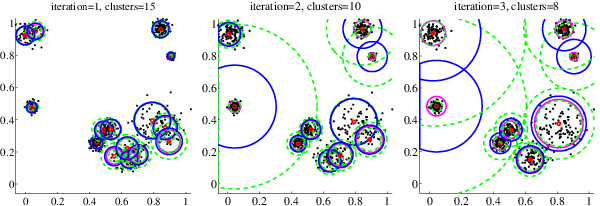
We introduce a clustering method that combines the flexibility of Gaussian mixtures with the scaling properties needed to construct visual vocabularies for image retrieval. It is a variant of expectation-maximization that can converge rapidly while dynamically estimating the number of components. We employ approximate nearest neighbor search to speed-up the E-step and exploit its iterative nature to make search incremental, boosting both speed and precision. We achieve superior performance in large scale retrieval, being as fast as the best known approximate k-means.
@conference{C91,
title = {Approximate {Gaussian} Mixtures for Large Scale Vocabularies},
author = {Avrithis, Yannis and Kalantidis, Yannis},
booktitle = {Proceedings of European Conference on Computer Vision (ECCV)},
month = {10},
address = {Florence, Italy},
year = {2012}
}
Nara, Japan Oct 2012
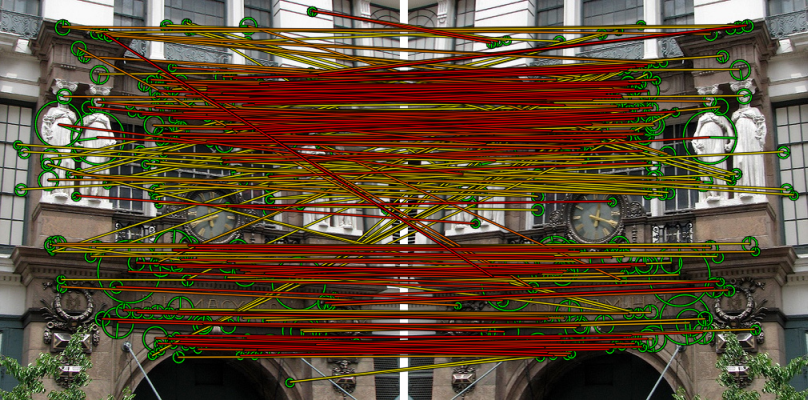
Many problems, including feature selection, vocabulary learning, location and landmark recognition, structure from motion and 3d reconstruction, rely on a learning process that involves wide-baseline matching on multiple views of the same object or scene. In practical large scale image retrieval applications however, most images depict unique views where this idea does not apply. We exploit self-similarities, symmetries and repeating patterns to select features within a single image. We achieve the same performance compared to the full feature set with only a small fraction of its index size on a dataset of unique views of buildings or urban scenes, in the presence of one million distractors of similar nature. Our best solution is linear in the number of correspondences, with practical running times of just a few milliseconds.
@conference{C90,
title = {{SymCity}: Feature Selection by Symmetry for Large Scale Image Retrieval},
author = {Tolias, Giorgos and Kalantidis, Yannis and Avrithis, Yannis},
publisher = {ACM},
booktitle = {Proceedings of ACM Multimedia Conference (ACM-MM) (Full paper)},
month = {10},
address = {Nara, Japan},
year = {2012}
}
2011
Barcelona, Spain Nov 2011
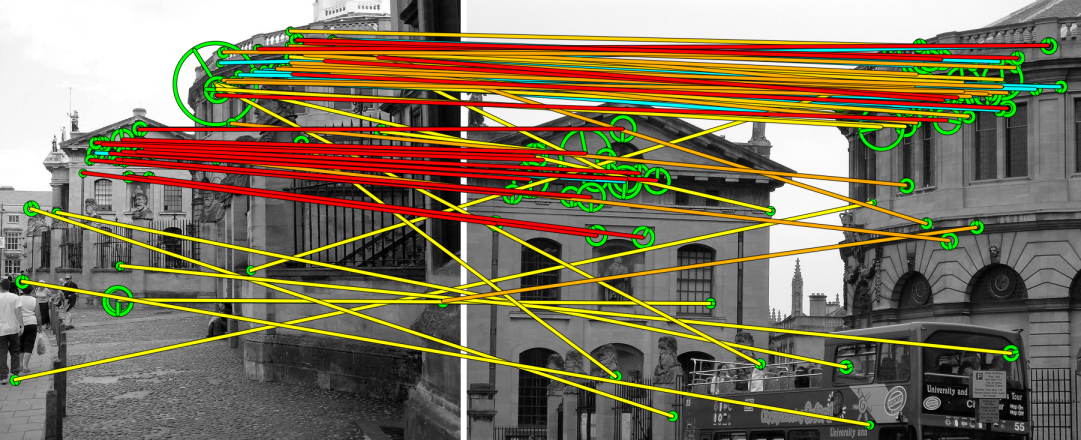
A wide range of properties and assumptions determine the most appropriate spatial matching model for an application, e.g. recognition, detection, registration, or large scale visual search. Most notably, these include discriminative power, geometric invariance, rigidity constraints, mapping constraints, assumptions made on the underlying features or descriptors and, of course, computational complexity. Having image retrieval in mind, we present a very simple model inspired by Hough voting in the transformation space, where votes arise from single feature correspondences. A relaxed matching process allows for multiple matching surfaces or non-rigid objects under one-to-one mapping, yet is linear in the number of correspondences. We apply it to geometry re-ranking in a search engine, yielding superior performance with the same space requirements but a dramatic speed-up compared to the state of the art.
@conference{C89,
title = {Speeded-up, Relaxed Spatial Matching},
author = {Tolias, Giorgos and Avrithis, Yannis},
booktitle = {Proceedings of International Conference on Computer Vision (ICCV)},
month = {11},
address = {Barcelona, Spain},
year = {2011}
}
Barcelona, Spain Nov 2011
We present a local feature detector that is able to detect regions of arbitrary scale and shape, without scale space construction. We compute a weighted distance map on image gradient, using our exact linear-time algorithm, a variant of group marching for Euclidean space. We find the weighted medial axis by extending residues, typically used in Voronoi skeletons. We decompose the medial axis into a graph representing image structure in terms of peaks and saddle points. A duality property enables reconstruction of regions using the same marching method. We greedily group regions taking both contrast and shape into account. On the way, we select regions according to our shape fragmentation factor, favoring those well enclosed by boundaries--even incomplete. We achieve state of the art performance in matching and retrieval experiments with reduced memory and computational requirements.
@conference{C88,
title = {The Medial Feature Detector: Stable Regions from Image Boundaries},
author = {Avrithis, Yannis and Rapantzikos, Konstantinos},
booktitle = {Proceedings of International Conference on Computer Vision (ICCV)},
month = {11},
address = {Barcelona, Spain},
year = {2011}
}
Trento, Italy Apr 2011
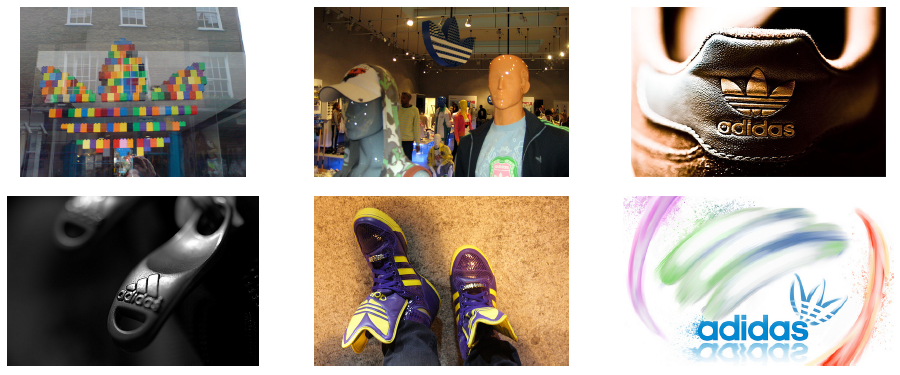
We propose a scalable logo recognition approach that extends the common bag-of-words model and incorporates local geometry in the indexing process. Given a query image and a large logo database, the goal is to recognize the logo contained in the query, if any. We locally group features in triples using multi-scale Delaunay triangulation and represent triangles by signatures capturing both visual appearance and local geometry. Each class is represented by the union of such signatures over all instances in the class. We see large scale recognition as a sub-linear search problem where signatures of the query image are looked up in an inverted index structure of the class models. We evaluate our approach on a large-scale logo recognition dataset with more than four thousand classes.
@conference{C87,
title = {Scalable Triangulation-based Logo Recognition},
author = {Kalantidis, Yannis and Pueyo, Lluis Garcia and Trevisiol, Michele and van Zwol, Roelof and Avrithis, Yannis},
booktitle = {Proceedings of ACM International Conference on Multimedia Retrieval (ICMR)},
month = {4},
address = {Trento, Italy},
year = {2011}
}
2010
Firenze, Italy Oct 2010
State of the art data mining and image retrieval in community photo collections typically focus on popular subsets, e.g. images containing landmarks or associated to Wikipedia articles. We propose an image clustering scheme that, seen as vector quantization, compresses a large corpus of images by grouping visually consistent ones while providing a guaranteed distortion bound. This allows us, for instance, to represent the visual content of all thousands of images depicting the Parthenon in just a few dozens of scene maps and still be able to retrieve any single, isolated, non-landmark image like a house or a graffiti on a wall. Starting from a geo-tagged dataset, we first group images geographically and then visually, where each visual cluster is assumed to depict different views of the the same scene. We align all views to one reference image and construct a 2D scene map by preserving details from all images while discarding repeating visual features. Our indexing, retrieval and spatial matching scheme then operates directly on scene maps. We evaluate the precision of the proposed method on a challenging one-million urban image dataset.
@conference{C86,
title = {Retrieving Landmark and Non-Landmark Images from Community Photo Collections},
author = {Avrithis, Yannis and Kalantidis, Yannis and Tolias, Giorgos and Spyrou, Evaggelos},
booktitle = {Proceedings of ACM Multimedia Conference (ACM-MM) (Full paper)},
month = {10},
address = {Firenze, Italy},
year = {2010}
}
Firenze, Italy Oct 2010
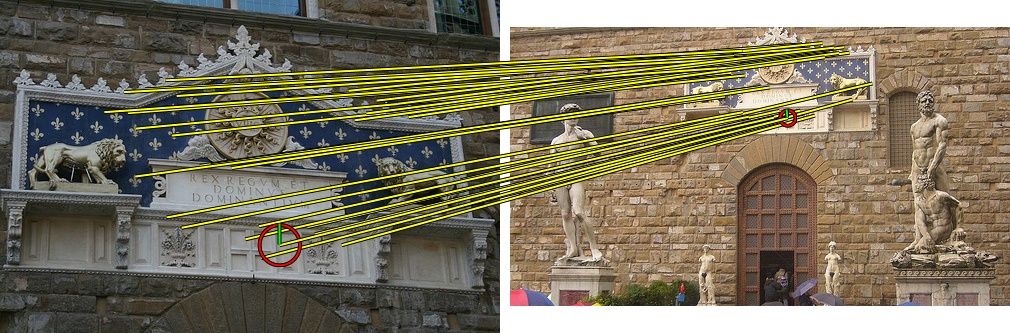
We present a new approach to image indexing and retrieval, which integrates appearance with global image geometry in the indexing process, while enjoying robustness against viewpoint change, photometric variations, occlusion, and background clutter. We exploit shape parameters of local features to estimate image alignment via a single correspondence. Then, for each feature, we construct a sparse spatial map of all remaining features, encoding their normalized position and appearance, typically vector quantized to visual word. An image is represented by a collection of such feature maps and RANSAC-like matching is reduced to a number of set intersections. Because the induced dissimilarity is still not a metric, we extend min-wise independent permutations to collections of sets and derive a similarity measure for feature map collections. We then exploit sparseness to build an inverted file whereby the retrieval process is sub-linear in the total number of images, ideally linear in the number of relevant ones. We achieve excellent performance on 10^4 images, with a query time in the order of milliseconds.
@conference{C85,
title = {Feature Map Hashing: Sub-linear Indexing of Appearance and Global Geometry},
author = {Avrithis, Yannis and Tolias, Giorgos and Kalantidis, Yannis},
booktitle = {Proceedings of ACM Multimedia Conference (ACM-MM) (Full paper)},
month = {10},
address = {Firenze, Italy},
year = {2010}
}
part of European Conference on Computer Vision
Hersonissos, Crete, Greece Sep 2010
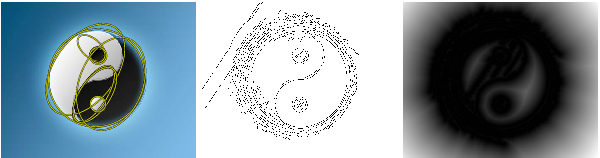
We believe that the potential of edges in local feature detection has not been fully exploited and therefore propose a detector that starts from single scale edges and produces reliable and interpretable blob-like regions and groups of regions of arbitrary shape. The detector is based on merging local maxima of the distance transform guided by the gradient strength of the surrounding edges. Repeatability and matching score are evaluated and compared to state-of-the-art detectors on standard benchmarks. Furthermore, we demonstrate the potential application of our method to wide-baseline matching and feature detection in sequences involving human activity.
@conference{C84,
title = {Detecting Regions from Single Scale Edges},
author = {Rapantzikos, Konstantinos and Avrithis, Yannis and Kollias, Stefanos},
booktitle = {Proceedings of International Workshop on Sign, Gesture and Activity (SGA), part of European Conference on Computer Vision (ECCV)},
month = {9},
address = {Hersonissos, Crete, Greece},
year = {2010}
}
2009
Miami, FL, US Jun 2009
Several spatiotemporal feature point detectors have been recently used in video analysis for action recognition. Feature points are detected using a number of measures, namely saliency, cornerness, periodicity, motion activity etc. Each of these measures is usually intensity-based and provides a different trade-off between density and informativeness. In this paper, we use saliency for feature point detection in videos and incorporate color and motion apart from intensity. Our method uses a multi-scale volumetric representation of the video and involves spatiotemporal operations at the voxel level. Saliency is computed by a global minimization process constrained by pure volumetric constraints, each of them being related to an informative visual aspect, namely spatial proximity, scale and feature similarity (intensity, color, motion). Points are selected as the extrema of the saliency response and prove to balance well between density and informativeness. We provide an intuitive view of the detected points and visual comparisons against state-of-the-art space-time detectors. Our detector outperforms them on the KTH dataset using Nearest-Neighbor classifiers and ranks among the top using different classification frameworks. Statistics and comparisons are also performed on the more difficult Hollywood Human Actions (HOHA) dataset increasing the performance compared to current published results.
@conference{C83,
title = {Dense saliency-based spatiotemporal feature points for action recognition},
author = {Rapantzikos, Konstantinos and Avrithis, Yannis and Kollias, Stefanos},
booktitle = {Proceedings of IEEE Conference on Computer Vision and Pattern Recognition (CVPR)},
month = {6},
address = {Miami, FL, US},
year = {2009}
}
Taipei, Taiwan Apr 2009
Detection of perceptually important video events is formulated here on the basis of saliency models for the audio, visual and textual information conveyed in a video stream. Audio saliency is assessed by cues that quantify multifrequency waveform modulations, extracted through nonlinear operators and energy tracking. Visual saliency is measured through a spatiotemporal attention model driven by intensity, color and motion. Text saliency is extracted from part-of-speech tagging on the subtitles information available with most movie distributions. The various modality curves are integrated in a single attention curve, where the presence of an event may be signified in one or multiple domains. This multimodal saliency curve is the basis of a bottom-up video summarization algorithm, that refines results from unimodal or audiovisual-based skimming. The algorithm performs favorably for video summarization in terms of informativeness and enjoyability.
@conference{C82,
title = {Video event detection and summarization using audio, visual and text saliency},
author = {Evangelopoulos, Georgios and Zlatintsi, Athanasia and Skoumas, Georgios and Rapantzikos, Konstantinos and Potamianos, Alexandros and Maragos, Petros and Avrithis, Yannis},
booktitle = {Proceedings of IEEE International Conference on Acoustics, Speech and Signal Processing (ICASSP)},
month = {4},
address = {Taipei, Taiwan},
year = {2009}
}
Sophia Antipolis, France Jan 2009
In this paper we propose a methodology for semantic indexing of images, based on techniques of image segmentation, classification and fuzzy reasoning. The proposed knowledge-assisted analysis architecture integrates algorithms applied on three overlapping levels of semantic information: i) no semantics, i.e. segmentation based on low-level features such as color and shape, ii) mid-level semantics, such as concurrent image segmentation and object detection, region-based classification and, iii) rich semantics, i.e. fuzzy reasoning for extraction of implicit knowledge. In that way, we extract semantic description of raw multimedia content and use it for indexing and retrieval purposes, backed up by a fuzzy knowledge repository. We conducted several experiments to evaluate each technique, as well as the whole methodology in overall and, results show the potential of our approach.
@conference{C81,
title = {Integrating Image Segmentation and Classification for Fuzzy Knowledge-based Multimedia Indexing},
author = {Athanasiadis, Thanos and Simou, Nikolaos and Papadopoulos, Georgios and Benmokhtar, Rachid and Chandramouli, Krishna and Tzouvaras, Vassilis and Mezaris, Vasileios and Phinikettos, Marios and Avrithis, Yannis and Kompatsiaris, Yiannis and Huet, Benoit and Izquierdo, Ebroul},
booktitle = {Proceedings of 15th International Multimedia Modeling Conference (MMM)},
month = {1},
pages = {263--274},
address = {Sophia Antipolis, France},
year = {2009}
}
Sophia Antipolis, France Jan 2009
This paper presents an approach on high-level feature detection within video documents, using a Region Thesaurus. A video shot is represented by a single keyframe and MPEG-7 features are extracted locally, from coarse segmented regions. Then a clustering algorithm is applied on those extracted regions and a region thesaurus is constructed to facilitate the description of each keyframe at a higher level than the low-level descriptors but at a lower than the high-level concepts. A model vector representation is formed and several high-level concept detectors are appropriately trained using a global keyframe annotation. The proposed approach is thoroughly evaluated on the TRECVID 2007 development data for the detection of nine high level concepts, demonstrating sufficient performance on large data sets.
@conference{C80,
title = {Large Scale Concept Detection in Video Using a Region Thesaurus},
author = {Spyrou, Evaggelos and Tolias, Giorgos and Avrithis, Yannis},
booktitle = {Proceedings of 15th International Multimedia Modeling Conference (MMM)},
month = {1},
address = {Sophia Antipolis, France},
year = {2009}
}
Chania, Greece Jun 2009
A cross media analysis scheme for the semantic interpretation of compound documents is presented. The proposed scheme is essentially a late-fusion mechanism that operates on top of single-media extractors output. Evidence extracted from heterogeneous sources are used to trigger probabilistic inference on a Bayesian network that encodes domain knowledge and quantifies causality. Experiments performed on a set of 54 compound documents showed that the proposed scheme is able to exploit the existing cross media relations and achieve performance improvements.
@conference{C79,
title = {Compound document analysis by fusing evidence across media},
author = {Nikolopoulos, Spiros and Lakka, Christina and Kompatsiaris, Ioannis and Varytimidis, Christos and Rapantzikos, Konstantinos and Avrithis, Yannis},
booktitle = {Proceedings of 7th International Workshop on Content-Based Multimedia Indexing (CBMI)},
month = {6},
address = {Chania, Greece},
year = {2009}
}
Chania, Greece Jun 2009
The popularity of social networks and web-based personal image collections has resulted to a continuously growing volume of publicly available photos and videos. Users are uploading, describing, tagging and annotating their personal photos. Moreover, a recent trend is to also "geotag" them, that is to mark the location they were taken onto a web-based map. Consequently, this growth of image collections has created the need for fast, robust and efficient systems, able to analyze large-scale diverse and heterogeneous visual content. This growing need for automatic metadata generation, concept detection, search and retrieval has boosted research efforts towards these directions. The work presented herein is a web-based system that aims not only to the retrieval of visually similar images, but also to determine the location they were taken by exploiting the available socially created metadata. This system makes use of a visual vocabulary and a bag-of words approach, in order to describe the visual properties of an image. Moreover, geometric constraints are applied, in order to extend the bag-of-words model towards more accurate results. We begin by describing some related work in the field of image retrieval, in order to present both the relation and the novelties of the presented system in comparison with the existing techniques.
@conference{C78,
title = {Visual Image Retrieval and Localization},
author = {Kalantidis, Yannis and Tolias, Giorgos and Spyrou, Evaggelos and Mylonas, Phivos and Avrithis, Yannis},
booktitle = {Proceedings of 7th International Workshop on Content-Based Multimedia Indexing (CBMI)},
month = {6},
address = {Chania, Greece},
year = {2009}
}
2008
San Diego, CA, US Oct 2008
Based on perceptual and computational attention modeling studies, we formulate measures of saliency for an audiovisual stream. Audio saliency is captured by signal modulations and related multifrequency band features, extracted through nonlinear operators and energy tracking. Visual saliency is measured by means of a spatiotemporal attention model driven by various feature cues (intensity, color, motion). Audio and video curves are integrated in a single attention curve, where events may be enhanced, suppressed or vanished. The presence of salient events is signified on this audiovisual curve by geometrical features such as local extrema, sharp transition points and level sets. An audiovisual saliency-based movie summarization algorithm is proposed and evaluated. The algorithm is shown to perform very well in terms of summary informativeness and enjoyability for movie clips of various genres.
@conference{C77,
title = {Movie Summarization Based on Audiovisual Saliency Detection},
author = {Evangelopoulos, Georgios and Rapantzikos, Konstantinos and Potamianos, Alexandros and Maragos, Petros and Zlatintsi, Athanasia and Avrithis, Yannis},
booktitle = {Proceedings of 15th International Conference on Image Processing (ICIP)},
month = {10},
address = {San Diego, CA, US},
year = {2008}
}
part of International Conference on Image Processing
San Diego, CA, US Oct 2008
In this paper we focus on scene classification and detection of high-level concepts within multimedia documents, by introducing an intermediate contextual approach as a means of exploiting the visual context of images. More specifically, we introduce and model a novel relational knowledge representation, founded on topological and semantic relations between the concepts of an image. We further develop an algorithm
to address computationally efficient handling of visual context and extraction of mid-level region characteristics. Based on the proposed knowledge model, we combine the notion of visual context with region semantics, in order to exploit their efficacy in dealing with scene classification problems. Finally, initial experimental results are presented, in order to demonstrate possible applications of the proposed methodology.
@conference{C76,
title = {Using Region Semantics And Visual Context For Scene Classification},
author = {Spyrou, Evaggelos and Mylonas, Phivos and Avrithis, Yannis},
booktitle = {Proceedings of 1st Workshop on Multimedia Information Retrieval: New Trends and Challenges (MIR), part of International Conference on Image Processing (ICIP)},
month = {10},
address = {San Diego, CA, US},
year = {2008}
}
Cairns, Australia Oct 2008
In this paper, we propose a framework to extend semantic labeling of images to video shot sequences and achieve efficient and semantic-aware spatiotemporal video segmentation. This task faces two major challenges, namely the temporal variations within a video sequence which affect image segmentation and labeling, and the computational cost of region labeling. Guided by these limitations, we design a method where spatiotemporal segmentation and object labeling are coupled to achieve semantic annotation of video shots. An internal graph structure that describes both visual and semantic properties of image and video regions is adopted. The process of spatiotemporal semantic segmentation is subdivided in two stages: Firstly, the video shot is split into small block of frames. Spatiotemporal regions (volumes) are extracted and labeled individually within each block. Then, we iteratively merge consecutive blocks by a matching procedure which considers both semantic and visual properties. Results on real video sequences show the potential of our approach.
@conference{C75,
title = {Spatiotemporal Semantic Video Segmentation},
author = {Galmar, Eric and Athanasiadis, Thanos and Huet, Benoit and Avrithis, Yannis},
publisher = {IEEE},
booktitle = {Proceedings of 10th International Workshop on Multimedia Signal Processing (MMSP)},
month = {10},
address = {Cairns, Australia},
year = {2008}
}
London, UK Jun 2008
Personalized content retrieval aims at improving the retrieval process by taking into account the particular interests of individual users. However, not all user preferences are relevant in all situations. It is well known that human preferences are complex, multiple, heterogeneous, changing, even contradictory, and should be understood in context with the user goals and tasks at hand. In this paper we propose a method to build a dynamic representation of the semantic context of ongoing retrieval tasks, which is used to activate different subsets of user interests at runtime, in such a way that out of context preferences are discarded. Our approach is based on an ontology-driven representation of the domain of discourse, providing enriched descriptions of the semantics involved in retrieval actions and preferences, and enabling the definition of effective means to relate preferences and context.
@conference{C74,
title = {Regions Of Interest for Accurate Object Detection},
author = {Kapsalas, Petros and Rapantzikos, Konstantinos and Sofou, Anastasia and Avrithis, Yannis},
booktitle = {Proceedings of 6th International Workshop on Content-Based Multimedia Indexing (CBMI)},
month = {6},
address = {London, UK},
year = {2008}
}
London, UK Jun 2008
In this paper, an affine invariant curve matching method using curvature scale-space and normalization is proposed. Prior to curve matching, curve normalization with respect to affine transformations is applied, allowing a lossless affine invariant curve representation. The maxima points of the curvature scale-space (CSS) image are then used to represent the normalized curve, while retaining the local properties of the curve. The matching algorithm that follows, matches the maxima sets of CSS images and the resulting matching cost provides a measure of similarity. The method's performance and robustness is evaluated through a variety of curves and affine transformations, obtaining precise shape similarity and retrieval.
@conference{C73,
title = {Affine invariant curve matching using normalization and curvature scale-space},
author = {Giannekou, Vicky and Tzouveli, Paraskevi and Avrithis, Yannis and Kollias, Stefanos},
booktitle = {Proceedings of 6th International Workshop on Content-Based Multimedia Indexing (CBMI)},
month = {6},
address = {London, UK},
year = {2008}
}
Delft, The Netherlands Jun 2008
The notion of context plays a significant role in multimedia content search and retrieval systems. In this paper we focus our research efforts on a visual context knowledge representation, to be utilized for multimedia high-level concept detection. We propose and describe in detail types of contextual relations evident within the multimedia content, model them and provide a clear methodology on how to extract them. A visual context ontology is introduced, containing relations among different types of content entities, such as images, regions, region types and high-level concepts. In this manner, we facilitate traditional object detection approaches towards semantical interpretation. The application of the proposed knowledge structure provides encouraging initial results, improving the efficacy of related multimedia analysis techniques.
@conference{C72,
title = {A Visual Context Ontology for Multimedia High-Level Concept Detection},
author = {Spyrou, Evaggelos and Mylonas, Phivos and Avrithis, Yannis},
booktitle = {Proceedings of 5th International Workshop in Modeling and Reasoning in Context (MRC)},
month = {6},
address = {Delft, The Netherlands},
year = {2008}
}
Klagenfurt, Austria May 2008
This paper presents an approach on high-level feature detection within video documents, using a Region Thesaurus and Latent Semantic Analysis. A video shot is represented by a single keyframe. MPEG-7 features are extracted from coarse regions of it. A clustering algorithm is applied on all extracted regions and a region thesaurus is constructed. Its use is to assist to the mapping of low- to high-level features by a model vector representation. Latent Semantic Analysis is then applied on the model vectors to exploit the latent relations among regions types aiming to improve detection performance. The proposed approach is thoroughly examined using TRECVID 2007 development data.
@conference{C71,
title = {A Semantic Multimedia Analysis Approach Utilizing a Region Thesaurus and {LSA}},
author = {Spyrou, Evaggelos and Tolias, Giorgos and Mylonas, Phivos and Avrithis, Yannis},
booktitle = {Proceedings of 9th International Workshop on Image Analysis for Multimedia Interactive Services (WIAMIS)},
month = {5},
address = {Klagenfurt, Austria},
year = {2008}
}
2007
London, UK Dec 2007
In this paper we propose the use of enhanced mid-level information, such as information obtained from the application of supervised or unsupervised learning methodologies on low-level characteristics, in order to improve semantic multimedia analysis. High-level, a priori contextual knowledge about the semantic meaning of objects and their low-level visual descriptions are combined in an integrated approach that handles in a uniform way the gap between semantics and low-level features. Prior work on low-level feature extraction is extended and a region thesaurus containing all mid-level features is constructed using a hierarchical clustering method. A model vector that contains the distances from each mid-level element is formed and a Neural Network-based detector is trained for each semantic concept. Contextual adaptation improves the quality of the produced results, by utilizing fuzzy algebra, fuzzy sets and relations. The novelty of the presented work is the context-driven mid-level manipulation of region types, utilizing a domain-independent ontology infrastructure to handle the knowledge. Early experimental results are presented using data derived from the beach domain.
@conference{C70,
title = {High-Level Concept Detection based on Mid-level Semantic Information and Contextual Adaptation},
author = {Mylonas, Phivos and Spyrou, Evaggelos and Avrithis, Yannis},
booktitle = {Proceedings of 2nd International Workshop on Semantic Media Adaptation and Personalization (SMAP)},
month = {12},
address = {London, UK},
year = {2007}
}
London, UK Dec 2007
This paper presents an approach for efficient keyframe extraction, using local semantics in form of a region thesaurus. More specifically, certain MPEG-7 color and texture features are locally extracted from keyframe regions. Then, using a hierarchical clustering approach a local region thesaurus is constructed to facilitate the description of each frame in terms of higher semantic features. The feature is consisted by the most common region types that are encountered within the video shot, along with their synonyms. These region types carry semantic information. Each keyframe is represented by a vector consisting of the degrees of confidence of the existence of all region types within this shot. Using this keyframe representation, the most representative keyframe is then selected for each shot. Where a single keyframe is not adequate, using the same algorithm and exploiting the coverage of the visual thesaurus, more keyframes are extracted.
@conference{C69,
title = {Keyframe Extraction using Local Visual Semantics in the form of a Region Thesaurus},
author = {Spyrou, Evaggelos and Avrithis, Yannis},
booktitle = {Proceedings of 2nd International Workshop on Semantic Media Adaptation and Personalization (SMAP)},
month = {12},
address = {London, UK},
year = {2007}
}
Genova, Italy Dec 2007
This paper presents an approach on high-level feature detection using a region thesaurus. MPEG-7 features are locally extracted from segmented regions and for a large set of images. A hierarchical clustering approach is applied and a relatively small number of region types is selected. This set of region types defines the region thesaurus. Using this thesaurus, low-level features are mapped to high-level concepts as model vectors. This representation is then used to train support vector machine-based feature detectors. As a next step, latent semantic analysis is applied on the model vectors, to further improve the analysis performance. High-level concepts detected derive from the natural disaster domain.
@conference{C68,
title = {A Region Thesaurus Approach for High-Level Concept Detection in the Natural Disaster Domain},
author = {Spyrou, Evaggelos and Avrithis, Yannis},
booktitle = {Proceedings of 2nd International Conference on Semantics and Digital Media Technologies (SAMT)},
month = {12},
address = {Genova, Italy},
year = {2007}
}
part of International Conference on Semantics And Digital Media Technologies
Genova, Italy Dec 2007
In this paper we focus on a contextual domain ontology representation aiding in the process of knowledge-assisted multimedia analysis. Previous work on the detection of high-level concepts within multimedia documents is extended by introducing a "mid-level" ontology as a means of exploiting the visual context of images, in terms of high-level concepts and mid-level region types they consist of. More specifically, we introduce a context ontology, define its components, its relations and integrate it in our knowledge modelling approach. In previous works we have developed algorithms to address computationally efficient handling of visual context and extraction of mid-level characteristics and now we expect these diverse algorithms and methodologies to be combined in order to exploit the proposed knowledge model. The ultimate goal remains that of efficient semantic multimedia analysis. Finally, a use case scenario derived from the beach domain is also presented, in order to demonstrate a possible application of the proposed knowledge representation.
@conference{C67,
title = {Enriching a context ontology with mid-level features for semantic multimedia analysis},
author = {Mylonas, Phivos and Spyrou, Evaggelos and Avrithis, Yannis},
booktitle = {Proceedings of 1st Workshop on Multimedia Annotation and Retrieval enabled by Shared Ontologies (MARESO), part of International Conference on Semantics And Digital Media Technologies (SAMT)},
month = {12},
address = {Genova, Italy},
year = {2007}
}
part of International Conference on Semantics And Digital Media Technologies
Genova, Italy Dec 2007
The task of multimedia document categorization forms a well-known problem in information retrieval. The task is to assign a multimedia document to one or more categories, based on its contents. In this case, effective management and thematic categorization requires the extraction of the underlying semantics. The proposed approach utilizes as input, analyzes and exploits the textual annotation that accompanies a multimedia document, in order to extract its underlying semantics, construct a semantic index and finally classify the documents to thematic categories. This process is based on a unified knowledge and semantics representation model introduced, as well as basic principles of fuzzy relational algebra. On top of that the fuzzy extension of expressive description logic language SHIN, f-SHIN and its reasoning services are used to further refine and optimize the initial categorization results. The proposed approach was tested on a set of real-life multimedia documents, derived from the Internet, as well as personal databases and shows rather promising results.
@conference{C66,
title = {Towards Semantic Multimedia Indexing by Classification and Reasoning on Textual Metadata},
author = {Mylonas, Phivos and Simou, Nikolaos and Tzouvaras, Vassilis and Avrithis, Yannis},
booktitle = {Proceedings of Knowledge Acquisition from Multimedia Content Workshop (KAMC), part of International Conference on Semantics And Digital Media Technologies (SAMT)},
month = {12},
address = {Genova, Italy},
year = {2007}
}
Crete, Greece Oct 2007
A saliency-based method for generating video summaries is presented, which exploits coupled audiovisual information from both media streams. Efficient and advanced speech and image processing algorithms to detect key frames that are acoustically and visually salient are used. Promising results are shown from experiments on a movie database.
@conference{C65,
title = {An Audio-Visual Saliency Model for Movie Summarization},
author = {Rapantzikos, Konstantinos and Evangelopoulos, Georgios and Maragos, Petros and Avrithis, Yannis},
booktitle = {Proceedings of IEEE International Workshop on Multimedia Signal Processing (MMSP)},
month = {10},
address = {Crete, Greece},
year = {2007}
}
Athens, Greece Sep 2007
In this paper previous work on the detection of high-level concepts within multimedia documents is extended by introducing a mid-level ontology as a means of exploiting the visual context of images in terms of the regions they consist of. More specifically, we construct a mid-level ontology, define its relations and integrate it in our knowledge modelling approach. In the past we have developed algorithms to address computationally efficient handling of visual context and extraction of mid-level characteristics and now we explain how these diverse algorithms and methodologies can be combined in order to approach a greater goal, that of semantic multimedia analysis. Early experimental results are presented using data derived from the beach domain.
@conference{C64,
title = {Semantic Multimedia Analysis based on Region Types and Visual Context},
author = {Spyrou, Evaggelos and Mylonas, Phivos and Avrithis, Yannis},
booktitle = {Proceedings of 4th IFIP Conference on Artificial Intelligence Applications and Innovations (AIAI)},
month = {9},
address = {Athens, Greece},
year = {2007}
}
San Antonio, TX, US Sep 2007
This paper describes salienShrink, a method to denoise images based on computing a map of salient coefficients in the wavelet domain and use it to improve common denoising algorithms. By salient, we refer to those coefficients that correspond mostly to pure signal and should therefore be preserved throughout the denoising procedure. We use a computationally efficient model to detect salient regions in the bands of the multiresolution wavelet transform. These regions are used to obtain a more accurate estimate of the noise level, improving the performance of existing well known shrinkage methods. Extensive experimental results on the BiShrink method show that the proposed method effectively enhances PSNR and improves the visual quality of the denoised images.
@conference{C63,
title = {SALIENShrink: Saliency-Based Wavelet Shrinkage},
author = {Rapantzikos, Konstantinos and Avrithis, Yannis and Kollias, Stefanos},
booktitle = {Proceedings of 14th International Conference on Image Processing (ICIP)},
volume = {3},
month = {9},
pages = {333--336},
address = {San Antonio, TX, US},
year = {2007}
}
Amsterdam, The Netherlands Jul 2007
Event detection and recognition is still one of the most active fields in computer vision, since the complexity of the dynamic events and the need for computational efficient solutions pose several difficulties. This paper addresses detection and representation of spatiotemporal salient regions using the 3D Discrete Wavelet Transform (DWT). We propose a framework to measure saliency based on the orientation selective bands of the 3D DWT and represent events using simple features of salient regions. We apply this method to human action recognition, test it on a large public video database consisting of six human actions and compare the results against an established method in the literature. Qualitative and quantitative evaluation indicates the potential of the proposed method to localize and represent human actions.
@conference{C62,
title = {Spatiotemporal saliency for event detection and representation in the 3D Wavelet Domain: Potential in human action recognition},
author = {Rapantzikos, Konstantinos and Avrithis, Yannis and Kollias, Stefanos},
booktitle = {Proceedings of ACM International Conference on Image and Video Retrieval (CIVR)},
month = {7},
pages = {294--301},
address = {Amsterdam, The Netherlands},
year = {2007}
}
Santorini, Greece Jun 2007
In this paper we propose an algorithm to improve the results of knowledge-assisted image analysis, based on contextual information. In order to achieve this, we utilize fuzzy algebra, fuzzy sets and relations, towards efficient manipulation of image region concepts. We provide a novel context modelling, based on the OWL language, using RDF reification. Initial image analysis results are enhanced by the utilization of domain-independent, semantic knowledge in terms of concepts and relations between them. The novelty of the presented work is the context-driven re-adjustment of the degrees of confidence of the detected concepts produced by any image analysis technique, utilizing a domain-independent ontology infrastructure to handle the knowledge, as well as multiple application domains.
@conference{C61,
title = {Using Multiple Domain Visual Context in Image Analysis},
author = {Mylonas, Phivos and Avrithis, Yannis},
booktitle = {Proceedings of 8th International Workshop on Image Analysis for Multimedia Interactive Services (WIAMIS)},
month = {6},
address = {Santorini, Greece},
year = {2007}
}
2006
Athens, Greece Dec 2006
In this paper, we propose the application of rule-based reasoning for knowledge assisted image segmentation and object detection. A region merging approach is proposed based on fuzzy labeling and not on visual descriptors, while reasoning is used in evaluation of dissimilarity between adjacent regions according to rules applied on local information.
@conference{C60,
title = {Rule-based Reasoning for Semantic Image Segmentation and Interpretation},
author = {Berka, Petr and Athanasiadis, Thanos and Avrithis, Yannis},
publisher = {CEUR-WS},
booktitle = {Poster \& Demo Proceedings of 1st International Conference on Semantics And digital Media Technology (SAMT)},
month = {12},
pages = {39--40},
address = {Athens, Greece},
year = {2006}
}
Athens, Greece Dec 2006
In this paper we present a framework for simultaneous image segmentation and region labeling leading to automatic image annotation. The proposed framework operates at semantic level using possible semantic labels to make decisions on handling image regions instead of visual features used traditionally. In order to stress its independence of a specific image segmentation approach we applied our idea on two region growing algorithms, i.e. watershed and recursive shortest spanning tree. Additionally we exploit the notion of visual context by employing fuzzy algebra and ontological taxonomic knowledge representation, incorporating in this way global information and improving region interpretation. In this process, semantic region growing labeling results are being re-adjusted appropriately, utilizing contextual knowledge in the form of domain-specific semantic concepts and relations. The performance of the overall methodology is demonstrated on a real-life still image dataset from the popular domains of beach holidays and motorsports.
@conference{C59,
title = {A Context-based Region Labeling Approach for Semantic Image Segmentation},
author = {Athanasiadis, Thanos and Mylonas, Phivos and Avrithis, Yannis},
booktitle = {Proceedings of 1st International Conference on Semantics And digital Media Technology (SAMT)},
month = {12},
pages = {212--225},
address = {Athens, Greece},
year = {2006}
}
Athens, Greece Dec 2006
In this poster, we present an approach to contextualized semantic image annotation as an optimization problem. Ontologies are used to capture general and contextual knowledge of the domain considered, and a genetic algorithm is applied to realize the final annotation. Experiments with images from the beach vacation domain demonstrate the performance of the proposed approach and illustrate the added value of utilizing contextual information.
@conference{C58,
title = {Using Context and a Genetic Algorithm for Knowledge-Assisted Image Analysis},
author = {Dasiopoulou, Stamatia and Papadopoulos, Georgios and Mylonas, Phivos and Avrithis, Yannis and Kompatsiaris, Ioannis},
booktitle = {Proceedings of 1st International Conference on Semantics And Digital Media Technology (SAMT)},
month = {12},
address = {Athens, Greece},
year = {2006}
}
Athens, Greece Dec 2006
This paper presents a framework for the detection of semantic features in video sequences. Low-level feature extraction is performed on the keyframes of the shots and a "feature vector" including color and texture features is formed. A region "thesaurus" that contains all the high-level features is constructed using a subtractive clustering method.Then, a "model vector" that contains the distances from each region type is formed and a SVM detector is trained for each semantic concept. Experiments were performed using TRECVID 2005 development data.
@conference{C57,
title = {Using Local Region Semantics for Concept Detection in Video},
author = {Spyrou, Evaggelos and Koumoulos, George and Avrithis, Yannis and Kollias, Stefanos},
booktitle = {Proceedings of 1st International Conference on Semantics And digital Media Technology (SAMT)},
month = {12},
address = {Athens, Greece},
year = {2006}
}
Budapest, Hungary Sep 2006
Tackling the problems of automatic object recognition and/or scene classification with generic algorithms is not producing efficient and reliable results in the field of image analysis. Restricting the problem to a specific domain is a common approach to cope with this, still unresolved, issue. In this paper we propose a methodology to improve the results of image analysis, based on available contextual information derived from the popular sports domain. Our research efforts include application of a knowledge-assisted image analysis algorithm that utilizes an ontology infrastructure to handle knowledge and MPEG-7 visual descriptors for region labeling. A novel ontological representation for context is introduced, combining fuzziness with Semantic Web characteristics, such as RDF. Initial region labeling analysis results are then being re-adjusted appropriately according to a confidence value readjustment algorithm, by means of fine-tuning the degrees of confidence of each detected region label. In this process contextual knowledge in the form of domain-specific semantic concepts and relations is utilized. Performance of the overall methodology is demonstrated through its application on a real-life still image dataset derived from the tennis sub-domain.
@conference{C56,
title = {Image Analysis Using Domain Knowledge and Visual Context},
author = {Mylonas, Phivos and Athanasiadis, Thanos and Avrithis, Yannis},
booktitle = {Proceedings of 13th International Conference on Systems, Signals and Image Processing (IWSSIP)},
month = {9},
address = {Budapest, Hungary},
year = {2006}
}
Alghero, Italy Sep 2006
In this paper we investigate the utilization of visual saliency maps for ROI-based video coding of video-telephony applications. Visually salient areas indicated in the saliency map are considered as ROIs. These areas are automatically detected using an algorithm for visual attention (VA) which builds on the bottom-up approach proposed by Itti et al. A top-down channel emulating the visual search for human faces performed by humans has been added, while orientation, intensity and color conspicuity maps are computed within a unified multi-resolution framework based on wavelet subband analysis. Priority encoding, for experimentation purposes, is utilized in a simple manner: Frame areas outside the priority regions are blurred using a smoothing filter and then passed to the video encoder. This leads to better compression of both Intra-coded (I) frames (more DCT coefficients are zeroed in the DCT-quantization step) and Inter coded (P,B) frames (lower prediction error). In more sophisticated approaches, priority encoding could be incorporated by varying the quality factor of the DCT quantization table. Extended experiments concerning both static images as well as low-quality video show the compression efficiency of the proposed method. The comparisons are made against standard JPEG and MPEG-1 encoding respectively.
@conference{C55,
title = {Priority Coding for Video-telephony Applications based on Visual Attention},
author = {Tsapatsoulis, Nicolas and Rapantzikos, Konstantinos and Avrithis, Yannis},
booktitle = {Proceedings of 2nd International Mobile Multimedia Communications Conference (MobiMedia)},
month = {9},
address = {Alghero, Italy},
year = {2006}
}
part of 17th European Conference on Artificial Intelligence
Riva del Garda, Italy Aug 2006
Combining traditional personalization techniques with novel knowledge representation paradigms, such as the ontology-based approach proposed in the Semantic Web field, is a challenging task. Personalization is a difficult problem when dealing with multimedia content and information retrieval, where context is increasingly acknowledged to be a key notion in order to make proper sense of user needs. This work focuses on contextualization within personalization in a multimedia environment. Towards that scope, we propose a novel contextual knowledge modeling scheme, and an approach for the dynamic, contextual activation of semantic user preferences to better represent user interests in coherence with ongoing user activities, e.g. in an interactive retrieval process. The application of this methodology is demonstrated using two user scenarios, and the performance results of a preliminary experiment are shown.
@conference{C54,
title = {A contextual personalization approach based on ontological knowledge},
author = {Vallet, David and Fern\'andez, Miriam and Castells, Pablo and Mylonas, Phivos and Avrithis, Yannis},
booktitle = {Proceedings of Contexts and Ontologies: Theory, Practice and Applications Workshop (CO), part of 17th European Conference on Artificial Intelligence (ECAI)},
month = {8},
address = {Riva del Garda, Italy},
year = {2006}
}
part of 21st National Conference on Artificial Intelligence
Boston, MA, US Jul 2006
Personalized content retrieval aims at improving the retrieval process by taking into account the particular interests of individual users. However, not all user preferences are relevant in all situations. It is well known that human preferences are complex, multiple, heterogeneous, changing, even contradictory, and should be understood in context with the user goals and tasks at hand. In this paper we propose a method to build a dynamic representation of the semantic context of ongoing retrieval tasks, which is used to activate different subsets of user interests at runtime, in such a way that out of context preferences are discarded. Our approach is based on an ontology-driven representation of the domain of discourse, providing enriched descriptions of the semantics involved in retrieval actions and preferences, and enabling the definition of effective means to relate preferences and context.
@conference{C53,
title = {Personalized Information Retrieval in Context},
author = {Vallet, David and Fern\'andez, Miriam and Castells, Pablo and Mylonas, Phivos and Avrithis, Yannis},
booktitle = {Proceedings of 3rd International Workshop on Modeling and Retrieval of Context (MRC), part of 21st National Conference on Artificial Intelligence (AAAI)},
month = {7},
address = {Boston, MA, US},
year = {2006}
}
part of 3rd European Semantic Web Conference
Budva, Montenegro Jun 2006
Personalization is a difficult problem related to fields and applications ranging from information retrieval to multimedia content manipulation. Challenge is greater, when trying to combine traditional personalization techniques with novel knowledge representations like ontologies. This paper proposes a novel contextual knowledge modeling, based on ontologies and fuzzy relations and exploits it in user profiling representation, extraction and use. The personalized results of the application of this methodology are then ranked accordingly. The performance of the proposed techniques is demonstrated through preliminary experimental results derived from a real-life data set.
@conference{C52,
title = {Ontology-based Personalization for Multimedia Content},
author = {Mylonas, Phivos and Vallet, David and Fern\'andez, Miriam and Castells, Pablo and Avrithis, Yannis},
booktitle = {Proceedings of Semantic Web Personalization Workshop (SWP), part of 3rd European Semantic Web Conference (ESWC)},
month = {6},
address = {Budva, Montenegro},
year = {2006}
}
Athens, Greece Jun 2006
One of the most common problems in computer vision and image processing applications is the localization of object boundaries in a video frame and its tracking in the next frames. In this paper, a fully automatic method for fast tracking of video objects in a video sequence using affine invariant normalization is proposed. Initially, the detection of a video object is achieved using a GVF snake. Next, a vector of the affine parameters of each contour of the extracted video object in two successive frames is computed using affine-invariant normalization. Under the hypothesis that these contours are similar, the affine transformation between the two contours is computed in a very fast way. Using this transformation to predict the position of the contour in the next frame allows initialization of the GVF snake very close to the real position. Applying this technique to the following frames, a very fast tracking technique is achieved. Moreover, this technique can be applied on sequences with very fast moving objects where traditional trackers usually fail. Results on synthetic sequences are presented which illustrate the theoretical developments.
@conference{C51,
title = {Fast Video Object Tracking using Affine Invariant Normalization},
author = {Tzouveli, Paraskevi and Avrithis, Yannis and Kollias, Stefanos},
booktitle = {Proceedings of 3rd IFIP Conference on Artificial Intelligence Applications \& Innovations (AIAI)},
month = {6},
address = {Athens, Greece},
year = {2006}
}
part of 15th World Wide Web Conference
Edinburgh, UK May 2006
In this position paper we examine the limitation of region growing segmentation techniques to extract semantically meaningful objects from an image. We propose a region growing algorithm that performs on a semantic level, driven by the knowledge of what each region represents at every iteration step of the merging process. This approach utilizes simultaneous segmentation and labeling of regions leading to automatic image annotation.
@conference{C50,
title = {A Semantic Region Growing Approach in Image Segmentation and Annotation},
author = {Athanasiadis, Thanos and Avrithis, Yannis and Kollias, Stefanos},
booktitle = {Proceedings of 1st International Workshop on Semantic Web Annotations for Multimedia (SWAMM), part of 15th World Wide Web Conference (WWW)},
month = {5},
address = {Edinburgh, UK},
year = {2006}
}
Seoul, Korea Apr 2006
Generic algorithms for automatic object recognition and/or scene classification are unfortunately not producing reliable and robust results. A common approach to cope with this, still unresolved, issue is to restrict the problem at hand to a specific domain. In this paper we propose an algorithm to improve the results of image analysis, based on the contextual information we have, which relates the detected concepts to any given domain. Initial results produced by the image analysis module are domain-specific semantic concepts and are being re-adjusted appropriately by the suggested algorithm, by means of fine-tuning the degrees of confidence of each detected concept. The novelty of the presented work is twofold: i) the knowledge-assisted image analysis algorithm, that utilizes an ontology infrastructure to handle the knowledge and MPEG-7 visual descriptors for the region labeling and ii) the context-driven re-adjustment of the degrees of confidence of the detected labels.
@conference{C49,
title = {Improving Image Analysis using a Contextual Approach},
author = {Mylonas, Phivos and Athanasiadis, Thanos and Avrithis, Yannis},
booktitle = {Proceedings of 7th International Workshop on Image Analysis for Multimedia Interactive Services (WIAMIS)},
month = {4},
address = {Seoul, Korea},
year = {2006}
}
2005
London, UK Nov 2005
This paper proposes a new type of a support vector machine which uses a kernel constituted from fuzzy basis functions. The proposed network combines the characteristics both of a support vector machine and a fuzzy system: high generalization performance, even when the dimension of the input space is very high, structured and numerical representation of knowledge and ability to extract linguistic fuzzy rules, in order to bridge the "semantic gap" between the low-level descriptors and the high-level semantics of an image. The Fuzzy SVM network was evaluated using images from the aceMedia Repository and more specifically in a beach/urban scenes classification problem.
@conference{C48,
title = {Fuzzy Support Vector Machines for Image Classification fusing {MPEG-7} Visual Descriptors},
author = {Spyrou, Evaggelos and Stamou, Giorgos and Avrithis, Yannis and Kollias, Stefanos},
booktitle = {Proceedings of 2nd European Workshop on the Integration of Knowledge, Semantic, and Digital Media Techniques (EWIMT)},
month = {11},
address = {London, UK},
year = {2005}
}
part of 4th International Semantic Web Conference
Galway, Ireland Nov 2005
In this paper we discuss the use of knowledge for the automatic extraction of semantic metadata from multimedia content. For the representation of knowledge we extended and enriched current general-purpose ontologies to include low-level visual features. More specifically, we implemented a tool that links MPEG-7 visual descriptors to high-level, domain-specific concepts. For the exploitation of this knowledge infrastructure we developed an experimentation platform, that allows us to analyze multimedia content and automatically create the associated semantic metadata, as well as to test, validate and refine the ontologies built. We pursued a tight and functional integration of the knowledge base and the analysis modules putting them in a loop of constant interaction instead of being the one just a pre- or post-processing step of the other.
@conference{C47,
title = {Using a Multimedia Ontology Infrastructure for Semantic Annotation of Multimedia Content},
author = {Athanasiadis, Thanos and Tzouvaras, Vassilis and Petridis, Kosmas and Precioso, Frederic and Avrithis, Yannis and Kompatsiaris, Yiannis},
publisher = {CEUR-WS},
booktitle = {Proceedings of 5th International Workshop on Knowledge Markup and Semantic Annotation, (SemAnnot), part of 4th International Semantic Web Conference (ISWC)},
month = {11},
pages = {59--68},
address = {Galway, Ireland},
year = {2005}
}
Koblenz, Germany Sep 2005
Knowledge representation and annotation of multimedia documents typically have been pursued in two different directions. Previous approaches have focused either on low level descriptors, such as dominant color, or on the content dimension and corresponding manual annotations, such as person or vehicle. In this paper, we present a knowledge infrastructure to bridge the gap between the two directions. Ontologies are being extended and enriched to include low-level audiovisual features and descriptors. Additionally, a tool for linking low-level MPEG-7 visual descriptions to ontologies and annotations has been developed. In this way, we construct ontologies that include prototypical instances of domain concepts together with a formal specification of the corresponding visual descriptors. Thus, we combine high-level domain concepts and low-level multimedia descriptions, enabling for new media content analysis.
@conference{C46,
title = {Combined Domain Specific and Multimedia Ontologies for Image Understanding},
author = {Petridis, Kosmas and Precioso, Frederic and Athanasiadis, Thanos and Avrithis, Yannis and Kompatsiaris, Yiannis},
booktitle = {Proceedings of 28th German Conference on Artificial Intelligence (KI)},
month = {9},
address = {Koblenz, Germany},
year = {2005}
}
Agia Napa, Cyprus Nov 2005
Reliability is a well-known concern in the field of personalization technologies. We propose the extension of an ontology-based retrieval system with semantic-based personalization techniques, upon which automatic mechanisms are devised that dynamically gauge the degree of personalization, so as to benefit from adaptivity but yet reduce the risk of obtrusiveness and loss of user control. On the basis of a common domain ontology KB, the personalization framework represents, captures and exploits user preferences to bias search results towards personal user interests. Upon this, the intensity of personalization is automatically increased or decreased according to an assessment of the imprecision contained in user requests and system responses before personalization is applied.
@conference{C45,
title = {Self-Tuning Personalized Information Retrieval in an Ontology-Based Framework},
author = {Castells, Pablo and Fern\'andez, Miriam and Vallet, David and Mylonas, Phivos and Avrithis, Yannis},
booktitle = {Proceedings of First IFIP WG 2.12 \& WG 12.4 International Workshop on Web Semantics (SWWS)},
month = {11},
address = {Agia Napa, Cyprus},
year = {2005}
}
Sardinia, Italy Sep 2005
It is common sense among experts that visual attention plays an important role in perception, being necessary for obtaining salient information about the surroundings. It may be the "glue" that binds simple visual features into an object [1]. Having proposed a spatiotemporal model for visual attention in the past, we elaborate on this work and use it for video classification. Our claim is that simple visual features bound to spatiotemporal salient regions will better represent the video content. Hence, we expect that feature vectors extracted from these regions will enhance the performance of the classifier. We present statistics on sports sequences of five different categories that verify our claims.
@conference{C44,
title = {On the use of spatiotemporal visual attention for video classification},
author = {Rapantzikos, Konstantinos and Avrithis, Yannis and Kollias, Stefanos},
booktitle = {Proceedings of International Workshop on Very Low Bitrate Video Coding (VLBV)},
month = {9},
address = {Sardinia, Italy},
year = {2005}
}
Sardinia, Italy Sep 2005
In this paper, an ontology infrastucture for multimedia reasoning is presented, making it possible to combine low-level visual descriptors with domain specific knowledge and subsequently analyze multimedia content with a generic algorithm that makes use of this knowledge. More specifically, the ontology infrastructure consists of a domain-specific ontology, a visual descriptor ontology (VDO) and an upper ontology. In order to interpret a scene, a set of atom regions is generated by an initial segmentation and their descriptors are extracted. Considering all descriptors in association with the related prototype instances and relations, a genetic algorithm labels the atom regions. Finally, a constraint reasoning engine enables the final region merging and labelling into meaningful objects.
@conference{C43,
title = {An Ontology Infrastructure for Multimedia Reasoning},
author = {Simou, Nikolaos and Saathoff, Carsten and Dasiopoulou, Stamatia and Spyrou, Evaggelos and Voisine, Nicolas and Tzouvaras, Vassilis and Kompatsiaris, Ioannis and Avrithis, Yannis and Staab, Steffen},
booktitle = {Proceedings of International Workshop Very Low Bitrate Video Coding (VLBV)},
month = {9},
address = {Sardinia, Italy},
year = {2005}
}
Lisbon, Portugal Oct 2005
This paper describes a comprehensive framework giving support to a wide range of personalization facilities in a multi-media content management environment. The framework builds upon a rich, ontology-based representation of the domain of discourse, whereby content semantics are linked to a rich representation of user preferences. The expressive power of ontologies is used to develop automatic learning capabilities, in order to update user profiles as users interact with the system. The resulting descriptions of user interests in terms of ontologies are exploited, along with available content metadata, to provide users with personalized content search, browsing, ranking, and retrieval. On a wider perspective, the framework is built as an open platform that provides for further user and device adaptive capability extensions.
@conference{C42,
title = {A Semantically-Enhanced Personalization Framework for Knowledge-Driven Media Services },
author = {Vallet, David and Mylonas, Phivos and Corella, Miguel A. and Fuentes, Jos\'e M. and Castells, Pablo and Avrithis, Yannis},
booktitle = {Proceedings of IADIS International Conference on WWW / Internet (ICWI)},
month = {10},
address = {Lisbon, Portugal},
year = {2005}
}
Warsaw, Poland Sep 2005
This paper proposes a number of content-based image classification techniques based on fusing various low-level MPEG-7 visual descriptors. The goal is to fuse several descriptors in order to improve the performance of several machine-learning classifiers. Fusion is necessary as descriptors would be otherwise incompatible and inappropriate to directly include e.g. in a Euclidean distance. Three approaches are described: A merging fusion combined with an SVM classifier, a back-propagation fusion combined with a K-Nearest Neighbor classifier and a Fuzzy-ART neurofuzzy network. In the latter case, fuzzy rules can be extracted in an effort to bridge the semantic gap between the low-level descriptors and the high-level semantics of an image. All networks were evaluated using content from the aceMedia Repository and more specifically in a beach/urban scenes classification problem.
@conference{C41,
title = {Fusing {MPEG-7} visual descriptors for image classification},
author = {Spyrou, Evaggelos and Le Borgne, Herv\'e and Mailis, Theofilos and Cooke, Eddie and Avrithis, Yannis and O'Connor, Noel},
booktitle = {Proceedings of International Conference on Artificial Neural Networks (ICANN)},
month = {9},
address = {Warsaw, Poland},
year = {2005}
}
Paris, France Jul 2005
Context is of great importance in a wide range of computing applications and has become a major topic in multimedia content search and retrieval systems. In this paper we focus our research efforts on visual context, a part of context suitable for multimedia analysis and usage. We introduce our efforts towards the scope of clarifying context in the fields of object detection and scene classification during multimedia analysis. We also present a method for visual context modelling, based on spatial object and region-based relations, to use in content-based multimedia search and retrieval systems.
@conference{C40,
title = {Context modelling for multimedia analysis},
author = {Mylonas, Phivos and Avrithis, Yannis},
booktitle = {Proceedings of 5th International and Interdisciplinary Conference on Modeling and Using Context (CONTEXT)},
month = {7},
address = {Paris, France},
year = {2005}
}
Riga, Latvia Jun 2005
Inspired by the human visual system, visual attention (VA) models seem to provide solutions to problems of semantic image understanding by selecting only a small but representative fraction of visual input to process. Having proposed a spatiotemporal VA model for video processing in the past, we propose considerable enhancements in this paper, including the use of steerable filters for 3D orientation estimation, and of PCA for fusion of features for the construction of saliency volumes. We further employ segmentation and feature extraction on salient regions to provide video classification using an SVM classifier. Finally, we provide results on sports video classification and comment on the usefulness of spatiotemporal VA for such purposes.
@conference{C39,
title = {An enhanced spatiotemporal visual attention model for sports video analysis},
author = {Rapantzikos, Konstantinos and Avrithis, Yannis},
booktitle = {Proceedings of 4th International Workshop on Content-Based Multimedia Indexing (CBMI)},
month = {6},
address = {Riga, Latvia},
year = {2005}
}
Heraklion, Greece May 2005
Annotations of multimedia documents typically have been pursued in two different directions. Either previous approaches have focused on low level descriptors, such as dominant color, or they have focused on the content dimension and corresponding annotations, such as person or vehicle. In this paper, we present a software environment to bridge between the two directions. M-OntoMat-Annotizer allows for linking low level MPEG-7 visual descriptions to conventional Semantic Web ontologies and annotations. We use M-OntoMat-Annotizer in order to construct ontologies that include prototypical instances of high-level domain concepts together with a formal specification of corresponding visual descriptors. Thus, we formalize the interrelationship of high- and low-level multimedia concept descriptions allowing for new kinds of multimedia content analysis and reasoning.
@conference{C38,
title = {Semantic Annotation of Images and Videos for Multimedia Analysis},
author = {Bloehdorn, Stephan and Petridis, Kosmas and Saathoff, Carsten and Simou, Nikolaos and Tzouvaras, Vassilis and Avrithis, Yannis and Handschuh, Siegfried and Kompatsiaris, Yiannis and Staab, Steffen and Strintzis, Michael G.},
booktitle = {Proceedings of 2nd European Semantic Web Conference (ESWC)},
month = {5},
address = {Heraklion, Greece},
year = {2005}
}
Montreux, Switzerland Apr 2005
In this paper we present the construction of an ontology that represents the structure of the MPEG-7 visual part. The goal of this ontology is to enable machines to generate and understand visual descriptions which can be used for multimedia reasoning. Within the specification, MPEG-7 definitions (description schemes and descriptors) are expressed in XML Schema. Although XML Schema provides the syntactic, structural, cardinality and datatyping constraints required by MPEG-7, it does not provide the semantic interoperability required to make MPEG-7 visual descriptors accessible by other domains. The knowledge representation provided by the ontology can be used to develop tools which perform knowledge-based reasoning. For the construction of the ontology we use the RDFS ontology language. We present the problems that occurred, mainly, due to the RDFS modelling limitations. Finally, we propose a way to apply reasoning using the VD ontology.
@conference{C37,
title = {A Visual Descriptor Ontology for Multimedia Reasoning},
author = {Simou, Nikolaos and Tzouvaras, Vassilis and Avrithis, Yannis and Stamou, Giorgos and Kollias, Stefanos},
booktitle = {Proceedings of 6th International Workshop on Image Analysis for Multimedia Interactive Services (WIAMIS)},
month = {4},
address = {Montreux, Switzerland},
year = {2005}
}
Montreux, Switzerland Apr 2005
Efficient video content management and exploitation requires extraction of the underlying semantics, which is a non-trivial task involving the association of low-level features with high-level concepts. In this paper, a knowledge-assisted approach for extracting semantic information of domain-specific video content is presented. Domain knowledge considers both low-level visual features (color, motion, shape) and spatial information (topological and directional relations). An initial segmentation algorithm generates a set of over-segmented atom-regions and a neural network is used to estimate the similarity distance between the extracted atom-region descriptors and the ones of the object models included in the domain ontology. A genetic algorithm is applied then in order to find the optimal interpretation according to the domain conceptualization. The proposed approach was tested on the Tennis and Formula One domains with promising results.
@conference{C36,
title = {Knowledge-Assisted Video Analysis Using A Genetic Algorithm},
author = {Voisine, Nicolas and Dasiopoulou, Stamatia and Mezaris, Vasileios and Spyrou, Evaggelos and Athanasiadis, Thanos and Kompatsiaris, Ioannis and Avrithis, Yannis and Strintzis, Michael G.},
booktitle = {Proceedings of 6th International Workshop on Image Analysis for Multimedia Interactive Services (WIAMIS)},
month = {4},
address = {Montreux, Switzerland},
year = {2005}
}
Reno, Nevada May 2005
In natural vision, we center our fixation on the most informative points in a scene in order to reduce our overall uncertainty about the scene and help interpret it. Even if we are looking for a specific stimulus around us, we face a great amount of uncertainty since that stimulus could be in any spatial location. Visual attention (VA) schemes have been proposed by researchers to account for the ability of the human eye to quickly fixate on informative regions. Recently, VA in images, and especially saliency-based VA, became an active research topic of the computer vision community. The proposed work provides an extension towards VA in video sequences by integrating spatiotemporal information. The potential applications include video classification, scene understanding, surveillance and segmentation.
@conference{C35,
title = {Handling Uncertainty in Video Analysis With Spatiotemporal Visual Attention},
author = {Rapantzikos, Konstantinos and Avrithis, Yannis and Kollias, Stefanos},
booktitle = {Proceedings of IEEE International Conference on Fuzzy Systems (FUZZ-IEEE)},
month = {5},
pages = {213--217},
address = {Reno, Nevada},
year = {2005}
}
2004
Athens, Greece Nov 2004
In this paper, an integrated information system is presented that offers enhanced search and retrieval capabilities to users of hetero-lingual digital audiovisual (a/v) archives. This innovative system exploits the advances in handling a/v content and related metadata, as introduced by MPEG-4 and worked out by MPEG-7, to offer advanced services characterized by the tri-fold "semantic phrasing of the request (query)", "unified handling" and "personalized response". The proposed system is targeting the intelligent extraction of semantic information from a/v and text related data taking into account the nature of the queries that users my issue, and the context determined by user profiles.
@conference{C34,
title = {A mediator system for hetero-lingual audiovisual content},
author = {Wallace, Manolis and Athanasiadis, Thanos and Avrithis, Yannis and Stamou, Giorgos and Kollias, Stefanos},
booktitle = {Proceedings of International Conference on Multi-platform e-Publishing (MPEP)},
month = {11},
address = {Athens, Greece},
year = {2004}
}
London, U.K. Nov 2004
Integration of knowledge and multimedia content technologies is important for the future of European industry and commerce. aceMedia is an IST FP6 project which aims to unite these two established disciplines to achieve significant advances by the combination of the two domains. This paper describes research in content processing and knowledge assisted multimedia analysis within the aceMedia project, and provides a scenario of use which illustrates the benefits of this combined approach.
@conference{C33,
title = {Achieving Integration of Knowledge and Content Technologies: the {aceMedia} Project},
author = {Hobson, Paola and May, Tony and Tromp, Jolanda and Kompatsiaris, Yiannis and Avrithis, Yannis},
booktitle = {Proceedings of European Workshop on the Integration of Knowledge, Semantics and Digital Media Technology (EWIMT)},
month = {11},
address = {London, U.K.},
year = {2004}
}
London, U.K. Nov 2004
In this paper, a knowledge representation infrastructure for semantic multimedia content analysis and reasoning is presented. This is one of the major objectives of the aceMedia Integrated Project where ontologies are being extended and enriched to include low-level audiovisual features, descriptors and behavioural models in order to support automatic content annotation. More specifically, the developed infrastructure consists of the core ontology based on extensions of the DOLCE core ontology and the multimedia-specific infrastructure components. These are, the Visual Descriptors Ontology, which is based on an RDFS representation of the MPEG-7 Visual Descriptors and the Multimedia Structure Ontology, based on the MPEG-7 MDS. Furthermore, the developed Visual Descriptor Extraction tool is presented, which will support the initialization of domain ontologies with multimedia features.
@conference{C32,
title = {Knowledge Representation for Semantic Multimedia Content Analysis and Reasoning},
author = {Petridis, Kosmas and Kompatsiaris, Ioannis and Strintzis, Michael G. and Bloehdorn, Stephan and Handschuh, Siegfried and Staab, Steffen and Simou, Nikolaos and Tzouvaras, Vassilis and Avrithis, Yannis},
booktitle = {Proceedings of European Workshop on the Integration of Knowledge, Semantics and Digital Media Technology (EWIMT)},
month = {11},
address = {London, U.K.},
year = {2004}
}
Siena, Italy Sep 2004
Several visual attention (VA) schemes have been proposed with the saliency-based ones being the most popular. The proposed work provides an extension towards VA video sequences by treating it as volumetric data. The architecture is presented in detail and potential applications are investigated. We expect that the extended VA scheme will reveal interesting events across the sequence like occlusions and short occurrences of objects, providing a basis for video surveillance (e.g. intruder detection) and summarization applications.
@conference{C31,
title = {Spatiotemporal Visual Attention Architecture for Video Analysis},
author = {Rapantzikos, Konstantinos and Tsapatsoulis, Nicolas and Avrithis, Yannis},
booktitle = {Proceedings of IEEE International Workshop On Multimedia Signal Processing (MMSP)},
month = {9},
pages = {83--86},
address = {Siena, Italy},
year = {2004}
}
Budapest, Hungary Jul 2004
In this paper we follow a fuzzy relational approach to knowledge representation. With the use of semantic fuzzy relations we define and extract the semantic context out of a set of semantic entities. Based on this, we then proceed to the case of information retrieval and explain how the three participating contexts, namely the context of the query, the context of the document and the context of the user, can be estimated and utilized towards the achievement of more intuitive information services.
@conference{C30,
title = {Fuzzy Relational Knowledge Representation and Context in the Service of Semantic Information Retrieval},
author = {Wallace, Manolis and Avrithis, Yannis},
booktitle = {Proceedings of IEEE International Conference on Fuzzy Systems (FUZZ-IEEE)},
month = {7},
address = {Budapest, Hungary},
year = {2004}
}
Dublin, Ireland Jul 2004
This paper presents FAETHON, a distributed information system that offers enhanced search and retrieval capabilities to users interacting with digital audiovisual (a/v) archives. Its novelty primarily originates in the unified intelligent access to heterogeneous a/v content. The paper emphasizes on the features that provide enhanced search and retrieval capabilities to users, as well as intelligent management of the a/v content by content creators / distributors. It describes the system's main components, the intelligent metadata creation package, the a/v search engine & portal, and the MPEG-7 compliant a/v archive interfaces. Finally, it provides ideas on the positioning of FAETHON in the market of a/v archives and video indexing and retrieval.
@conference{C29,
title = {Adding Semantics to Audiovisual Content: The {FAETHON} Project},
author = {Athanasiadis, Thanos and Avrithis, Yannis},
booktitle = {Proceedings of 3rd International Conference for Image and Video Retrieval (CIVR)},
month = {7},
pages = {665--673},
address = {Dublin, Ireland},
year = {2004}
}
Dublin, Ireland Jul 2004
In this paper we discuss the use of knowledge for the analysis and semantic retrieval of video. We follow a fuzzy relational approach to knowledge representation, based on which we define and extract the context of either a multimedia document or a user query. During indexing, the context of the document is utilized for the detection of objects and for automatic thematic categorization. During retrieval, the context of the query is used to clarify the exact meaning of the query terms and to meaningfully guide the process of query expansion and index matching. Indexing and retrieval tools have been implemented to demonstrate the proposed techniques and results are presented using video from audiovisual archives.
@conference{C28,
title = {Knowledge Assisted Analysis and Categorization for Semantic Video Retrieval},
author = {Wallace, Manolis and Athanasiadis, Thanos and Avrithis, Yannis},
booktitle = {Proceedings of 3rd International Conference for Image and Video Retrieval (CIVR)},
month = {7},
pages = {555--563},
address = {Dublin, Ireland},
year = {2004}
}
Lisboa, Portugal Apr 2004
In this paper, an approach for knowledge and context-assisted content analysis and reasoning based on a multimedia ontology infrastructure is presented. This is one of the major objectives of the aceMedia Integrated Project. In aceMedia, ontologies will be extended and enriched to include lowlevel audiovisual features, descriptors and behavioural models in order to support automatic content annotation. This approach is part of an integrated framework consisting of: user-oriented design, knowledge-driven content processing and distributed system architecture. The overall objective of aceMedia is the implementation of a novel concept for unified media representation: the Autonomous Content Entity (ACE), which has three layers: content, its associated metadata, and an intelligence layer. The ACE concept will be verified by two user focused application prototypes, enabled for both home network and mobile communication environments.
@conference{C27,
title = {Integrating Knowledge, Semantics and Content for User-Centred Intelligent Media Services: the {aceMedia} Project},
author = {Kompatsiaris, Ioannis and Avrithis, Yannis and Hobson, Paola and Strintzis, Michael G.},
booktitle = {Proceedings of Workshop on Image Analysis for Multimedia Interactive Services (WIAMIS)},
month = {4},
address = {Lisboa, Portugal},
year = {2004}
}
2003
Hamburg, Germany Sep 2003
Extraction of visual descriptor is a crucial problem for state-of-the-art visual information analysis. In this paper, we present a knowledge-based approach for detection of visual objects in video sequences. The propose approach models objects through their visual descriptors defined in MPEG7. It first extracts moving regions using an efficient active contours technique. It then computes visual descriptions of the moving regions including color features, shape features which are invariant to affine transformations, as well as motion features. The extracted features are matched to a-priori knowledge about the objects' descriptions,using appropriately defined matching functions. Results are presented which illustrate the theoretical developments
@conference{C26,
title = {Intelligent Visual Descriptor Extraction from Video Sequences},
author = {Tzouveli, Paraskevi and Andreou, Georgios and Tsechpenakis, Gabriel and Avrithis, Yannis and Kollias, Stefanos},
booktitle = {Proceedings of 1st International Workshop on Adaptive Multimedia Retrieval (AMR)},
month = {9},
address = {Hamburg, Germany},
year = {2003}
}
Rennes, France Sep 2003
Object detection techniques are coming closer to the automatic detection and identification of objects in multimedia documents. Still, this is not sufficient for the understanding of multimedia content, mainly because a simple object may be related to multiple topics, few of which are indeed related to a given document. In this paper we determine the thematic categories that are related to a document based on the objects that have been automatically detected in it. Our approach relies on stored knowledge and a fuzzy hierarchical clustering algorithm; this algorithm uses a similarity measure that is based on the notion of context. The context is extracted using fuzzy ontological relations.
@conference{C25,
title = {Using context and fuzzy relations to interpret multimedia content},
author = {Wallace, Manolis and Akrivas, Giorgos and Mylonas, Phivos and Avrithis, Yannis and Kollias, Stefanos},
booktitle = {Proceedings of 3rd International Workshop on Content-Based Multimedia Indexing (CBMI)},
month = {9},
address = {Rennes, France},
year = {2003}
}
Graz, Austria Jul 2003
In this paper, an integrated information system is presented that offers enhanced search and retrieval capabilities to users of heterogeneous digital audiovisual (a/v) archives. This innovative system exploits the advances in handlings a/v content and related metadata, as introduced by MPEG-4 and worked out by MPEG-7, to offer advanced services characterized by the tri-fold "semantic phrasing of the request (query)", "unified handling" and "personalized response". The proposed system is targeting the intelligent extraction of semantic information from a/v and text related data taking into account the nature of the queries that users my issue, and the context determined by user profiles. It also provides a personalisation process of the response in order to provide end-users with desired information. From a technical point of view, the FAETHON system plays the role of an intermediate access server residing between the end users and multiple heterogeneous audiovisual archives organized according to the new MPEG standards.
@conference{C24,
title = {Unified Access to Heterogeneous Audiovisual Archives},
author = {Avrithis, Yannis and Stamou, Giorgos and Wallace, Manolis and Marques, Ferran and Salembier, Philippe and Giro, Xavier and Haas, Werner and Vallant, Heribert and Zufferey, Michael},
booktitle = {Proceedings of 3rd International Conference on Knowledge Management (IKNOW)},
month = {7},
address = {Graz, Austria},
year = {2003}
}
London, UK Apr 2003
Multimedia Content is described via textual, semantic and structural Descriptors and Description Schemes, as introduced in MPEG-7. The semantic part of the description is closer to what the user expects from a multimedia search engine, however it poses difficulties, because of the potential incompatibility of the semantic entities among different archives. In this paper, we present FAETHON, a system that unifies the semantic description of heterogeneous archives, through the use of a semantic encyclopaedia.
@conference{C23,
title = {Semantic Unification of Heterogenous Multimedia Archives},
author = {Stamou, Giorgos and Avrithis, Yannis and Kollias, Stefanos and Marques, Ferran and Salembier, Philippe},
booktitle = {Proceedings of 4th European Workshop on Image Analysis for Multimedia Interactive Services (WIAMIS)},
month = {4},
address = {London, UK},
year = {2003}
}
2002
Thessaloniki, Greece Apr 2002
In this paper, an integrated information system is presented that offers enhanced search and retrieval capabilities to users of heterogeneous digital audiovisual (a/v) archives. This novel system exploits the advances in handling a/v content and related metadata, as introduced by MPEG-4 and worked out by MPEG-7, to offer advanced access services characterized by the tri-fold "semantic phrasing of the request (query)", "unified handling" and "personalized response". The proposed system is targeting the intelligent extraction of semantic information from a/v and text related data taking into account the nature of useful queries that users may issue, and the context determined by user profiles. From a technical point of view, it will play the role of an intermediate access server residing between the end users and multiple heterogeneous audiovisual archives organized according to new MPEG standards.
@conference{C22,
title = {Intelligent Semantic Access to Audiovisual Content},
author = {Avrithis, Yannis and Stamou, Giorgos and Delopoulos, Anastasios and Kollias, Stefanos},
booktitle = {Proceedings of 2nd Hellenic Conference on Artificial Intelligence (SETN)},
month = {4},
address = {Thessaloniki, Greece},
year = {2002}
}
2001
Tenerife, Spain Dec 2001
A system for digitization, storage and retrieval of audiovisual information and its associated data (meta-info) is presented. The principles of the evolving MPEG-7 standard have been adopted for the creation of the data model used by the system, permitting efficient separation of database design, content description, business logic and presentation of query results. XML Schema is used in defining the data model, and XML in describing audiovisual content. Issues regarding problems that emerged during system design and their solutions are discussed, such as customization, deviations from the standard MPEG-7 DSs or even the design of entirely custom DSs. Although the system includes modules for digitization, annotation, archiving and intelligent data mining, the paper mainly focuses on the use of MPEG-7 as the information model.
@conference{C21,
title = {An Intelligent System for Retrieval and Mining of Audiovisual Material Based on the {MPEG-7} Description Schemes},
author = {Akrivas, Giorgos and Ioannou, Spyros and Karakoulakis, Elias and Karpouzis, Kostas and Avrithis, Yannis and Delopoulos, Anastasios and Kollias, Stefanos and Varlamis, Iraklis and Vaziriannis, Michalis},
booktitle = {Proceedings of European Symposium on Intelligent Technologies, Hybrid Systems and their implementation on Smart Adaptive Systems (EUNITE)},
month = {12},
address = {Tenerife, Spain},
year = {2001}
}
Athens, Greece Oct 2001
In this paper, the FAETHON project is presented, whose overall objective is to develop an integrated information system that offers enhanced search and retrieval capabilities to users of heterogeneous digital audiovisual (a/v) archives. This novel system will exploit the advances in handling a/v content and related metadata, as introduced by MPEG-4 and worked out by MPEG-7, to offer advanced access services characterized by the tri-fold "semantic phrasing of the request (query)", "unified handling" and "personalized response".
@conference{C20,
title = {{FAETHON}: Unified Intelligent Access to Heterogeneous Audiovisual Content},
author = {Avrithis, Yannis and Stamou, Giorgos},
booktitle = {Proceedings of International Workshop on Very Low Bitrate Video Coding (VLBV)},
month = {10},
address = {Athens, Greece},
year = {2001}
}
Brescia, Italy Sep 2001
Content-based audiovisual data retrieval utilizing new emerging related standards such as MPEG-7 will yield ineffective results, unless major focus is given to the semantic information level. Mapping of low level, sub-symbolic descriptors of a/v archives to high level symbolic ones is in general difficult, even impossible with the current state of technology. It can, however, be tackled when dealing with specific application domains. It seems that the extraction of semantic information from a/v and text related data is tractable taking into account the nature of useful queries that users may issue and the context determined by user profile. The IST project FAETHON is developing a novel platform to implement these ideas for user friendly and highly informative access to distributed audiovisual archives.
@conference{C19,
title = {Unified Intelligent Access to Heterogeneous Audiovisual Content},
author = {Delopoulos, Anastasios and Kollias, Stefanos and Avrithis, Yannis and Haas, Werner and Majcen, Kurt},
booktitle = {Proceedings of 2nd International Workshop in Content-Based Multimedia Indexing (CBMI)},
month = {9},
address = {Brescia, Italy},
year = {2001}
}
2000
Barcelona, Spain Sep 2000
A novel method for two-dimensional curve normalization with respect to affine transformations is presented in this paper, allowing an affine-invariant curve representation to be obtained without any actual loss of information on the original curve. It can be applied as a pre-processing step to any shape representation, classification, recognition or retrieval technique, since it effectively decouples the problem of affine-invariant description from feature extraction and pattern matching. Curves estimated from object contours are first modeled by cubic B-splines and then normalized in several steps in order to eliminate translation, scaling, skew, starting point, rotation and reflection transformations, based on a combination of curve features including moments and Fourier descriptors.
@conference{C18,
title = {Affine-Invariant Curve Normalization for Shape-Based Retrieval},
author = {Avrithis, Yannis and Xirouhakis, Yiannis and Kollias, Stefanos},
booktitle = {Proceedings of 15th International Conference on Pattern Recognition (ICPR)},
month = {9},
pages = {1015--1018},
address = {Barcelona, Spain},
year = {2000}
}
Vancouver, BC, Canada Sep 2000
Face detection is becoming an important tool in the framework of many multimedia applications. Several face detection algorithms based on skin color characteristics have recently appeared in the literature. Most of them face generalization problems due to the skin color model they use. In this work we present a study which attempts to minimize the generalization problem by combining the M-RSST color segmentation algorithm with a Gaussian model of the skin color distribution and global shape features. Moreover by associating the resultant segments with a face probability we can index and retrieve facial images from multimedia databases.
@conference{C17,
title = {Efficient Face Detection for Multimedia Applications},
author = {Tsapatsoulis, Nicolas and Avrithis, Yannis and Kollias, Stefanos},
booktitle = {Proceedings of the International Conference on Image Processing (ICIP)},
month = {9},
address = {Vancouver, BC, Canada},
year = {2000}
}
Tampere, Finland Sep 2000
Content-based retrieval from image databases attracts increasing interest the last few years. On the other hand several recent works on face detection based on the chrominance components of the color space have been presented in the literature showing promising results. In this work we combine color segmentation techniques and color based face detection in an efficient way for the purpose of facial image retrieving. In particular, images stored in a multimedia database are analyzed using the M-RSST segmentation algorithm and segment features including average color components, size, location, shape and texture are extracted for several image resolutions. An adaptive two-dimensional Gaussian density function is then employed for modeling skin-tone chrominance color component distribution and detecting image segments that probably correspond to human faces. This information is combined with object shape characteristics so that robust face detection is achieved. Based on the above, a query by example framework is proposed, supporting a highly interactive, configurable and flexible content-based retrieval system for human faces. Experimental results have shown that the proposed implementation combines efficiency, robustness and speed, and could be extended to generic visual information retrieval or video databases.
@conference{C16,
title = {Color-Based Retrieval of Facial Images},
author = {Avrithis, Yannis and Tsapatsoulis, Nicolas and Kollias, Stefanos},
booktitle = {Proceedings of 10th European Signal Processing Conference (EUSIPCO)},
month = {9},
pages = {1397--1400},
address = {Tampere, Finland},
year = {2000}
}
New York City, NY, US Jul 2000
Automatic content-based analysis and indexing of broadcast news recordings or digitized news archives is becoming an important tool in the framework of many multimedia interactive services such as news summarization, browsing, retrieval and news-on-demand (NoD) applications. Existing approaches have achieved high performance in such applications but heavily rely on textual cues such as closed caption tokens and teletext transcripts. In this work we present an efficient technique for temporal segmentation and parsing of news recordings based on visual cues that can either be employed as stand-alone application for non-closed captioned broadcasts or integrated with audio and textual cues of existing systems. The technique involves robust face detection by means of color segmentation, skin color matching and shape processing, and is able to identify typical news instances like anchorpersons, reports and outdoor shots.
@conference{C15,
title = {Broadcast News Parsing Using Visual Cues: A Robust Face Detection Approach},
author = {Avrithis, Yannis and Tsapatsoulis, Nicolas and Kollias, Stefanos},
booktitle = {Proceedings of IEEE International Conference on Multimedia and Expo (ICME)},
month = {7},
pages = {1469--1472},
address = {New York City, NY, US},
year = {2000}
}
1999
Copenhagen, Denmark Sep 1999
A video content representation framework is proposed in this paper for extracting limited but meaningful information of video data directly from MPEG compressed domain. A hierarchical color and motion segmentation scheme is applied to each video shot, transforming the conventional frame-based representation to a feature-based one. Then, all features are gathered together using a fuzzy formulation and extraction of several key frames is performed for each shot in a content-based rate sampling framework. In particular, our approach is based on minimization of a cross-correlation criterion among video frames of a given shot, so as to be located a set of minimally correlated feature vectors.
@conference{C14,
title = {A stochastic framework for optimal key frame extraction from {MPEG} video databases},
author = {Doulamis, Nikolaos and Doulamis, Anastasios and Avrithis, Yannis and Kollias, Stefanos},
booktitle = {Proceedings of IEEE International Workshop on Multimedia Signal Processing (MMSP)},
month = {9},
pages = {141--146},
address = {Copenhagen, Denmark},
year = {1999}
}
Santorini, Greece Sep 1999
In the context of this paper a generalized framework for non-linear representation of 3-D video sequences is proposed, regardless of the scene complexity. In particular, depth information is exploited to provide a more reliable video content segmentation. In this paper this is accomplished by merging color segments which belong to similar depth since a video object is usually located on the same depth plane while color segments give very accurate contours of the objects. To accelerate the segmentation algorithm a multiresolution implementation of the Recursive Shortest Spanning Tree (RSST) algorithm is presented both for color and depth segmentation. All features extracted by the video sequence analysis module are gathered together using a fuzzy feature vector formulation to increase the robustness of the proposed summarization scheme. Finally, key frames within each shot are extracted by minimizing a cross correlation criterion by means of a genetic algorithm.
@conference{C13,
title = {An Optimal Framework for Summarization of Stereoscopic Video Sequences},
author = {Doulamis, Nikolaos and Doulamis, Anastasios and Avrithis, Yannis and Ntalianis, Klimis and Kollias, Stefanos},
booktitle = {Proceedings of International Workshop on Synthetic-Natural Hybrid Coding and Three Dimensional Imaging (IWSNHC3DI)},
month = {9},
address = {Santorini, Greece},
year = {1999}
}
Orlando, FL, US Aug 1999
A facial expression recognition scheme is presented in this paper, based on features derived from the optical flow between two instances of a face in the same emotional state. A pre-processing step of isolating the human face from the background is first employed by means of face detection and registration. A spatio-temporal description of the expression is then obtained by evaluating the Radon transform of the motion vectors between the face in its neutral condition and at the 'apex' of the expression. A linear curve normalization scheme is proposed, achieving a translation, scaling and resolution invariant representation of the Radon curves. Finally, experimental results are presented, illustrating the performance of the proposed algorithm for expression classification using a correlation criterion and a neural network classifier.
@conference{C12,
title = {On the use of Radon Transform for Facial Expression Recognition},
author = {Tsapatsoulis, Nicolas and Avrithis, Yannis and Kollias, Stefanos},
booktitle = {Proceedings of 5th International Conference on Information Systems Analysis and Synthesis (ISAS)},
month = {8},
address = {Orlando, FL, US},
year = {1999}
}
Athens, Greece Jul 1999
Recent literature comprises a large number of papers on the query and retrieval of visual information based on its content. At the same time, a number of prototype systems have been implemented enabling searching through on-line image databases and still image retrieval. However, it has been often pointed out that meaningful/semantic information should be extracted from visual information in order to improve the efficiency and functionality of a content-based retrieval tool. In this context, present work focuses on the extraction of objects from images and video clips and modeling of the resulting object contours using B-splines. Affine-invariant curve representation is obtained through Normalized Fourier descriptors (NFD), curve moments, as well as a novel curve normalization algorithm that leads to major preservation of object shape information. A neural network approach is employed for supervised classification of video objects into prototype object classes. Experiments on several real-life and simulated video sequences are included to evaluate the classification results for all affine-invariant representations used.
@conference{C11,
title = {Affine Invariant Representation and Classification of Object Contours for Image and Video Retrieval},
author = {Avrithis, Yannis and Xirouhakis, Yiannis and Kollias, Stefanos},
booktitle = {Proceedings of 3rd IEEE/IMACS World Multiconference on Circuits, Systems, Communications and Computers (CSCC)},
month = {7},
address = {Athens, Greece},
year = {1999}
}
Florence, Italy Jun 1999
This paper presents an integrated framework for interactive content-based retrieval in video databases by means of visual queries. The proposed system incorporates algorithms for video shot detection, key frame and shot selection, automated video object segmentation and tracking, and construction of multidimensional feature vectors using fuzzy classification of color, motion or texture segment properties. Retrieval is then performed in an interactive way by employing a parametric distance between feature vectors and updating distance parameters according to user requirements using relevance feedback. Experimental results demonstrate increased performance and flexibility according to user information needs.
@conference{C10,
title = {Interactive Content-Based Retrieval in Video Databases Using Fuzzy Classification and Relevance Feedback},
author = {Doulamis, Anastasios and Avrithis, Yannis and Doulamis, Nikolaos and Kollias, Stefanos},
booktitle = {Proceedings of IEEE International Conference on Multimedia Computing and Systems (ICMSC)},
month = {6},
pages = {954--958},
address = {Florence, Italy},
year = {1999}
}
1998
London, UK Oct 1998
In this paper, a system for content-based image retrieval from video databases is introduced, using B-splines for affine invariant object representation. A small number of key-frames is extracted from each video sequence, which provide sufficient information about the video content. Color and motion segmentation and tracking is then employed for automatic extraction of video objects. A B-spline representation of the object contours is then obtained, which possesses important properties, such as smoothness, continuity and invariance under affine transformation. A neural network approach is used for supervised classification of video objects into prototype object classes. Finally, higher level classes can be constructed combining primary classes, providing the ability to obtain a high level of abstraction in the representation of each video sequence.
@conference{C9,
title = {Image Retrieval and Classification Using Affine Invariant B-Spline Representation and Neural Networks},
author = {Xirouhakis, Yiannis and Avrithis, Yannis and Kollias, Stefanos},
booktitle = {Proceedings of IEE Colloquium on Neural Nets and Multimedia (ICNNM)},
month = {10},
pages = {4/1--4/4},
address = {London, UK},
year = {1998}
}
Urbana, IL, US Oct 1998
An integrated framework for content-based indexing and retrieval in video databases is presented in this paper, which has the capability of adapting its performance according to user requirements. Video sequences are represented by extracting a small number of key frames or scenes and constructing multidimensional feature vectors using fuzzy classification of color, motion or texture segment properties. Queries are then performed by employing a parametric distance between feature vectors, and adaptation is achieved by estimating distance parameters according to user requirements, resulting in a content based retrieval system of increased performance and flexibility.
@conference{C8,
title = {An Adaptive Approach to Video Indexing and Retrieval Using Fuzzy Classification},
author = {Avrithis, Yannis and Doulamis, Anastasios and Doulamis, Nikolaos and Kollias, Stefanos},
booktitle = {Proceedings of International Conference on Very Low Bitrate Video Coding (VLBV)},
month = {10},
address = {Urbana, IL, US},
year = {1998}
}
Chicago, IL, US Oct 1998
In this paper, an efficient video content representation is proposed using optimal extraction of characteristic frames and scenes. This representation, apart from providing browsing capabilities to digital video databases, also allows more efficient content-based queries and indexing. For performing the frame/scene extraction, a feature vector formulation of the images is proposed based on color and motion segmentation. Then, the scene selection is accomplished by clustering similar scenes based on a distortion criterion. Frame selection is performed using an optimization method for locating a set of minimally correlated feature vectors.
@conference{C7,
title = {Video Content Representation Using Optimal Extraction of Frames and Scenes},
author = {Doulamis, Nikolaos and Doulamis, Anastasios and Avrithis, Yannis and Kollias, Stefanos},
booktitle = {Proceedings of IEEE International Conference on Image Processing (ICIP)},
month = {10},
pages = {875--879},
address = {Chicago, IL, US},
year = {1998}
}
Rhodes, Greece Sep 1998
A new method for designing ultrasonic imaging systems is presented in this paper. The method is based on the use of transducer arrays whose elements transmit wideband signals generated by pseudo-random codes, similarly to code division multiple access (CDMA) systems in communications. The use of code sequences instead of pulses, which are typically used in conventional phased arrays, combined with transmit and receive beamforming for steering different codes at each direction, permits parallel acquisition of a large number of measurements corresponding to different directions. Significantly higher image acquisition rate as well as lateral and contrast resolution are thus obtained, while axial resolution remains close to that of phased arrays operating in pulse-echo mode. Time and frequency division techniques are also studied and a unified theoretical model is derived, which is validated by experimental results.
@conference{C6,
title = {Ultrasonic Array Imaging Using {CDMA} Techniques},
author = {Avrithis, Yannis and Delopoulos, Anastasios and Papageorgiou, Grigorios},
booktitle = {Proceedings of IX European Signal Processing Conference (EUSIPCO)},
month = {9},
address = {Rhodes, Greece},
year = {1998}
}
Athens, Greece Jun 1998
The first stage of the proposed algorithm includes a scene cut detection mechanism. Then, video processing and image analysis techniques are applied to each video frame for extracting color, motion and texture information. Color information is extracted by applying a hierarchical color segmentation algorithm to each video frame. Consequently, apart from the color histogram of each frame additional features are collected concerning the number of color segments, and their location, size and shape. Motion information is also extracted in a similar way by using a motion estimation and segmentation algorithm. All the above features are gathered in order to form a multidimensional feature vector for each video frame. The representation of each frame by a feature vector, apart from reducing storage requirements, transforms the image domain to another domain, more efficient for key frame selection. Since similar frames can be characterized by different color or motion segments, due to imperfections of the segmentation algorithms, a fuzzy representation of feature vectors is adopted in order to provide more robust searching capabilities. In particular, we classify color as well as motion and texture segments into pre-determined classes forming a multidimensional histogram and a degree of membership is allocated to each category so that the possibility of erroneous comparisons is eliminated.
@conference{C5,
title = {A Genetic Algorithm for Efficient Video Content Representation},
author = {Doulamis, Anastasios and Avrithis, Yannis and Doulamis, Nikolaos and Kollias, Stefanos},
booktitle = {Proceedings of IMACS/IFAC International Symposium on Soft Computing in Engineering Applications (SOFTCOM)},
month = {6},
address = {Athens, Greece},
year = {1998}
}
part of IEEE Conference on Computer Vision and Pattern Recognition
Santa Barbara, CA, US Jun 1998
In this paper, an efficient video content representation system is presented which permits automatic extraction of a limited number of characteristic frames or scenes that provide sufficient information about the content of an MPEG video sequence. This can be used for reduction of the amount of stored information that is necessary in order to provide search capabilities in a multimedia database, resulting in faster and more efficient video queries. Moreover, the proposed system can be used for automatic generation of low resolution video clip previews (trailers), giving the ability to browse databases on web pages. Finally, direct content-based retrieval with image queries is possible using the feature vector representation incorporated in our system.
@conference{C4,
title = {Efficient Content Representation in {MPEG} Video Databases},
author = {Avrithis, Yannis and Doulamis, Nikolaos and Doulamis, Anastasios and Kollias, Stefanos},
booktitle = {Proceedings of IEEE Workshop on Content-Based Access of Image and Video Libraries (CBAIVL), part of IEEE Conference on Computer Vision and Pattern Recognition (CVPR)},
month = {6},
pages = {91--95},
address = {Santa Barbara, CA, US},
year = {1998}
}
1997
Santorini, Greece Jul 1997
Recent progress in supervised image classification research, has demonstrated the potential usefulness of incorporating fuzziness in the training, allocation and testing stages of several classification techniques. In this paper a multiresolution neural network approach to supervised classification is presented, exploiting the inherent fuzziness of such techniques in order to perform classification at different resolution levels and gain in computational complexity. In particular, multiresolution image analysis is carried out and hierarchical neural networks are used as an efficient architecture for classification of the derived multiresolution image representations. A new scheme is then introduced for transferring classification results to higher resolutions based on the fuzziness of the results of lower resolutions, resulting in faster implementation. Experimental results on land cover mapping applications from remotely sensed data illustrate significant improvements in classification speed without deterioration of representation accuracy.
@conference{C3,
title = {Fuzzy Image Classification Using Multiresolution Neural Networks with Applications to Remote Sensing},
author = {Avrithis, Yannis and Kollias, Stefanos},
booktitle = {Proceedings of 13th International Conference on Digital Signal Processing (DSP)},
month = {7},
pages = {261--264},
address = {Santorini, Greece},
year = {1997}
}
Louvain-la-Neuve, Belgium Jun 1997
An integrated framework for automatic extraction of the most characteristic frames or scenes of a video sequence is presented in this paper. This is accomplished by extracting a collection of a small number of frames or scenes that provide sufficient information about the video sequence. The scene/frame selection mechanism is based on a transformation from the image to a feature domain, which is more suitable for image comparisons, queries and retrieval.
@conference{C2,
title = {Indexing and Retrieval of the Most Characteristic Frames / Scenes in Video Databases},
author = {Doulamis, Anastasios and Avrithis, Yannis and Doulamis, Nikolaos and Kollias, Stefanos},
booktitle = {Proceedings of Workshop on Image Analysis for Multimedia Interactive Services (WIAMIS)},
month = {6},
pages = {105--110},
address = {Louvain-la-Neuve, Belgium},
year = {1997}
}
1993
Nicosia, Cyprus Jul 1993
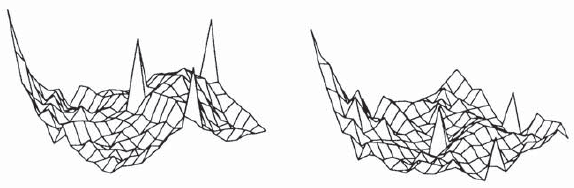
The implementation of an efficient scheme for translation, rotation and scale invariant optical character recognition is presented in this paper. An image representation is used, which is based on appropriate clustering and transformation of the image triple-correlation domain. This representation is one-to-one related to the class of all shifted-rotated-scaled versions of the original image, as well as robust to a wide variety of additive noises. Special attention is given to binary images, which are used for Optical Character Recognition, and simulation results illustrate the performance of the proposed implementation.
@conference{C1,
title = {An Efficient Scheme for Invariant Optical Character Recognition Using Triple Correlations},
author = {Avrithis, Yannis and Delopoulos, Anastasios and Kollias, Stefanos},
booktitle = {Proceedings of International Conference on Digital Signal Processing (DSP)},
month = {7},
address = {Nicosia, Cyprus},
year = {1993}
}
Preprints / technical reports
2024
This work addresses composed image retrieval in the context of domain conversion, where the content of a query image is retrieved in the domain specified by the query text. We show that a strong vision-language model provides sufficient descriptive power without additional training. The query image is mapped to the text input space using textual inversion. Unlike common practice that invert in the continuous space of text tokens, we use the discrete word space via a nearest-neighbor search in a text vocabulary. With this inversion, the image is softly mapped across the vocabulary and is made more robust using retrieval-based augmentation. Database images are retrieved by a weighted ensemble of text queries combining mapped words with the domain text. Our method outperforms prior art by a large margin on standard and newly introduced benchmarks. Code: this https URL
@article{R53,
title = {Composed Image Retrieval for Training-Free Domain Conversion},
author = {Efthymiadis, Nikos and Psomas, Bill and Laskar, Zakaria and Karantzalos, Konstantinos and Avrithis, Yannis and Chum, Ondrej and Tolias, Giorgos},
journal = {arXiv preprint arXiv:2412.03297},
month = {12},
year = {2024}
}
This work introduces composed image retrieval to remote sensing. It allows to query a large image archive by image examples alternated by a textual description, enriching the descriptive power over unimodal queries, either visual or textual. Various attributes can be modified by the textual part, such as shape, color, or context. A novel method fusing image-to-image and text-to-image similarity is introduced. We demonstrate that a vision-language model possesses sufficient descriptive power and no further learning step or training data are necessary. We present a new evaluation benchmark focused on color, context, density, existence, quantity, and shape modifications. Our work not only sets the state-of-the-art for this task, but also serves as a foundational step in addressing a gap in the field of remote sensing image retrieval. Code at: this https URL.
@article{R52,
title = {Composed Image Retrieval for Remote Sensing},
author = {Psomas, Bill and Kakogeorgiou, Ioannis and Efthymiadis, Nikos and Tolias, Giorgos and Chum, Ondrej and Avrithis, Yannis and Karantzalos, Konstantinos},
journal = {arXiv preprint arXiv:2405.15587},
month = {5},
year = {2024}
}
Multi-target unsupervised domain adaptation (UDA) aims to learn a unified model to address the domain shift between multiple target domains. Due to the difficulty of obtaining annotations for dense predictions, it has recently been introduced into cross-domain semantic segmentation. However, most existing solutions require labeled data from the source domain and unlabeled data from multiple target domains concurrently during training. Collectively, we refer to this data as "external". When faced with new unlabeled data from an unseen target domain, these solutions either do not generalize well or require retraining from scratch on all data. To address these challenges, we introduce a new strategy called "multi-target UDA without external data" for semantic segmentation. Specifically, the segmentation model is initially trained on the external data. Then, it is adapted to a new unseen target domain without accessing any external data. This approach is thus more scalable than existing solutions and remains applicable when external data is inaccessible. We demonstrate this strategy using a simple method that incorporates self-distillation and adversarial learning, where knowledge acquired from the external data is preserved during adaptation through "one-way" adversarial learning. Extensive experiments in several synthetic-to-real and real-to-real adaptation settings on four benchmark urban driving datasets show that our method significantly outperforms current state-of-the-art solutions, even in the absence of external data. Our source code is available online this https URL.
@article{R51,
title = {Multi-Target Unsupervised Domain Adaptation for Semantic Segmentation without External Data},
author = {Xu, Yonghao and Ghamisi, Pedram and Avrithis, Yannis},
journal = {arXiv preprint arXiv:2405.06502},
month = {5},
year = {2024}
}
This paper studies interpretability of convolutional networks by means of saliency maps. Most approaches based on Class Activation Maps (CAM) combine information from fully connected layers and gradient through variants of backpropagation. However, it is well understood that gradients are noisy and alternatives like guided backpropagation have been proposed to obtain better visualization at inference. In this work, we present a novel training approach to improve the quality of gradients for interpretability. In particular, we introduce a regularization loss such that the gradient with respect to the input image obtained by standard backpropagation is similar to the gradient obtained by guided backpropagation. We find that the resulting gradient is qualitatively less noisy and improves quantitatively the interpretability properties of different networks, using several interpretability methods.
@article{R50,
title = {A Learning Paradigm for Interpretable Gradients},
author = {Torres Figueroa, Felipe and Zhang, Hanwei and Sicre, Ronan and Avrithis, Yannis and Ayache, Stephane},
journal = {arXiv preprint arXiv:2404.15024},
month = {4},
year = {2024}
}
Explanations obtained from transformer-based architectures in the form of raw attention, can be seen as a class-agnostic saliency map. Additionally, attention-based pooling serves as a form of masking the in feature space. Motivated by this observation, we design an attention-based pooling mechanism intended to replace Global Average Pooling (GAP) at inference. This mechanism, called Cross-Attention Stream (CA-Stream), comprises a stream of cross attention blocks interacting with features at different network depths. CA-Stream enhances interpretability in models, while preserving recognition performance.
@article{R49,
title = {{CA}-Stream: Attention-based pooling for interpretable image recognition},
author = {Torres Figueroa, Felipe and Zhang, Hanwei and Sicre, Ronan and Ayache, Stephane and Avrithis, Yannis},
journal = {arXiv preprint arXiv:2404.14996},
month = {4},
year = {2024}
}
How important is it for training and evaluation sets to not have class overlap in image retrieval? We revisit Google Landmarks v2 clean, the most popular training set, by identifying and removing class overlap with Revisited Oxford and Paris [34], the most popular evaluation set. By comparing the original and the new RGLDv2-clean on a benchmark of reproduced state-of-the-art methods, our findings are striking. Not only is there a dramatic drop in performance, but it is inconsistent across methods, changing the ranking.What does it take to focus on objects or interest and ignore background clutter when indexing? Do we need to train an object detector and the representation separately? Do we need location supervision? We introduce Single-stage Detect-to-Retrieve (CiDeR), an end-to-end, single-stage pipeline to detect objects of interest and extract a global image representation. We outperform previous state-of-the-art on both existing training sets and the new RGLDv2-clean. Our dataset is available at this https URL.
@article{R48,
title = {On Train-Test Class Overlap and Detection for Image Retrieval},
author = {Song, Chull Hwan and Yoon, Jooyoung and Hwang, Taebaek and Choi, Shunghyun and Gu, Yeong Hyeon and Avrithis, Yannis},
journal = {arXiv preprint arXiv:2404.01524},
month = {4},
year = {2024}
}
2023
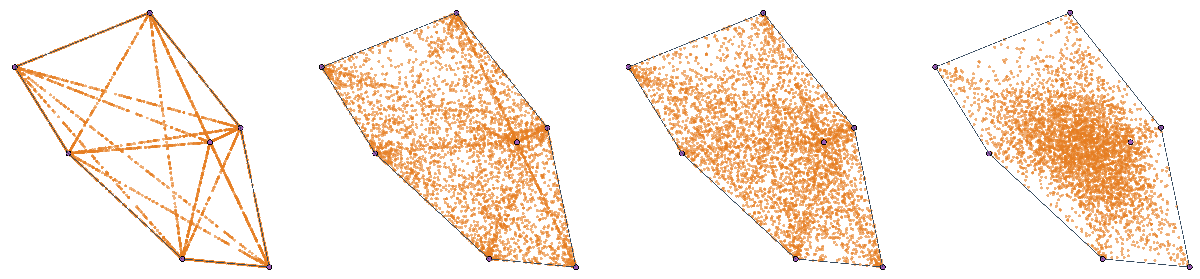
Multimodal sentiment analysis (MSA) leverages heterogeneous data sources to interpret the complex nature of human sentiments. Despite significant progress in multimodal architecture design, the field lacks comprehensive regularization methods. This paper introduces PowMix, a versatile embedding space regularizer that builds upon the strengths of unimodal mixing-based regularization approaches and introduces novel algorithmic components that are specifically tailored to multimodal tasks. PowMix is integrated before the fusion stage of multimodal architectures and facilitates intra-modal mixing, such as mixing text with text, to act as a regularizer. PowMix consists of five components: 1) a varying number of generated mixed examples, 2) mixing factor reweighting, 3) anisotropic mixing, 4) dynamic mixing, and 5) cross-modal label mixing. Extensive experimentation across benchmark MSA datasets and a broad spectrum of diverse architectural designs demonstrate the efficacy of PowMix, as evidenced by consistent performance improvements over baselines and existing mixing methods. An in-depth ablation study highlights the critical contribution of each PowMix component and how they synergistically enhance performance. Furthermore, algorithmic analysis demonstrates how PowMix behaves in different scenarios, particularly comparing early versus late fusion architectures. Notably, PowMix enhances overall performance without sacrificing model robustness or magnifying text dominance. It also retains its strong performance in situations of limited data. Our findings position PowMix as a promising versatile regularization strategy for MSA. Code will be made available.
@article{R47,
title = {{PowMix}: A Versatile Regularizer for Multimodal Sentiment Analysis},
author = {Georgiou, Efthymios and Avrithis, Yannis and Potamianos, Alexandros},
journal = {arXiv preprint arXiv:2312.12334},
month = {12},
year = {2023}
}
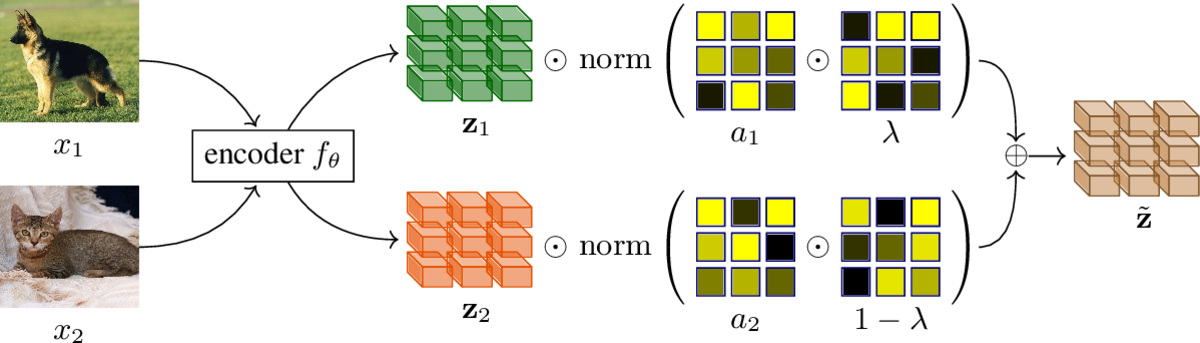
Mixup refers to interpolation-based data augmentation, originally motivated as a way to go beyond empirical risk minimization (ERM). Its extensions mostly focus on the definition of interpolation and the space (input or feature) where it takes place, while the augmentation process itself is less studied. In most methods, the number of generated examples is limited to the mini-batch size and the number of examples being interpolated is limited to two (pairs), in the input space.
We make progress in this direction by introducing MultiMix, which generates an arbitrarily large number of interpolated examples beyond the mini-batch size and interpolates the entire mini-batch in the embedding space. Effectively, we sample on the entire convex hull of the mini-batch rather than along linear segments between pairs of examples.
On sequence data, we further extend to Dense MultiMix. We densely interpolate features and target labels at each spatial location and also apply the loss densely. To mitigate the lack of dense labels, we inherit labels from examples and weight interpolation factors by attention as a measure of confidence.
Overall, we increase the number of loss terms per mini-batch by orders of magnitude at little additional cost. This is only possible because of interpolating in the embedding space. We empirically show that our solutions yield significant improvement over state-of-the-art mixup methods on four different benchmarks, despite interpolation being only linear. By analyzing the embedding space, we show that the classes are more tightly clustered and uniformly spread over the embedding space, thereby explaining the improved behavior.
@article{R46,
title = {Embedding Space Interpolation Beyond Mini-Batch, Beyond Pairs and Beyond Examples},
author = {Venkataramanan, Shashanka and Kijak, Ewa and Amsaleg, Laurent and Avrithis, Yannis},
journal = {arXiv preprint arXiv:2311.05538},
month = {11},
year = {2023}
}
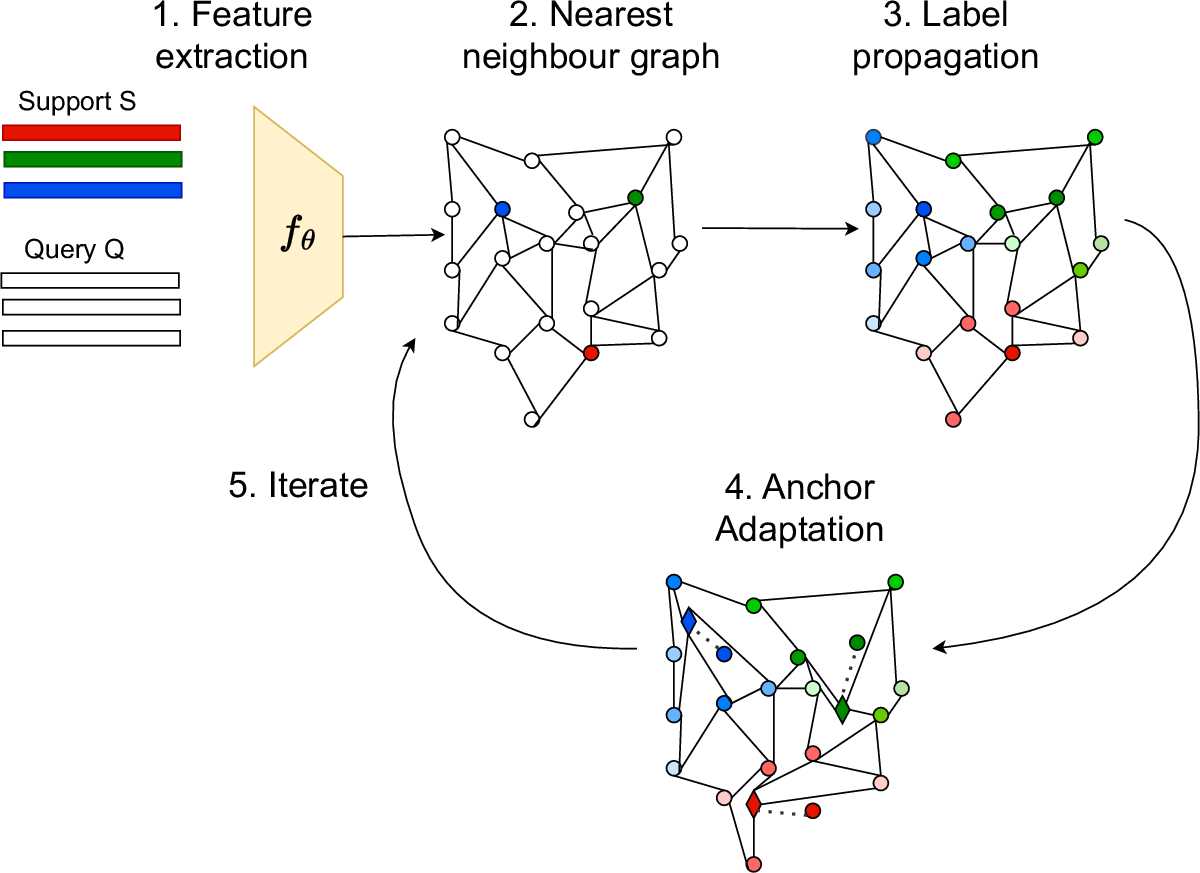
Few-shot learning addresses the issue of classifying images using limited labeled data. Exploiting unlabeled data through the use of transductive inference methods such as label propagation has been shown to improve the performance of few-shot learning significantly. Label propagation infers pseudo-labels for unlabeled data by utilizing a constructed graph that exploits the underlying manifold structure of the data. However, a limitation of the existing label propagation approaches is that the positions of all data points are fixed and might be sub-optimal so that the algorithm is not as effective as possible. In this work, we propose a novel algorithm that adapts the feature embeddings of the labeled data by minimizing a differentiable loss function optimizing their positions in the manifold in the process. Our novel algorithm, Adaptive Anchor Label Propagation}, outperforms the standard label propagation algorithm by as much as 7% and 2% in the 1-shot and 5-shot settings respectively. We provide experimental results highlighting the merits of our algorithm on four widely used few-shot benchmark datasets, namely miniImageNet, tieredImageNet, CUB and CIFAR-FS and two commonly used backbones, ResNet12 and WideResNet-28-10. The source code can be found at this https URL.
@article{R45,
title = {Adaptive Anchors Label Propagation for Transductive Few-Shot Learning},
author = {Lazarou, Michalis and Avrithis, Yannis and Ren, Guangyu and Stathaki, Tania},
journal = {arXiv preprint arXiv:2310.19996},
month = {10},
year = {2023}
}
Self-supervised learning has unlocked the potential of scaling up pretraining to billions of images, since annotation is unnecessary. But are we making the best use of data? How more economical can we be? In this work, we attempt to answer this question by making two contributions. First, we investigate first-person videos and introduce a "Walking Tours" dataset. These videos are high-resolution, hours-long, captured in a single uninterrupted take, depicting a large number of objects and actions with natural scene transitions. They are unlabeled and uncurated, thus realistic for self-supervision and comparable with human learning.
Second, we introduce a novel self-supervised image pretraining method tailored for learning from continuous videos. Existing methods typically adapt image-based pretraining approaches to incorporate more frames. Instead, we advocate a "tracking to learn to recognize" approach. Our method called DoRA, leads to attention maps that Discover and tRAck objects over time in an end-to-end manner, using transformer cross-attention. We derive multiple views from the tracks and use them in a classical self-supervised distillation loss. Using our novel approach, a single Walking Tours video remarkably becomes a strong competitor to ImageNet for several image and video downstream tasks.
@article{R44,
title = {Is ImageNet worth 1 video? Learning strong image encoders from 1 long unlabelled video},
author = {Venkataramanan, Shashanka and Rizve, Mamshad Nayeem and Carreira, Jo\~ao and Asano, Yuki M. and Avrithis, Yannis},
journal = {arXiv preprint arXiv:2310.08584},
month = {10},
year = {2023}
}
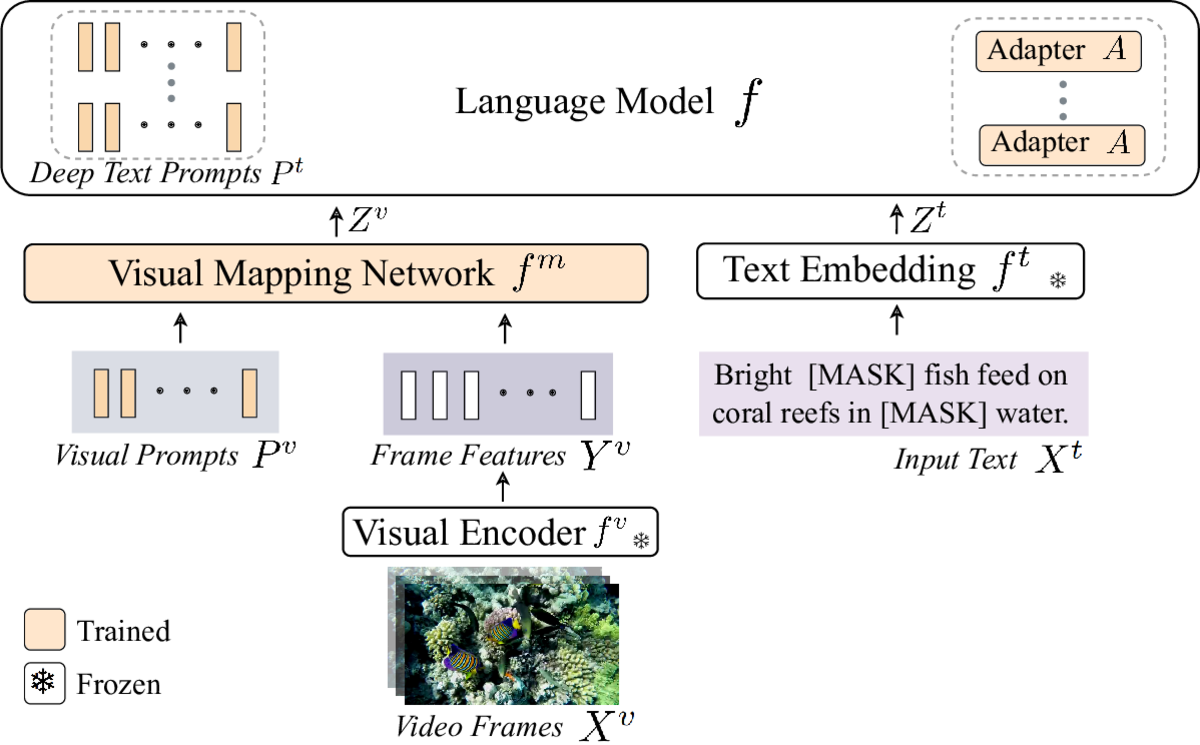
Recent vision-language models are driven by large-scale pretrained models. However, adapting pretrained models on limited data presents challenges such as overfitting, catastrophic forgetting, and the cross-modal gap between vision and language. We introduce a parameter-efficient method to address these challenges, combining multimodal prompt learning and a transformer-based mapping network, while keeping the pretrained models frozen. Our experiments on several video question answering benchmarks demonstrate the superiority of our approach in terms of performance and parameter efficiency on both zero-shot and few-shot settings. Our code is available at this https URL.
@article{R43,
title = {Zero-Shot and Few-Shot Video Question Answering with Multi-Modal Prompts},
author = {Engin, Deniz and Avrithis, Yannis},
journal = {arXiv preprint arXiv:2309.15915},
month = {9},
year = {2023}
}
Convolutional networks and vision transformers have different forms of pairwise interactions, pooling across layers and pooling at the end of the network. Does the latter really need to be different? As a by-product of pooling, vision transformers provide spatial attention for free, but this is most often of low quality unless self-supervised, which is not well studied. Is supervision really the problem?
In this work, we develop a generic pooling framework and then we formulate a number of existing methods as instantiations. By discussing the properties of each group of methods, we derive SimPool, a simple attention-based pooling mechanism as a replacement of the default one for both convolutional and transformer encoders. We find that, whether supervised or self-supervised, this improves performance on pre-training and downstream tasks and provides attention maps delineating object boundaries in all cases. One could thus call SimPool universal. To our knowledge, we are the first to obtain attention maps in supervised transformers of at least as good quality as self-supervised, without explicit losses or modifying the architecture. Code at: this https URL.
@article{R42,
title = {Keep It {SimPool}: Who Said Supervised Transformers Suffer from Attention Deficit?},
author = {Psomas, Bill and Kakogeorgiou, Ioannis and Karantzalos, Konstantinos and Avrithis, Yannis},
journal = {arXiv preprint arXiv:2309.06891},
month = {9},
year = {2023}
}
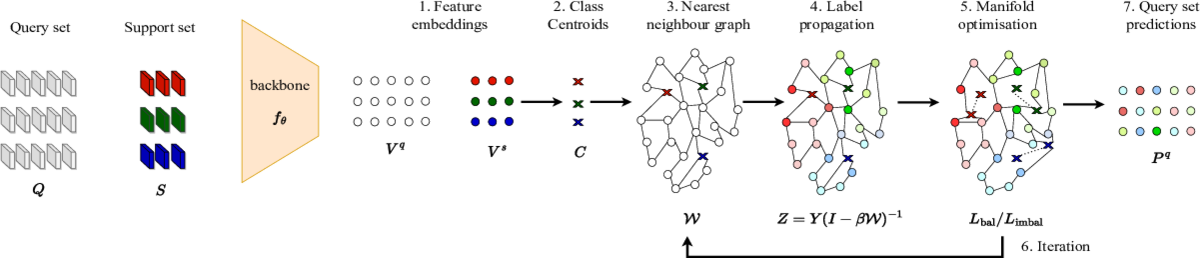
Transductive few-shot learning algorithms have showed substantially superior performance over their inductive counterparts by leveraging the unlabeled queries. However, the vast majority of such methods are evaluated on perfectly class-balanced benchmarks. It has been shown that they undergo remarkable drop in performance under a more realistic, imbalanced setting. To this end, we propose a novel algorithm to address imbalanced transductive few-shot learning, named Adaptive Manifold. Our method exploits the underlying manifold of the labeled support examples and unlabeled queries by using manifold similarity to predict the class probability distribution per query. It is parameterized by one centroid per class as well as a set of graph-specific parameters that determine the manifold. All parameters are optimized through a loss function that can be tuned towards class-balanced or imbalanced distributions. The manifold similarity shows substantial improvement over Euclidean distance, especially in the 1-shot setting. Our algorithm outperforms or is on par with other state of the art methods in three benchmark datasets, namely miniImageNet, tieredImageNet and CUB, and three different backbones, namely ResNet-18, WideResNet-28-10 and DenseNet-121. In certain cases, our algorithm outperforms the previous state of the art by as much as 4.2%.
@article{R41,
title = {Adaptive manifold for imbalanced transductive few-shot learning},
author = {Lazarou, Michalis and Avrithis, Yannis and Stathaki, Tania},
journal = {arXiv preprint arXiv:2304.14281},
month = {4},
year = {2023}
}
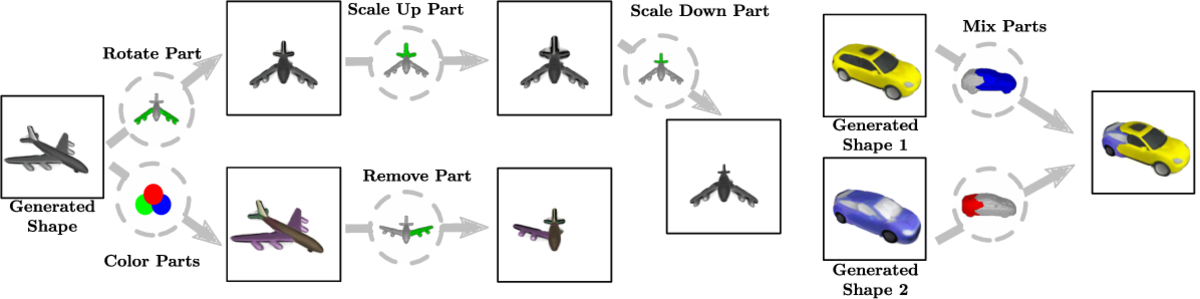
Impressive progress in generative models and implicit representations gave rise to methods that can generate 3D shapes of high quality. However, being able to locally control and edit shapes is another essential property that can unlock several content creation applications. Local control can be achieved with part-aware models, but existing methods require 3D supervision and cannot produce textures. In this work, we devise PartNeRF, a novel part-aware generative model for editable 3D shape synthesis that does not require any explicit 3D supervision. Our model generates objects as a set of locally defined NeRFs, augmented with an affine transformation. This enables several editing operations such as applying transformations on parts, mixing parts from different objects etc. To ensure distinct, manipulable parts we enforce a hard assignment of rays to parts that makes sure that the color of each ray is only determined by a single NeRF. As a result, altering one part does not affect the appearance of the others. Evaluations on various ShapeNet categories demonstrate the ability of our model to generate editable 3D objects of improved fidelity, compared to previous part-based generative approaches that require 3D supervision or models relying on NeRFs.
@article{R40,
title = {{PartNeRF}: Generating Part-Aware Editable 3D Shapes without 3D Supervision},
author = {Tertikas, Konstantinos and Paschalidou, Despoina and Pan, Boxiao and Park, Jeong Joon and Uy, Mikaela Angelina and Emiris, Ioannis and Avrithis, Yannis and Guibas, Leonidas},
journal = {arXiv preprint arXiv:2303.09554},
month = {3},
year = {2023}
}
Methods based on class activation maps (CAM) provide a simple mechanism to interpret predictions of convolutional neural networks by using linear combinations of feature maps as saliency maps. By contrast, masking-based methods optimize a saliency map directly in the image space or learn it by training another network on additional data.
In this work we introduce Opti-CAM, combining ideas from CAM-based and masking-based approaches. Our saliency map is a linear combination of feature maps, where weights are optimized per image such that the logit of the masked image for a given class is maximized. We also fix a fundamental flaw in two of the most common evaluation metrics of attribution methods. On several datasets, Opti-CAM largely outperforms other CAM-based approaches according to the most relevant classification metrics. We provide empirical evidence supporting that localization and classifier interpretability are not necessarily aligned.
@article{R39,
title = {Opti-{CAM}: Optimizing saliency maps for interpretability},
author = {Zhang, Hanwei and Torres, Felipe and Sicre, Ronan and Avrithis, Yannis and Ayache, Stephane},
journal = {arXiv preprint arXiv:2301.07002},
month = {1},
year = {2023}
}
2022
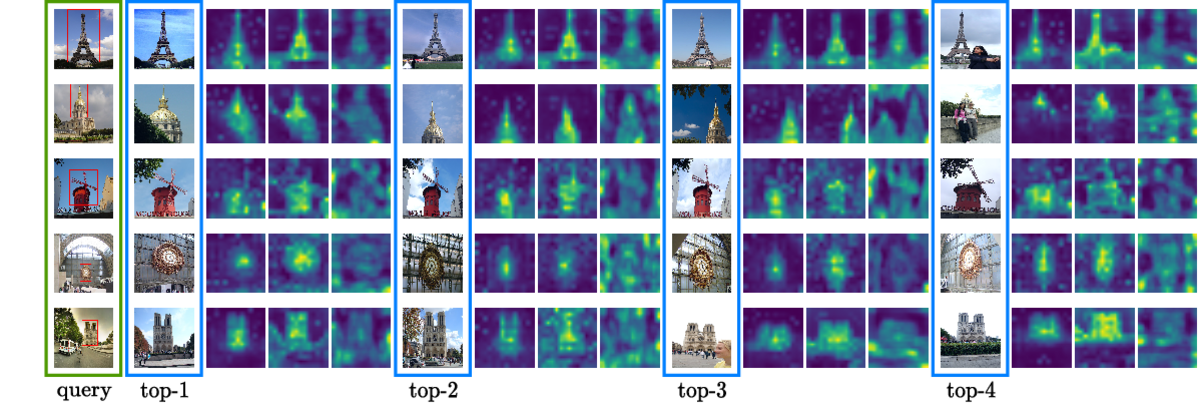
Vision transformers have achieved remarkable progress in vision tasks such as image classification and detection. However, in instance-level image retrieval, transformers have not yet shown good performance compared to convolutional networks. We propose a number of improvements that make transformers outperform the state of the art for the first time. (1) We show that a hybrid architecture is more effective than plain transformers, by a large margin. (2) We introduce two branches collecting global (classification token) and local (patch tokens) information, from which we form a global image representation. (3) In each branch, we collect multi-layer features from the transformer encoder, corresponding to skip connections across distant layers. (4) We enhance locality of interactions at the deeper layers of the encoder, which is the relative weakness of vision transformers. We train our model on all commonly used training sets and, for the first time, we make fair comparisons separately per training set. In all cases, we outperform previous models based on global representation. Public code is available at this https URL.
@article{R38,
title = {Boosting vision transformers for image retrieval},
author = {Song, Chull Hwan and Yoon, Jooyoung and Choi, Shunghyun and Avrithis, Yannis},
journal = {arXiv preprint arXiv:2210.11909},
month = {10},
year = {2022}
}
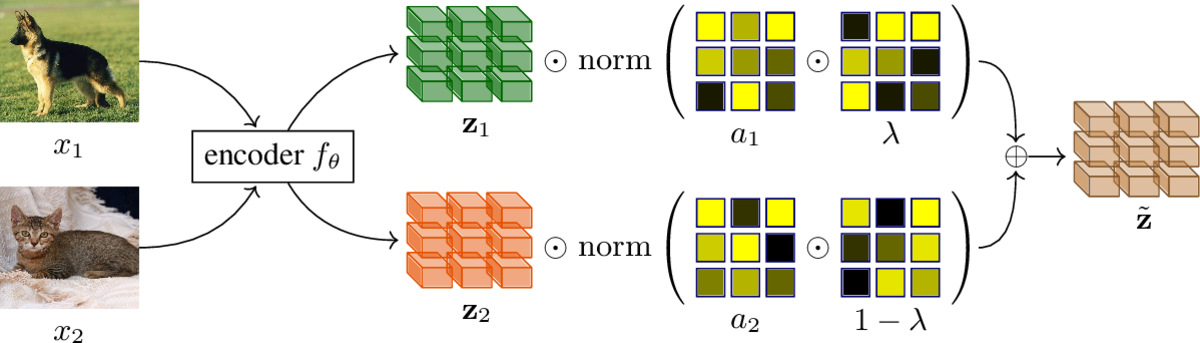
Mixup refers to interpolation-based data augmentation, originally motivated as a way to go beyond empirical risk minimization (ERM). Yet, its extensions focus on the definition of interpolation and the space where it takes place, while the augmentation itself is less studied: For a mini-batch of size $m$, most methods interpolate between $m$ pairs with a single scalar interpolation factor $\lambda$.
In this work, we make progress in this direction by introducing MultiMix, which interpolates an arbitrary number $n$ of tuples, each of length $m$, with one vector $\lambda$ per tuple. On sequence data, we further extend to dense interpolation and loss computation over all spatial positions. Overall, we increase the number of tuples per mini-batch by orders of magnitude at little additional cost. This is possible by interpolating at the very last layer before the classifier. Finally, to address inconsistencies due to linear target interpolation, we introduce a self-distillation approach to generate and interpolate synthetic targets.
We empirically show that our contributions result in significant improvement over state-of-the-art mixup methods on four benchmarks. By analyzing the embedding space, we observe that the classes are more tightly clustered and uniformly spread over the embedding space, thereby explaining the improved behavior.
@article{R37,
title = {Teach me how to Interpolate a Myriad of Embeddings},
author = {Venkataramanan, Shashanka and Kijak, Ewa and Amsaleg, Laurent and Avrithis, Yannis},
journal = {arXiv preprint arXiv:2206.14868},
month = {6},
year = {2022}
}
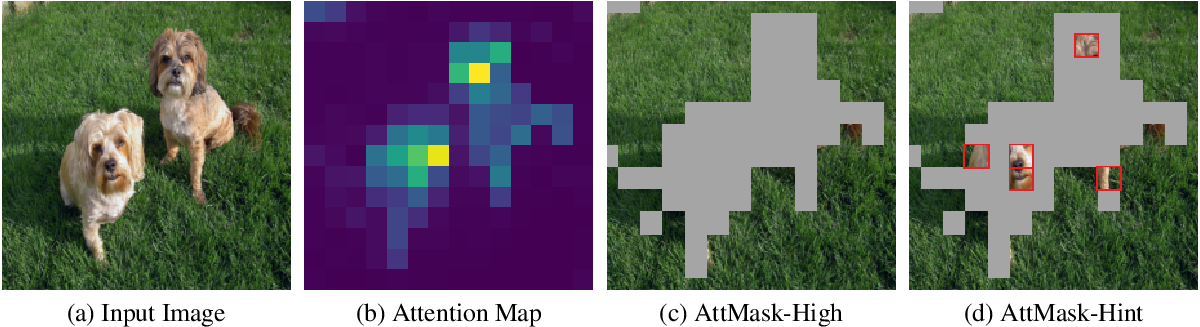
Transformers and masked language modeling are quickly being adopted and explored in computer vision as vision transformers and masked image modeling (MIM). In this work, we argue that image token masking differs from token masking in text, due to the amount and correlation of tokens in an image. In particular, to generate a challenging pretext task for MIM, we advocate a shift from random masking to informed masking. We develop and exhibit this idea in the context of distillation-based MIM, where a teacher transformer encoder generates an attention map, which we use to guide masking for the student. We thus introduce a novel masking strategy, called attention-guided masking (AttMask), and we demonstrate its effectiveness over random masking for dense distillation-based MIM as well as plain distillation-based self-supervised learning on classification tokens. We confirm that AttMask accelerates the learning process and improves the performance on a variety of downstream tasks. We provide the implementation code at this https URL.
@article{R36,
title = {What to Hide from Your Students: Attention-Guided Masked Image Modeling},
author = {Kakogeorgiou, Ioannis and Gidaris, Spyros and Psomas, Bill and Avrithis, Yannis and Bursuc, Andrei and Karantzalos, Konstantinos and Komodakis, Nikos},
journal = {arXiv preprint arXiv:2203.12719},
month = {7},
year = {2022}
}
2021
We address representation learning for large-scale instance-level image retrieval. Apart from backbone, training pipelines and loss functions, popular approaches have focused on different spatial pooling and attention mechanisms, which are at the core of learning a powerful global image representation. There are different forms of attention according to the interaction of elements of the feature tensor (local and global) and the dimensions where it is applied (spatial and channel). Unfortunately, each study addresses only one or two forms of attention and applies it to different problems like classification, detection or retrieval.
We present global-local attention module (GLAM), which is attached at the end of a backbone network and incorporates all four forms of attention: local and global, spatial and channel. We obtain a new feature tensor and, by spatial pooling, we learn a powerful embedding for image retrieval. Focusing on global descriptors, we provide empirical evidence of the interaction of all forms of attention and improve the state of the art on standard benchmarks.
@article{R35,
title = {All the attention you need: Global-local, spatial-channel attention for image retrieval},
author = {Song, Chull Hwan and Han, Hye Joo and Avrithis, Yannis},
journal = {arXiv preprint arXiv:2107.08000},
month = {7},
year = {2021}
}
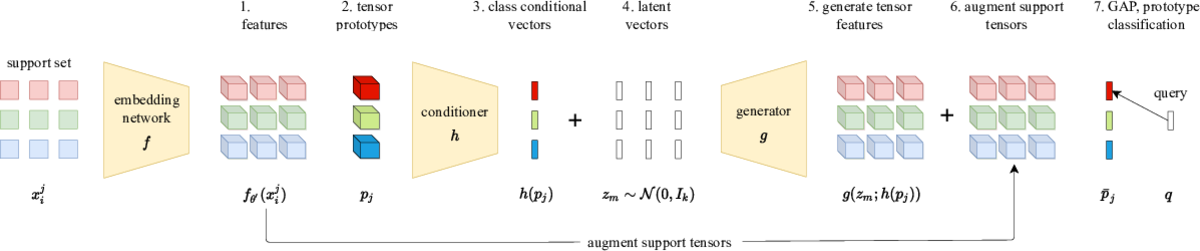
Few-shot classification addresses the challenge of classifying examples given not just limited supervision but limited data as well. An attractive solution is synthetic data generation. However, most such methods are overly sophisticated, focusing on high-quality, realistic data in the input space. It is unclear whether adapting them to the few-shot regime and using them for the downstream task of classification is the right approach. Previous works on synthetic data generation for few-shot classification focus on exploiting complex models, e.g. a Wasserstein GAN with multiple regularizers or a network that transfers latent diversities from known to novel classes.
We follow a different approach and investigate how a simple and straightforward synthetic data generation method can be used effectively. We make two contributions, namely we show that: (1) using a simple loss function is more than enough for training a feature generator in the few-shot setting; and (2) learning to generate tensor features instead of vector features is superior. Extensive experiments on miniImagenet, CUB and CIFAR-FS datasets show that our method sets a new state of the art, outperforming more sophisticated few-shot data augmentation methods.
@article{R34,
title = {Tensor feature hallucination for few-shot learning},
author = {Lazarou, Michalis and Stathaki, Tania and Avrithis, Yannis},
journal = {arXiv preprint arXiv:2106.05321},
month = {6},
year = {2021}
}
Metric learning involves learning a discriminative representation such that embeddings of similar classes are encouraged to be close, while embeddings of dissimilar classes are pushed far apart. State-of-the-art methods focus mostly on sophisticated loss functions or mining strategies. On the one hand, metric learning losses consider two or more examples at a time. On the other hand, modern data augmentation methods for classification consider two or more examples at a time. The combination of the two ideas is under-studied.
In this work, we aim to bridge this gap and improve representations using mixup, which is a powerful data augmentation approach interpolating two or more examples and corresponding target labels at a time. This task is challenging because, unlike classification, the loss functions used in metric learning are not additive over examples, so the idea of interpolating target labels is not straightforward. To the best of our knowledge, we are the first to investigate mixing examples and target labels for deep metric learning. We develop a generalized formulation that encompasses existing metric learning loss functions and modify it to accommodate for mixup, introducing Metric Mix, or Metrix. We show that mixing inputs, intermediate representations or embeddings along with target labels significantly improves representations and outperforms state-of-the-art metric learning methods on four benchmark datasets.
@article{R33,
title = {It Takes Two to Tango: Mixup for Deep Metric Learning},
author = {Venkataramanan, Shashanka and Psomas, Bill and Avrithis, Yannis and Kijak, Ewa and Amsaleg, Laurent and Karantzalos, Konstantinos},
journal = {arXiv preprint arXiv:2106.04990},
month = {6},
year = {2021}
}
Few-shot classification addresses the challenge of classifying examples given only limited labeled data. A powerful approach is to go beyond data augmentation, towards data synthesis. However, most of data augmentation/synthesis methods for few-shot classification are overly complex and sophisticated, e.g. training a wGAN with multiple regularizers or training a network to transfer latent diversities from known to novel classes. We make two contributions, namely we show that: (1) using a simple loss function is more than enough for training a feature generator in the few-shot setting; and (2) learning to generate tensor features instead of vector features is superior. Extensive experiments on miniImagenet, CUB and CIFAR-FS datasets show that our method sets a new state of the art, outperforming more sophisticated few-shot data augmentation methods.
@article{R32,
title = {Few-shot learning via tensor hallucination},
author = {Lazarou, Michalis and Avrithis, Yannis and Stathaki, Tania},
journal = {arXiv preprint arXiv:2104.09467},
month = {4},
year = {2021}
}
Mixup is a powerful data augmentation method that interpolates between two or more examples in the input or feature space and between the corresponding target labels. Many recent mixup methods focus on cutting and pasting two or more objects into one image, which is more about efficient processing than interpolation. However, how to best interpolate images is not well defined. In this sense, mixup has been connected to autoencoders, because often autoencoders "interpolate well", for instance generating an image that continuously deforms into another.
In this work, we revisit mixup from the interpolation perspective and introduce AlignMix, where we geometrically align two images in the feature space. The correspondences allow us to interpolate between two sets of features, while keeping the locations of one set. Interestingly, this gives rise to a situation where mixup retains mostly the geometry or pose of one image and the texture of the other, connecting it to style transfer. More than that, we show that an autoencoder can still improve representation learning under mixup, without the classifier ever seeing decoded images. AlignMix outperforms state-of-the-art mixup methods on five different benchmarks.
@article{R31,
title = {{AlignMixup}: Improving representations by interpolating aligned features},
author = {Venkataramanan, Shashanka and Avrithis, Yannis and Kijak, Ewa and Amsaleg, Laurent},
journal = {arXiv preprint arXiv:2103.15375},
month = {3},
year = {2021}
}
High-level understanding of stories in video such as movies and TV shows from raw data is extremely challenging. Modern video question answering (VideoQA) systems often use additional human-made sources like plot synopses, scripts, video descriptions or knowledge bases. In this work, we present a new approach to understand the whole story without such external sources. The secret lies in the dialog: unlike any prior work, we treat dialog as a noisy source to be converted into text description via dialog summarization, much like recent methods treat video. The input of each modality is encoded by transformers independently, and a simple fusion method combines all modalities, using soft temporal attention for localization over long inputs. Our model outperforms the state of the art on the KnowIT VQA dataset by a large margin, without using question-specific human annotation or human-made plot summaries. It even outperforms human evaluators who have never watched any whole episode before.
@article{R30,
title = {On the hidden treasure of dialog in video question answering},
author = {Engin, Deniz and Duong, Ngoc Q. K. and Schnitzler, Fran\c{c}ois and Avrithis, Yannis},
journal = {arXiv preprint arXiv:2103.14517},
month = {3},
year = {2021}
}
The challenge in few-shot learning is that available data is not enough to capture the underlying distribution. To mitigate this, two emerging directions are (a) using local image representations, essentially multiplying the amount of data by a constant factor, and (b) using more unlabeled data, for instance by transductive inference, jointly on a number of queries. In this work, we bring these two ideas together, introducing local propagation. We treat local image features as independent examples, we build a graph on them and we use it to propagate both the features themselves and the labels, known and unknown. Interestingly, since there is a number of features per image, even a single query gives rise to transductive inference. As a result, we provide a universally safe choice for few-shot inference under both non-transductive and transductive settings, improving accuracy over corresponding methods. This is in contrast to existing solutions, where one needs to choose the method depending on the quantity of available data.
@article{R29,
title = {Local propagation for few-shot learning},
author = {Lifchitz, Yann and Avrithis, Yannis and Picard, Sylvaine},
journal = {arXiv preprint arXiv:2101.01480},
month = {1},
year = {2021}
}
2020
Few-shot learning amounts to learning representations and acquiring knowledge such that novel tasks may be solved with both supervision and data being limited. Improved performance is possible by transductive inference, where the entire test set is available concurrently, and semi-supervised learning, where more unlabeled data is available. These problems are closely related because there is little or no adaptation of the representation in novel tasks.
Focusing on these two settings, we introduce a new algorithm that leverages the manifold structure of the labeled and unlabeled data distribution to predict pseudo-labels, while balancing over classes and using the loss value distribution of a limited-capacity classifier to select the cleanest labels, iterately improving the quality of pseudo-labels. Our solution sets new state of the art on four benchmark datasets, namely miniImageNet, tieredImageNet, CUB and CIFAR-FS, while being robust over feature space pre-processing and the quantity of available data.
@article{R28,
title = {Iterative label cleaning for transductive and semi-supervised few-shot learning},
author = {Lazarou, Michalis and Avrithis, Yannis and Stathaki, Tania},
journal = {arXiv preprint arXiv:2012.07962},
month = {12},
year = {2020}
}
Knowledge transfer from large teacher models to smaller student models has recently been studied for metric learning, focusing on fine-grained classification. In this work, focusing on instance-level image retrieval, we study an asymmetric testing task, where the database is represented by the teacher and queries by the student. Inspired by this task, we introduce asymmetric metric learning, a novel paradigm of using asymmetric representations at training. This acts as a simple combination of knowledge transfer with the original metric learning task.
We systematically evaluate different teacher and student models, metric learning and knowledge transfer loss functions on the new asymmetric testing as well as the standard symmetric testing task, where database and queries are represented by the same model. We find that plain regression is surprisingly effective compared to more complex knowledge transfer mechanisms, working best in asymmetric testing. Interestingly, our asymmetric metric learning approach works best in symmetric testing, allowing the student to even outperform the teacher.
@article{R27,
title = {Asymmetric metric learning for knowledge transfer},
author = {Budnik, Mateusz and Avrithis, Yannis},
journal = {arXiv preprint arXiv:2006.16331},
month = {6},
year = {2020}
}
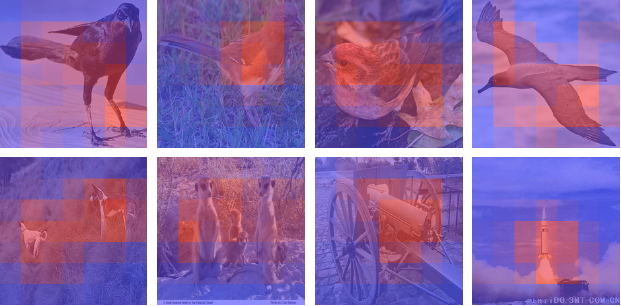
Few-shot learning is often motivated by the ability of humans to learn new tasks from few examples. However, standard few-shot classification benchmarks assume that the representation is learned on a limited amount of base class data, ignoring the amount of prior knowledge that a human may have accumulated before learning new tasks. At the same time, even if a powerful representation is available, it may happen in some domain that base class data are limited or non-existent. This motivates us to study a problem where the representation is obtained from a classifier pre-trained on a large-scale dataset of a different domain, assuming no access to its training process, while the base class data are limited to few examples per class and their role is to adapt the representation to the domain at hand rather than learn from scratch. We adapt the representation in two stages, namely on the few base class data if available and on the even fewer data of new tasks. In doing so, we obtain from the pre-trained classifier a spatial attention map that allows focusing on objects and suppressing background clutter. This is important in the new problem, because when base class data are few, the network cannot learn where to focus implicitly. We also show that a pre-trained network may be easily adapted to novel classes, without meta-learning.
@article{R26,
title = {Few-Shot Few-Shot Learning and the role of Spatial Attention},
author = {Lifchitz, Yann and Avrithis, Yannis and Picard, Sylvaine},
journal = {arXiv preprint arXiv:2002.07522},
month = {2},
year = {2020}
}
2019

Adversarial examples of deep neural networks are receiving ever increasing attention because they help in understanding and reducing the sensitivity to their input. This is natural given the increasing applications of deep neural networks in our everyday lives. When white-box attacks are almost always successful, it is typically only the distortion of the perturbations that matters in their evaluation.
In this work, we argue that speed is important as well, especially when considering that fast attacks are required by adversarial training. Given more time, iterative methods can always find better solutions. We investigate this speed-distortion trade-off in some depth and introduce a new attack called boundary projection (BP) that improves upon existing methods by a large margin. Our key idea is that the classification boundary is a manifold in the image space: we therefore quickly reach the boundary and then optimize distortion on this manifold.
@article{R25,
title = {Walking on the Edge: Fast, Low-Distortion Adversarial Examples},
author = {Zhang, Hanwei and Avrithis, Yannis and Furon, Teddy and Amsaleg, Laurent},
journal = {arXiv preprint arXiv:1912.02153},
month = {12},
year = {2019}
}
Weakly-supervised object detection attempts to limit the amount of supervision by dispensing the need for bounding boxes, but still assumes image-level labels on the entire training set are available. In this work, we study the problem of training an object detector from one or few clean images with image-level labels and a larger set of completely unlabeled images. This is an extreme case of semi-supervised learning where the labeled data are not enough to bootstrap the learning of a classifier or detector. Our solution is to use a standard weakly-supervised pipeline to train a student model from image-level pseudo-labels generated on the unlabeled set by a teacher model, bootstrapped by region-level similarities to clean labeled images. By using the recent pipeline of PCL and more unlabeled images, we achieve performance competitive or superior to many state of the art weakly-supervised detection solutions.
@article{R24,
title = {Training Object Detectors from Few Weakly-Labeled and Many Unlabeled Images},
author = {Yang, Zhaohui and Shi, Miaojing and Avrithis, Yannis and Xu, Chao and Ferrari, Vittorio},
journal = {arXiv preprint arXiv:1912.00384},
month = {12},
year = {2019}
}
Active learning typically focuses on training a model on few labeled examples alone, while unlabeled ones are only used for acquisition. In this work we depart from this setting by using both labeled and unlabeled data during model training across active learning cycles. We do so by using unsupervised feature learning at the beginning of the active learning pipeline and semi-supervised learning at every active learning cycle, on all available data. The former has not been investigated before in active learning, while the study of latter in the context of deep learning is scarce and recent findings are not conclusive with respect to its benefit. Our idea is orthogonal to acquisition strategies by using more data, much like ensemble methods use more models. By systematically evaluating on a number of popular acquisition strategies and datasets, we find that the use of unlabeled data during model training brings a spectacular accuracy improvement in image classification, compared to the differences between acquisition strategies. We thus explore smaller label budgets, even one label per class.
@article{R23,
title = {Rethinking deep active learning: Using unlabeled data at model training},
author = {Sim\'eoni, Oriane and Budnik, Mateusz and Avrithis, Yannis and Gravier, Guillaume},
journal = {arXiv preprint arXiv:1911.08177},
month = {11},
year = {2019}
}
In this work we consider the problem of learning a classifier from noisy labels when a few clean labeled examples are given. The structure of clean and noisy data is modeled by a graph per class and Graph Convolutional Networks (GCN) are used to predict class relevance of noisy examples. For each class, the GCN is treated as a binary classifier learning to discriminate clean from noisy examples using a weighted binary cross-entropy loss function, and then the GCN-inferred "clean" probability is exploited as a relevance measure. Each noisy example is weighted by its relevance when learning a classifier for the end task. We evaluate our method on an extended version of a few-shot learning problem, where the few clean examples of novel classes are supplemented with additional noisy data. Experimental results show that our GCN-based cleaning process significantly improves the classification accuracy over not cleaning the noisy data and standard few-shot classification where only few clean examples are used. The proposed GCN-based method outperforms the transductive approach (Douze et al., 2018) that is using the same additional data without labels.
@article{R22,
title = {Graph convolutional networks for learning with few clean and many noisy labels},
author = {Iscen, Ahmet and Tolias, Giorgos and Avrithis, Yannis and Chum, Ond\v{r}ej and Schmid, Cordelia},
journal = {arXiv preprint arXiv:1910.00324},
month = {10},
year = {2019}
}
We propose a novel method of deep spatial matching (DSM) for image retrieval. Initial ranking is based on image descriptors extracted from convolutional neural network activations by global pooling, as in recent state-of-the-art work. However, the same sparse 3D activation tensor is also approximated by a collection of local features. These local features are then robustly matched to approximate the optimal alignment of the tensors. This happens without any network modification, additional layers or training. No local feature detection happens on the original image. No local feature descriptors and no visual vocabulary are needed throughout the whole process.
We experimentally show that the proposed method achieves the state-of-the-art performance on standard benchmarks across different network architectures and different global pooling methods. The highest gain in performance is achieved when diffusion on the nearest-neighbor graph of global descriptors is initiated from spatially verified images.
@article{R21,
title = {Local Features and Visual Words Emerge in Activations},
author = {Sim\'eoni, Oriane and Avrithis, Yannis and Chum, Ond\v{r}ej},
journal = {arXiv preprint arXiv:1905.06358},
month = {5},
year = {2019}
}
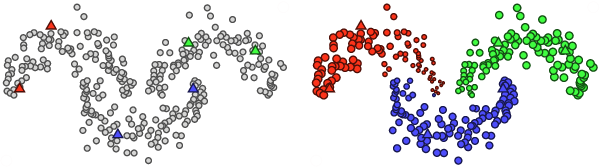
Semi-supervised learning is becoming increasingly important because it can combine data carefully labeled by humans with abundant unlabeled data to train deep neural networks. Classic methods on semi-supervised learning that have focused on transductive learning have not been fully exploited in the inductive framework followed by modern deep learning. The same holds for the manifold assumption---that similar examples should get the same prediction. In this work, we employ a transductive label propagation method that is based on the manifold assumption to make predictions on the entire dataset and use these predictions to generate pseudo-labels for the unlabeled data and train a deep neural network. At the core of the transductive method lies a nearest neighbor graph of the dataset that we create based on the embeddings of the same network.Therefore our learning process iterates between these two steps. We improve performance on several datasets especially in the few labels regime and show that our work is complementary to current state of the art.
@article{R20,
title = {Label propagation for Deep Semi-supervised Learning},
author = {Iscen, Ahmet and Tolias, Giorgos and Avrithis, Yannis and Chum, Ond\v{r}ej},
journal = {arXiv preprint arXiv:1904.04717},
month = {4},
year = {2019}
}
This paper investigates the visual quality of the adversarial examples. Recent papers propose to smooth the perturbations to get rid of high frequency artefacts. In this work, smoothing has a different meaning as it perceptually shapes the perturbation according to the visual content of the image to be attacked. The perturbation becomes locally smooth on the flat areas of the input image, but it may be noisy on its textured areas and sharp across its edges.
This operation relies on Laplacian smoothing, well-known in graph signal processing, which we integrate in the attack pipeline. We benchmark several attacks with and without smoothing under a white-box scenario and evaluate their transferability. Despite the additional constraint of smoothness, our attack has the same probability of success at lower distortion.
@article{R19,
title = {Smooth Adversarial Examples},
author = {Zhang, Hanwei and Avrithis, Yannis and Furon, Teddy and Amsaleg, Laurent},
journal = {arXiv preprint arXiv:1903.11862},
month = {3},
year = {2019}
}
Training deep neural networks from few examples is a highly challenging and key problem for many computer vision tasks. In this context, we are targeting knowledge transfer from a set with abundant data to other sets with few available examples. We propose two simple and effective solutions: (i) dense classification over feature maps, which for the first time studies local activations in the domain of few-shot learning, and (ii) implanting, that is, attaching new neurons to a previously trained network to learn new, task-specific features. On miniImageNet, we improve the prior state-of-the-art on few-shot classification, i.e., we achieve 62.5%, 79.8% and 83.8% on 5-way 1-shot, 5-shot and 10-shot settings respectively.
@article{R18,
title = {Dense Classification and Implanting for Few-Shot Learning},
author = {Lifchitz, Yann and Avrithis, Yannis and Picard, Sylvaine and Bursuc, Andrei},
journal = {arXiv preprint arXiv:1903.05050},
month = {3},
year = {2019}
}
2018
State of the art image retrieval performance is achieved with CNN features and manifold ranking using a k-NN similarity graph that is pre-computed off-line. The two most successful existing approaches are temporal filtering, where manifold ranking amounts to solving a sparse linear system online, and spectral filtering, where eigen-decomposition of the adjacency matrix is performed off-line and then manifold ranking amounts to dot-product search online. The former suffers from expensive queries and the latter from significant space overhead. Here we introduce a novel, theoretically well-founded hybrid filtering approach allowing full control of the space-time trade-off between these two extremes. Experimentally, we verify that our hybrid method delivers results on par with the state of the art, with lower memory demands compared to spectral filtering approaches and faster compared to temporal filtering.
@article{R17,
title = {Hybrid Diffusion: Spectral-Temporal Graph Filtering for Manifold Ranking},
author = {Iscen, Ahmet and Avrithis, Yannis and Tolias, Giorgos and Furon, Teddy and Chum, Ond\v{r}ej},
journal = {arXiv preprint arXiv:1807.08692},
month = {7},
year = {2018}
}
In this paper we address issues with image retrieval benchmarking on standard and popular Oxford 5k and Paris 6k datasets. In particular, annotation errors, the size of the dataset, and the level of challenge are addressed: new annotation for both datasets is created with an extra attention to the reliability of the ground truth. Three new protocols of varying difficulty are introduced. The protocols allow fair comparison between different methods, including those using a dataset pre-processing stage. For each dataset, 15 new challenging queries are introduced. Finally, a new set of 1M hard, semi-automatically cleaned distractors is selected.
An extensive comparison of the state-of-the-art methods is performed on the new benchmark. Different types of methods are evaluated, ranging from local-feature-based to modern CNN based methods. The best results are achieved by taking the best of the two worlds. Most importantly, image retrieval appears far from being solved.
@article{R16,
title = {Revisiting Oxford and Paris: Large-Scale Image Retrieval Benchmarking},
author = {Radenovi\'c, Filip and Iscen, Ahmet and Tolias, Giorgos and Avrithis, Yannis and Chum, Ond\v{r}ej},
journal = {arXiv preprint arXiv:1803.11285},
month = {3},
year = {2018}
}
In this work we present a novel unsupervised framework for hard training example mining. The only input to the method is a collection of images relevant to the target application and a meaningful initial representation, provided e.g. by pre-trained CNN. Positive examples are distant points on a single manifold, while negative examples are nearby points on different manifolds. Both types of examples are revealed by disagreements between Euclidean and manifold similarities. The discovered examples can be used in training with any discriminative loss. The method is applied to unsupervised fine-tuning of pre-trained networks for fine-grained classification and particular object retrieval. Our models are on par or are outperforming prior models that are fully or partially supervised.
@article{R15,
title = {Mining on Manifolds: Metric Learning without Labels},
author = {Iscen, Ahmet and Tolias, Giorgos and Avrithis, Yannis and Chum, Ond\v{r}ej},
journal = {arXiv preprint arXiv:1803.11095},
month = {3},
year = {2018}
}
2017
Severe background clutter is challenging in many computer vision tasks, including large-scale image retrieval. Global descriptors, that are popular due to their memory and search efficiency, are especially prone to corruption by such a clutter. Eliminating the impact of the clutter on the image descriptor increases the chance of retrieving relevant images and prevents topic drift due to actually retrieving the clutter in the case of query expansion. In this work, we propose a novel salient region detection method. It captures, in an unsupervised manner, patterns that are both discriminative and common in the dataset. Saliency is based on a centrality measure of a nearest neighbor graph constructed from regional CNN representations of dataset images. The descriptors derived from the salient regions improve particular object retrieval, most noticeably in a large collections containing small objects.
@article{R14,
title = {Unsupervised object discovery for instance recognition},
author = {Sim\'eoni, Oriane and Iscen, Ahmet and Tolias, Giorgos and Avrithis, Yannis and Chum, Ond\v{r}ej},
journal = {arXiv preprint arXiv:1709.04725},
month = {9},
year = {2017}
}
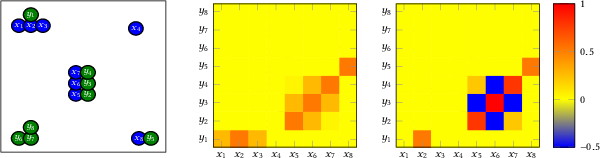
Location recognition is commonly treated as visual instance retrieval on "street view" imagery. The dataset items and queries are panoramic views, i.e. groups of images taken at a single location. This work introduces a novel panorama-to-panorama matching process, either by aggregating features of individual images in a group or by explicitly constructing a larger panorama. In either case, multiple views are used as queries. We reach near perfect location recognition on a standard benchmark with only four query views.
@article{R13,
title = {Panorama to panorama matching for location recognition},
author = {Iscen, Ahmet and Tolias, Giorgos and Avrithis, Yannis and Furon, Teddy and Chum, Ond\v{r}ej},
journal = {arXiv preprint arXiv:1704.06591},
month = {4},
year = {2017}
}
Part-based image classification aims at representing categories by small sets of learned discriminative parts, upon which an image representation is built. Considered as a promising avenue a decade ago, this direction has been neglected since the advent of deep neural networks. In this context, this paper brings two contributions: first, it shows that despite the recent success of end-to-end holistic models, explicit part learning can boosts classification performance. Second, this work proceeds one step further than recent part-based models (PBM), focusing on how to learn parts without using any labeled data. Instead of learning a set of parts per class, as generally done in the PBM literature, the proposed approach both constructs a partition of a given set of images into visually similar groups, and subsequently learn a set of discriminative parts per group in a fully unsupervised fashion. This strategy opens the door to the use of PBM in new applications for which the notion of image categories is irrelevant, such as instance-based image retrieval, for example. We experimentally show that our learned parts can help building efficient image representations, for classification as well as for indexing tasks, resulting in performance superior to holistic state-of-the art Deep Convolutional Neural Networks (DCNN) encoding.
@article{R12,
title = {Unsupervised part learning for visual recognition},
author = {Sicre, Ronan and Avrithis, Yannis and Kijak, Ewa and Jurie, Fr\'ed\'eric},
journal = {arXiv preprint arXiv:1704.03755},
month = {4},
year = {2017}
}
Despite the success of deep learning on representing images for particular object retrieval, recent studies show that the learned representations still lie on manifolds in a high dimensional space. Therefore, nearest neighbor search cannot be expected to be optimal for this task. Even if a nearest neighbor graph is computed offline, exploring the manifolds online remains expensive. This work introduces an explicit embedding reducing manifold search to Euclidean search followed by dot product similarity search. We show this is equivalent to linear graph filtering of a sparse signal in the frequency domain, and we introduce a scalable offline computation of an approximate Fourier basis of the graph. We improve the state of art on standard particular object retrieval datasets including a challenging one containing small objects. At a scale of 10^5 images, the offline cost is only a few hours, while query time is comparable to standard similarity search.
@article{R11,
title = {Fast Spectral Ranking for Similarity Search},
author = {Iscen, Ahmet and Avrithis, Yannis and Tolias, Giorgos and Furon, Teddy and Chum, Ond\v{r}ej},
journal = {arXiv preprint arXiv:1703.06935},
month = {3},
year = {2017}
}
2016
Query expansion is a popular method to improve the quality of image retrieval with both conventional and CNN representations. It has been so far limited to global image similarity. This work focuses on diffusion, a mechanism that captures the image manifold in the feature space. The diffusion is carried out on descriptors of overlapping image regions rather than on a global image descriptor like in previous approaches. An efficient off-line stage allows optional reduction in the number of stored regions. In the on-line stage, the proposed handling of unseen queries in the indexing stage removes additional computation to adjust the precomputed data. A novel way to perform diffusion through a sparse linear system solver yields practical query times well below one second. Experimentally, we observe a significant boost in performance of image retrieval with compact CNN descriptors on standard benchmarks, especially when the query object covers only a small part of the image. Small objects have been a common failure case of CNN-based retrieval.
@article{R10,
title = {Efficient Diffusion on Region Manifolds: Recovering Small Objects with Compact {CNN} Representations},
author = {Iscen, Ahmet and Tolias, Giorgos and Avrithis, Yannis and Furon, Teddy and Chum, Ond\v{r}ej},
journal = {arXiv preprint arXiv:1611.05113},
month = {11},
year = {2016}
}
Part-based image classification consists in representing categories by small sets of discriminative parts upon which a representation of the images is built. This paper addresses the question of how to automatically learn such parts from a set of labeled training images. The training of parts is cast as a quadratic assignment problem in which optimal correspondences between image regions and parts are automatically learned. The paper analyses different assignment strategies and thoroughly evaluates them on two public datasets: Willow actions and MIT 67 scenes. State-of-the art results are obtained on these datasets.
@article{R9,
title = {Automatic Discovery of Discriminative Parts As a Quadratic Assignment Problem},
author = {Sicre, Ronan and Rabin, Julien and Avrithis, Yannis and Furon, Teddy and Jurie, Fr\'ed\'eric},
journal = {arXiv preprint arXiv:1611.04413},
month = {11},
year = {2016}
}
We propose a new data-structure, the generalized randomized k-d forest, or k-d GeRaF, for approximate nearest neighbor searching in high dimensions. In particular, we introduce new randomization techniques to specify a set of independently constructed trees where search is performed simultaneously, hence increasing accuracy. We omit backtracking, and we optimize distance computations, thus accelerating queries. We release public domain software GeRaF and we compare it to existing implementations of state-of-the-art methods including BBD-trees, Locality Sensitive Hashing, randomized k-d forests, and product quantization. Experimental results indicate that our method would be the method of choice in dimensions around 1,000, and probably up to 10,000, and pointsets of cardinality up to a few hundred thousands or even one million; this range of inputs is encountered in many critical applications today. For instance, we handle a real dataset of 10^6 images represented in 960 dimensions with a query time of less than 1sec on average and 90% responses being true nearest neighbors.
@article{R8,
title = {High-Dimensional Approximate Nearest Neighbor: {k-d} Generalized Randomized Forests},
author = {Avrithis, Yannis and Emiris, Ioannis and Samaras, Georgios},
journal = {arXiv preprint arXiv:1603.09596},
month = {3},
year = {2016}
}
2008
In this paper we describe K-Space's participation in TRECVid 2008 in the interactive search task. For 2008 the K-Space group performed one of the largest interactive video information retrieval experiments conducted in a laboratory setting. We had three institutions participating in a multi-site multi-system experiment. In total 36 users participated, 12 each from Dublin City University (DCU, Ireland), University of Glasgow (GU, Scotland) and Centrum Wiskunde & Informatica (CWI, the Netherlands). Three user interfaces were developed, two from DCU which were also used in 2007 as well as an interface from GU. All interfaces leveraged the same search service. Using a latin squares arrangement, each user conducted 12 topics, leading in total to 6 runs per site, 18 in total. We officially submitted for evaluation 3 of these runs to NIST with an additional expert run using a 4th system. Our submitted runs performed around the median. In this paper we will present an overview of the search system utilized, the experimental setup and a preliminary analysis of our results.
@inproceedings{R7,
title = {{K-Space} at {TRECVID} 2008},
author = {Tolias, Giorgos and Spyrou, Evaggelos and Avrithis, Yannis},
booktitle = {Proceedings of 6th TRECVID Workshop},
month = {11},
address = {Gaithersburg, USA},
year = {2008}
}
In this paper, we give an overview of the four tasks submitted to TRECVID 2008 by COST292. The high-level feature extraction framework comprises four systems. The first system transforms a set of low-level descriptors into the semantic space using Latent Semantic Analysis and utilises neural networks for feature detection. The second system uses a multi-modal classifier based on SVMs and several descriptors. The third system uses three image classifiers based on ant colony optimisation, particle swarm optimisation and a multi-objective learning algorithm.The fourth system uses a Gaussian model for singing detection and a person detection algorithm. The search task is based on an interactive retrieval application combining retrieval functionalities in various modalities with a user interface supporting automatic and interactive search over all queries submitted. The rushes task submission is based on a spectral clustering approach for removing similar scenes based on eigenvalues of frame similarity matrix and and a redundancy removal strategy which depends on semantic features extraction such as camera motion and faces. Finally, the submission to the copy detection task is conducted by two different systems. The first system consists of a video module and an audio module. The second system is based on mid-level features that are related to the temporal structure of videos.
@inproceedings{R6,
title = {{COST292} experimental framework for {TRECVID} 2008},
author = {Tolias, Giorgos and Spyrou, Evaggelos and Kapsalas, Petros and Avrithis, Yannis},
booktitle = {Proceedings of 6th TRECVID Workshop},
month = {11},
address = {Gaithersburg, USA},
year = {2008}
}
2007
In this paper we describe K-Space participation in TRECVid 2007. K-Space participated in two tasks, high-level feature extraction and interactive search. We present our approaches for each of these activities and provide a brief analysis of our results. Our high-level feature submission utilized multi-modal low-level features which included visual, audio and temporal elements. Specific concept detectors (such as Face detectors) developed by K-Space partners were also used. We experimented with different machine learning approaches including logistic regression and support vector machines (SVM). Finally we also experimented with both early and late fusion for feature combination. This year we also participated in interactive search, submitting 6 runs. We developed two interfaces which both utilized the same retrieval functionality. Our objective was to measure the effect of context, which was supported to different degrees in each interface, on user performance. The first of the two systems was a 'shot' based interface, where the results from a query were presented as a ranked list of shots. The second interface was 'broadcast' based, where results were presented as a ranked list of broadcasts. Both systems made use of the outputs of our high-level feature submission as well as low-level visual features.
@inproceedings{R5,
title = {{K-Space} at {TRECVID} 2007},
author = {Spyrou, Evaggelos and Avrithis, Yannis},
booktitle = {Proceedings of 5th TRECVID Workshop},
month = {11},
address = {Gaithersburg, USA},
year = {2007}
}
In this paper, we give an overview of the four tasks submitted to TRECVID 2007 by COST292. In shot boundary (SB) detection task, four SB detectors have been developed and the results are merged using two merging algorithms. The framework developed for the high-level feature extraction task comprises four systems. The first system transforms a set of low-level descriptors into the semantic space using Latent Semantic Analysis and utilises neural networks for feature detection. The second system uses a Bayesian classifier trained with a "bag of subregions". The third system uses a multi-modal classifier based on SVMs and several descriptors. The fourth system uses two image classifiers based on ant colony optimisation and particle swarm optimisation respectively. The system submitted to the search task is an interactive retrieval application combining retrieval functionalities in various modalities with a user interface supporting automatic and interactive search over all queries submitted. Finally, the rushes task submission is based on a video summarisation and browsing system comprising two different interest curve algorithms and three features.
@inproceedings{R4,
title = {The {COST292} experimental framework for {TRECVID} 2007},
author = {Spyrou, Evaggelos and Kapsalas, Petros and Tolias, Giorgos and Mylonas, Phivos and Avrithis, Yannis},
booktitle = {Proceedings of 5th TRECVID Workshop},
month = {11},
address = {Gaithersburg, USA},
year = {2007}
}
2006
In this paper we describe the K-Space participation in TRECVid 2006. K-Space participated in two tasks, high-level feature extraction and search. We present our approaches for each of these activities and provide a brief analysis of our results. Our high-level feature submission made use of support vector machines (SVMs) created with low-level MPEG-7 visual features, fused with specific concept detectors. Search submissions were both manual and automatic and made use of both low- and high-level features. In the high-level feature extraction submission, four of our six runs achieved performance above the TRECVid median, whilst our search submission performed around the median. The K-Space team consisted of eight partner institutions from the EU-funded K-Space Network, and our submissions made use of tools and techniques from each partner. As such this paper will provide overviews of each partner's contributions and provide appropriate references for specific descriptions of individual components.
@inproceedings{R3,
title = {{K-Space} at {TRECVID} 2006},
author = {Spyrou, Evaggelos and Koumoulos, George and Avrithis, Yannis},
booktitle = {Proceedings of 4th TRECVID Workshop},
month = {11},
address = {Gaithersburg, USA},
year = {2006}
}
In this paper we give an overview of the four TRECVID tasks submitted by COST292, European network of institutions in the area of semantic multimodal analysis and retrieval of digital video media. Initially,we present shot boundary evaluation method based on results merged using a confidence measure. The two SB detectors user here are presented, one of the Technical University of Delft and one of the LaBRI, University of Bordeaux 1, followed by the description of the merging algorithm. The high-level feature extraction task comprises three separate systems. The first system, developed by the National Technical University of Athens (NTUA) utilises a set of MPEG-7 low-level descriptors and Latent Semantic Analysis to detect the features. The second system, developed by Bilkent University, uses a Bayesian classifier trained with a "bag of subregions" for each keyframe. The third system by the Middle East Technical University (METU) exploits textual information in the video using character recognition methodology. The system submitted to the search task is an interactive retrieval application developed by Queen Mary, University of London, University of Zilina and ITI from Thessaloniki, combining basic retrieval functionalities in various modalities (i.e. visual, audio, textual) with a user interface supporting the submission of queries using any combination of the available retrieval tools and the accumulation of relevant retrieval results over all queries submitted by a single user during a specified time interval. Finally, the rushes task submission comprises a video summarisation and browsing system specifically designed to intuitively and efficiently presents rushes material in video production environment. This system is a result of joint work of University of Bristol, Technical University of Delft and LaBRI, University of Bordeaux 1.
@inproceedings{R2,
title = {{COST292} experimental framework for {TRECVID} 2006},
author = {Spyrou, Evaggelos and Koumoulos, George and Avrithis, Yannis},
booktitle = {Proceedings of 4th TRECVID Workshop},
month = {11},
address = {Gaithersburg, USA},
year = {2006}
}
1999
Motion Estimation (ME) is an important part of the MPEG-4 encoder, since it could significantly affect the output quality of the encoded sequence. Unfortunately this feature requires a significant part of the encoding time especially when using the straightforward Full Search (FS) Algorithm. The Diamond Search (DS) was recently accepted as a fast motion estimation algorithm for the MPEG4 VM. In this report we verify the results extracted by the Advanced Diamond Zonal Search with Embedded Radar algorithm (ADZS-ER), proposed by Alexis M. Tourapis, Oscar C. Au, Ming L. Liou, and Guobin Shen (ISO/IEC JTC1/SC29/WG11, MPEG99/M4980). The experiments were carried out under the same conditions and the results verify the superiority of the proposed algorithm towards the DS algorithm, especially in the high bit rate cases, regarding the speed (in terms of number of checking points and total encoding time) and the quality (in terms of PSNR) of the output sequence.
@techreport{R1,
title = {Verification Report of Core Experiment on Fast Block-Matching Motion Estimation using Advanced Diamond Zonal Search with Embedded Radar},
author = {Tsechpenakis, Gabriel and Avrithis, Yannis and Kollias, Stefanos},
institution = {ISO/IEC JTC1/SC29/WG11},
number = {MPEG99/M5116},
month = {10},
year = {1999}
}
Book chapters
2022
Ed. by William Puech
pp. 41-75 Wiley, 2022
Machine learning using deep neural networks applied to image recognition works extremely well. However, it is possible to modify the images very slightly and intentionally, with modifications almost invisible to the eye, to deceive the classification system into misclassifying such content into the incorrect visual category. This chapter provides an overview of these intentional attacks, as well as the defense mechanisms used to counter them.
@incollection{B9,
title = {Deep Neural Network Attacks and Defense: The Case of Image Classification},
author = {Zhang, Hanwei and Furon, Teddy and Amsaleg, Laurent and Avrithis, Yannis},
publisher = {Wiley},
booktitle = {Multimedia Security 1: Authentication and Data Hiding},
editor = {William Puech},
pages = {41--75},
year = {2022}
}
2011
Ed. by V. Cutsuridis, A. Hussain, J.G. Taylor
pp. 363-386 Springer, 2011
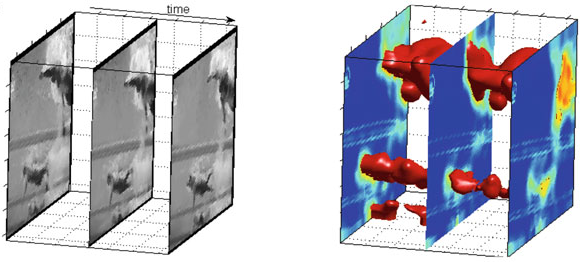
Biological visual attention has been long studied by experts in the field of cognitive psychology. The Holy Grail of this study is the exact modeling of the interaction between the visual sensory and the process of perception. It seems that there is an informal agreement on the four important functions of the attention process: (a) the bottom-up process, which is responsible for the saliency of the input stimuli; (b) the top-down process that bias attention toward known areas or regions of predefined characteristics; (c) the attentional selection that fuses information derived from the two previous processes and enables focus; and (d) the dynamic evolution of the attentional selection process. In the following, we will outline established computational solutions for each of the four functions.
@incollection{B8,
title = {Vision, Attention Control, and Goals Creation System},
author = {Rapantzikos, Konstantinos and Avrithis, Yannis and Kollias, Stefanos},
publisher = {Springer},
booktitle = {Perception-Action Cycle: Models, Architectures and Hardware},
editor = {V. Cutsuridis and A. Hussain and J.G. Taylor},
pages = {363--386},
year = {2011}
}
2009
Ed. by R. Troncy, B. Huet, S. Schenk
pp. 163-181 Wiley, 2009
In this chapter a first attempt will be made to examine how the coupling of multimedia processing and knowledge representation techniques, presented separately in previous chapters, can improve analysis. No formal reasoning techniques will be introduced at this stage; our exploration of how multimedia analysis and knowledge can be combined will start by revisiting the image and video segmentation problem. Semantic segmentation, presented in the first section of this chapter, starts with an elementary segmentation and region classification and refines it using similarity measures and merging criteria defined at the semantic level. Our discussion will continue in the next sections of the chapter with knowledge-driven classification approaches, which exploit knowledge in the form of contextual information for refining elementary classification results obtained via machine learning. Two relevant approaches will be presented. The first one deals with visual context and treats it as interaction between global classification and local region labels. The second one deals with spatial context and formulates the exploitation of it as a global optimization problem.
@incollection{B7,
title = {Knowledge Driven Segmentation and Classification},
author = {Athanasiadis, Thanos and Mylonas, Phivos and Papadopoulos, Georgios and Mezaris, Vasileios and Avrithis, Yannis and Kompatsiaris, Ioannis and Strintzis, Michael G.},
publisher = {Wiley},
booktitle = {Multimedia Semantics: Metadata, Analysis and Interaction},
editor = {R. Troncy and B. Huet and S. Schenk},
pages = {163--181},
year = {2009}
}
2008
Ed. by P. Maragos, A. Potamianos, P. Gros
pp. 179-199 Springer, 2008
Although human perception appears to be automatic and unconscious, complex sensory mechanisms exist that form the preattentive component of understanding and lead to awareness. Considerable research has been carried out into these preattentive mechanisms and computational models have been developed for similar problems in the fields of computer vision and speech analysis. The focus here is to explore aural nd visual information in video streams for modeling attention and detecting salient events. The separate aural and visual modules may convey explicit, complementary or mutually exclusive information around the detected audio-visual events. Based on recent studies on perceptual and computational attention modeling, we formulate measures of attention using features of saliency for the audio-visual stream. Audio saliency is captured by signal modulations and related multifrequency band features, extracted through nonlinear operators and energy tracking. Visual saliency is measured by means of a spatiotemporal attention model driven by various feature cues (intensity, color, motion). Features from both modules mapped to one-dimensional, time-varying saliency curves, from which statistics of salient segments can be extracted and important audio or visual events can be detected through adaptive, threshold-based mechanisms. Audio and video curves are integrated in a single attention curve, where events may be enhanced, suppressed or vanished. Salient events from the audio-visual curve are detected through geometrical features such as local extrema, sharp transitions and level sets. The potential of inter-module fusion and audio-visual event detection is demonstrated in applications such as video key-frame selection, video skimming and video annotation.
@incollection{B6,
title = {Audiovisual Attention Modeling and Salient Event Detection},
author = {Evangelopoulos, Georgios and Rapantzikos, Konstantinos and Maragos, Petros and Avrithis, Yannis and Potamianos, Alexandros},
publisher = {Springer},
booktitle = {Multimodal Processing and Interaction: Audio, Video, Text},
editor = {P. Maragos and A. Potamianos and P. Gros},
pages = {179--199},
year = {2008}
}
Ed. by Y. Kompatsiaris, P. Hobson
pp. 99-122 Springer, 2008
In this chapter,we propose an ontology-based framework for enhancing segment-level annotations resulting from typical image analysis, through the exploitation of visual context and topological information. The concepts (objects) of interest and their spatial topology are modelled in RDFS ontologies, and through the use of reification, a fuzzy ontological representation is achieved, enabling the seamless integration of contextual knowledge. The formalisation of contextual information enables a first refinement of the input image analysis annotations utilising the semantic associations that characterise the context of appearance.
@incollection{B5,
title = {Introducing Context and Reasoning in Visual Content Analysis: An Ontology-based Framework},
author = {Dasiopoulou, Stamatia and Saathoff, Carsten and Mylonas, Phivos and Avrithis, Yannis and Kompatsiaris, Yiannis and Staab, Steffen},
publisher = {Springer},
booktitle = {Semantic Multimedia and Ontologies: Theory and Applications},
editor = {Y. Kompatsiaris and P. Hobson},
month = {1},
pages = {99--122},
edition = {1st},
isbn = {978-1-84800-075-9},
year = {2008}
}
2006
Ed. by R. Lukac, K.N. Plataniotis
pp. 259-284 CRC Press, 2006
This chapter discusses semantic image analysis for the purpose of automatic image understanding and efficient visual content access and retrieval at semantic level. It presents the current state of the art analysis approaches aiming at bridging the "semantic gap" in image analysis and retrieval, highlights the major achievements of the existing approaches and sheds light to the challenges still unsolved. Its main subject is to present a generic framework for performing knowledge-assisted semantic analysis of images and also to present the Knowledge-Assisted Analysis as performed in the aceMedia project.
@incollection{B4,
title = {Semantic Processing of Color Images},
author = {Dasiopoulou, Stamatia and Spyrou, Evaggelos and Avrithis, Yannis and Kompatsiaris, Yiannis and Strintzis, Michael G.},
publisher = {CRC Press},
booktitle = {Color Image Processing: Emerging Applications},
editor = {R. Lukac and K.N. Plataniotis},
pages = {259--284},
year = {2006}
}
Ed. by Z. Ma
pp. 247-272 Springer, 2006
The semantic gap is the main problem of content based multimedia retrieval. This refers to the extraction of the semantic content of multimedia documents, the understanding of user information needs and requests, as well as to the matching between the two. In this chapter we focus on the analysis of multimedia documents for the extraction of their semantic content. Our approach is based on fuzzy algebra, as well as fuzzy ontological information. We start by outlining the methodologies that may lead to the creation of a semantic index; these methodologies are integrated in a video annotating environment. Based on the semantic index, we then explain how multimedia content may be analyzed for the extraction of semantic information in the form of thematic categorization. The latter relies on stored knowledge and a fuzzy hierarchical clustering algorithm that uses a similarity measure that is based on the notion of context.
@incollection{B3,
title = {Automatic thematic categorization of multimedia documents using ontological information and fuzzy algebra},
author = {Wallace, Manolis and Mylonas, Phivos and Akrivas, Giorgos and Avrithis, Yannis and Kollias, Stefanos},
publisher = {Springer},
booktitle = {Soft Computing in Ontologies and Semantic Web},
editor = {Z. Ma},
volume = {204},
pages = {247--272},
year = {2006}
}
2005
Ed. by G. Stamou, S. Kollias
pp. 299-338 Wiley, 2005
In this chapter, an integrated information system is presented that offers enhanced search and retrieval capabilities to users of heterogeneous digital audiovisual archives. This novel system exploits the advances in handling a/v content related metadata, as introduced by MPEG-7 and worked out by MPEG-21, to offer advanced access services characterized by the tri-fold "semantic phrasing of the request (query)", "unified handling" of multimedia documents and "personalized response". The proposed system is targeting the intelligent extraction of semantic information from multimedia document descriptions, taking into account the nature of useful queries that users may issue, and the context determined by user profiles. From a technical point of view, it plays the role of an intermediate access server residing between the end users and multiple diverse in nature audiovisual archives, organized according to the latest MPEG standards.
@incollection{B2,
title = {Knowledge-Based Multimedia Content Indexing and Retrieval},
author = {Wallace, Manolis and Avrithis, Yannis and Stamou, Giorgos and Kollias, Stefanos},
publisher = {Wiley},
booktitle = {Multimedia Content and Semantic Web: Methods, Standards and Tools},
editor = {G. Stamou and S. Kollias},
month = {8},
pages = {299-338},
year = {2005}
}
2002
Ed. by E. Kerre
pp. 195-215 Springer, 2002
Fusion of multiple cue image partitions is described as an indispensable tool towards the goal of automatic object-based image and video segmentation, interpretation and coding. Since these tasks involve human cognition and knowledge of image semantics, which are absent in most cases, fusion of all available cues is crucial for effective segmentation of generic video sequences. This chapter investigates fuzzy data fusion techniques which are capable of integrating the results of multiple cue segmentation and provide time consistent spatiotemporal image partitions corresponding to moving objects.
@incollection{B1,
title = {Fuzzy Data Fusion For Multiple Cue Image And Video Segmentation},
author = {Ioannou, Spyros and Avrithis, Yannis and Stamou, Giorgos and Kollias, Stefanos},
publisher = {Springer},
booktitle = {Fuzzy Technologies and Applications},
editor = {E. Kerre},
month = {5},
pages = {195--215},
year = {2002}
}
Theses
2020
University of Rennes 1
Rennes, France Jul 2020
This manuscript is about a journey. The journey of computer vision and machine learning research from the early years of Gabor filters and linear classifiers to surpassing human skills in several tasks today. The journey of the author's own research, designing representations and matching processes to explore visual data and exploring visual data to learn better representations.
Part I addresses instance-level visual search and clustering, building on shallow visual representations and matching processes. The representation is obtained by a pipeline of local features, hand-crafted descriptors and visual vocabularies. Improvements in the pipeline are introduced, including the construction of large-scale vocabularies, spatial matching for geometry verification, representations beyond vocabularies and nearest neighbor search. Applications to exploring photo collections are discussed, including location recognition, landmark recognition and automatic discovery of photos depicting the same scene.
Part II addresses instance-level visual search and object discovery, building on deep visual representations and matching processes, focusing on the manifold structure of the feature space. The representation is obtained by deep parametric models learned from visual data. Contributions are made to advancing manifold search over global or regional CNN representations. This process is seen as graph filtering, including spatial and spectral. Spatial matching is revisited with local features detected on CNN activations. Finally, a method is introduced for object discovery from CNN activations over an unlabeled image collection.
Part III addresses learning deep visual representations by exploring visual data, focusing on limited or no supervision. It progresses from instance-level to category-level tasks and studies the sensitivity of models to their input. It introduces methods for unsupervised metric learning and semi-supervised learning, based again on the manifold structure of the feature space. It contributes to few-shot learning, studying activation maps and learning multiple layers to convergence for the first time. Finally, it introduces an attack as an attempt to improve upon the visual quality of adversarial examples in terms of imperceptibility.
Part IV summarizes more of the author's past and present contributions, reflects on these contributions in the present context and consolidates the ideas exposed in this manuscript. It then attempts to draw a road map of ideas that are likely to come.
@phdthesis{T4,
type = {HDR Thesis},
title = {Exploring and Learning from Visual Data},
author = {Avrithis, Yannis},
school = {University of Rennes 1 (UR1)},
month = {7},
address = {Rennes, France},
year = {2020}
}
2001
School of Electrical and Computer Engineering
National Technical University of Athens
Athens, Greece Feb 2001
The main research area of this Ph.D. thesis is image and video sequence processing and analysis for description and indexing of their visual content. Its objective is to contribute to the development of an automated computational system that has the capabilities of object-based segmentation of audiovisual material, automatic content description and annotation, summarization for preview and browsing, as well as content-based search and retrieval. The thesis consists of four parts.
The first part introduces video sequence analysis, segmentation and object extraction based on color, motion, as well as depth field in the case of stereoscopic video sequences. A fusion technique is proposed that combines individual cue segmentations and allows for reliable identification of semantic objects.
The second part refers to automatic annotation of the visual content by means of feature vectors calculated by multidimensional fuzzy classification of low-level object descriptors. This information is used for summarization, which is implemented by optimal selection of a limited set of key frames and shots providing meaningful visual content description. The representation of the selected material by feature vectors is then employed for content-based search and retrieval.
In the third part, the problem of object contour analysis and representation is examined, with application to shape-based object classification and retrieval. An original contour normalization scheme is presented, permitting invariant shape representation with respect to a large number of transformations without any actual loss of information.
In the fourth part, a novel technique is proposed for temporal segmentation and parsing of broadcast news recordings into elementary story units or news topics using visual cues. The technique is based on an advanced algorithm for automatic detection of human faces; the extracted information is also employed for the development of new semantic criteria for content-based retrieval.
@phdthesis{T3,
title = {Video Sequence Analysis for Content Description, Summarization and Content-Based Retrieval},
author = {Avrithis, Yannis},
school = {School of Electrical and Computer Engineering; National Technical University of Athens (NTUA)},
month = {2},
address = {Athens, Greece},
year = {2001}
}
1994
Department of Electrical and Electronic Engineering
Imperial College of Science, Technology and Medicine, University of London
London, UK Oct 1994
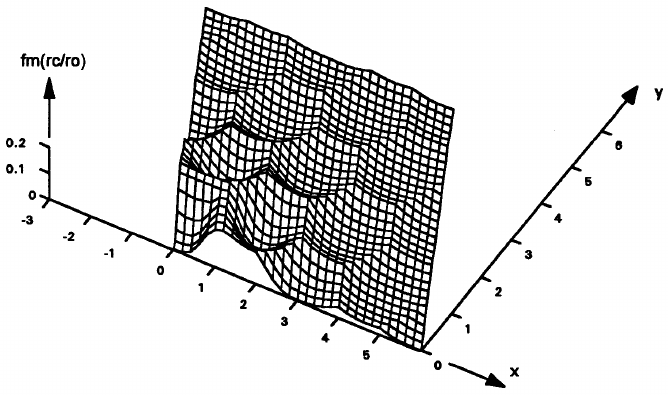
Code Division Multiple Access (CDMA) is a multiple access scheme based on spread spectrum techniques, that has been used for many years for military communications, and quite recently for commercial applications, such as satellite and digital cellular radio communications. Certain inherent characteristics of spread spectrum, such as interference and multipath suppression capabilities, privacy, and more efficient spectrum reuse, make CDMA advantageous for mobile cellular communications.
It is the intention of this project to provide an examination of how these properties of CDMA can be used to increase capacity comparing with conventional multiple access techniques. For this purpose, the capacity of a single-cell, power controlled, asynchronous direct-sequence (DS) CDMA system is first investigated using Gold codes and both binary and quadrature phase-shift-keying (BPSK and QPSK) modulation.
The investigation carries on with the calculation of both the forward and reverse link capacity of a multiple-cell CDMA system by means of analytical calculations and Monte Carlo simulations. Finally, a straightforward comparison with conventional techniques such as FDMA and TDMA shows that CDMA can indeed provide much higher capacity.
@mastersthesis{T2,
title = {Investigating the Capacity of a Cellular {CDMA} System},
author = {Avrithis, Yannis},
school = {Department of Electrical and Electronic Engineering; Imperial College of Science, Technology and Medicine, University of London (IC)},
month = {10},
address = {London, UK},
year = {1994}
}
1993
School of Electrical and Computer Engineering
National Technical University of Athens
Athens, Greece Sep 1993
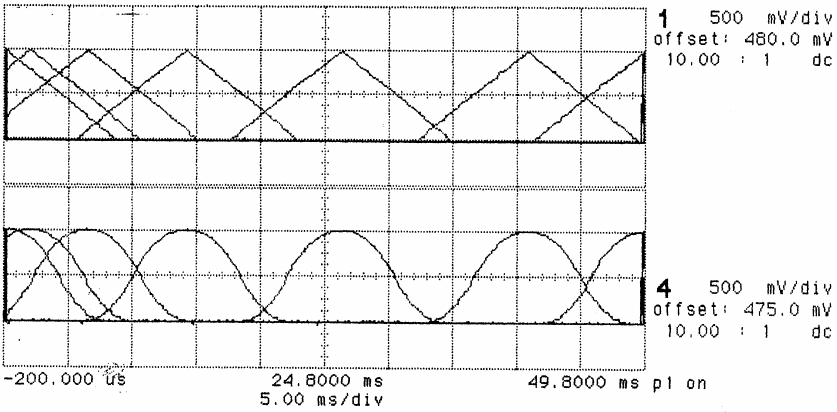
Fuzzy logic is a recent branch of mathematics that allows modelling of the imprecise way of reasoning that plays an important role in the ability of humans to make decisions in uncertain environments. Interest in fuzzy logic has grown in the last decade, with its successful use in diverse fields of study including expert systems, control systems, artificial intelligence, signal and image processing, computer vision, robotics, medical diagnosis, finance and decision support systems.
The first part of this thesis addresses the alternative types of operations that are available at each stage of computation, carries out a theoretical study of how these operations need to be combined into a consistent logical system and investigates new, simpler computational methods and conditions under which such methods can be used.
The second part concerns the analysis, design and implementation of a fuzzy rule-based controller, including a mechanical construction and an electronic circuit. The objective is to control the position of a ball inside a vertical transparent tube by measuring the actual position via an ultrasound sensor on top of the tube and accordingly adjusting the voltage applied to a fan that is fixed underneath. The fuzzy processor is implemented with discrete components and all computations are entirely analog, without any intermediate A/D or D/A conversion. Fuzzy membership functions and rules are specified by manually adjusting an array of potentiometers. This allows studying the effect of different parameters on the behavior of the controller in tasks like stabilizing the ball or moving it quickly between two positions. This work serves as a demonstrator and a testbed for future implementations with analog VLSI circuits.
@mastersthesis{T1,
title = {Fuzzy Logic Processor for Control Systems},
author = {Avrithis, Yannis},
school = {School of Electrical and Computer Engineering; National Technical University of Athens (NTUA)},
month = {9},
address = {Athens, Greece},
year = {1993}
}
Edited volumes
2009
Vol. 5371 Jan 2009
Springer ISBN 978-3-540-92891-1
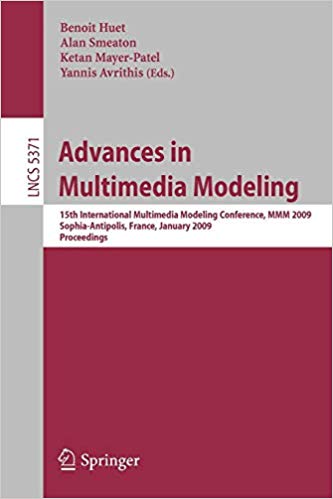
This book constitutes the refereed proceedings of the 15th International Multimedia Modeling Conference, MMM 2009, held in Sophia-Antipolis, France, in January 2009. The 26 revised full papers and 20 revised poster papers presented together with 2 invited talks were carefully reviewed and selected from 135 submissions. The papers are organized in topical sections on automated annotation, coding and streaming, video semantics and relevance, audio, recognition, classification and retrieval, as well as query and summarization.
@book{V3,
title = {Advances in Multimedia Modeling},
editor = {Huet, Benoit and Smeaton, Alan and Mayer-Patel, Ketan and Avrithis, Yannis},
publisher = {Springer},
series = {Lecture Notes in Computer Science (LNCS)},
volume = {5371},
month = {1},
isbn = {978-3-540-92891-1},
year = {2009}
}
2007
Vol. 4816 Dec 2007
Springer ISBN 978-3-540-77033-6
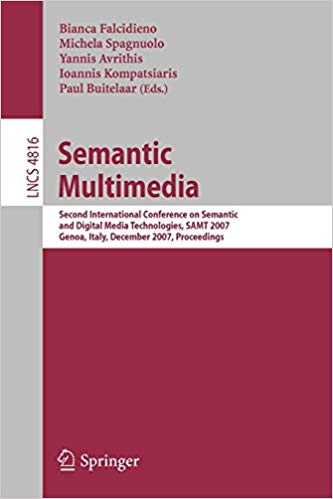
This book constitutes the refereed proceedings of the Second International Conference on Semantics and Digital Media Technologies, SAMT 2007, held in Genoa, Italy, in December 2007. The 16 revised full papers, 10 revised short papers and 10 poster papers presented together with three awarded PhD papers were carefully reviewed and selected from 55 submissions. The conference brings together forums, projects, institutions and individuals investigating the integration of knowledge, semantics and low-level multimedia processing, including new emerging media and application areas. The papers are organized in topical sections on knowledge based content processing, semantic multimedia annotation, domain-restricted generation of semantic metadata from multimodal sources, classification and annotation of multidimensional content, content adaptation, MX: the IEEE standard for interactive music, as well as poster papers and K-space awarded PhD papers.
@book{V2,
title = {Semantic Multimedia},
editor = {Falcidieno, Bianca and Spagnuolo, Michela and Avrithis, Yannis and Kompatsiaris, Ioannis and Buitelaar, Paul},
publisher = {Springer},
series = {Lecture Notes in Computer Science (LNCS)},
volume = {4816},
month = {12},
isbn = {978-3-540-77033-6},
year = {2007}
}
2006
Vol. 4306 Dec 2006
Springer ISBN 978-3-540-49335-8
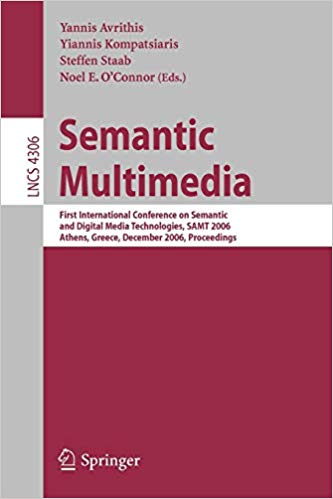
This book constitutes the refereed proceedings of the First International Conference on Semantics and Digital Media Technologies, SAMT 2006, held in Athens, Greece in December 2006. The 17 revised full papers presented together with a invited keynote paper were carefully reviewed and selected from 68 submissions. SAMT 2006 targets to narrow the "Semantic Gap", i.e. the large disparity between the low-level descriptors that can be computed automatically from multimedia content and the richness and subjectivity of semantics in user queries and human interpretations of audiovisual media. The papers address a wide area of integrative research on new knowledge-based forms of digital media systems, semantics and low-level multimedia processing.
@book{V1,
title = {Semantic Multimedia},
editor = {Avrithis, Yannis and Kompatsiaris, Yiannis and Staab, Steffen and O'Connor, Noel},
publisher = {Springer},
series = {Lecture Notes in Computer Science (LNCS)},
volume = {4306},
month = {12},
edition = {1st},
isbn = {978-3-540-49335-8},
year = {2006}
}
Unpublished
1993
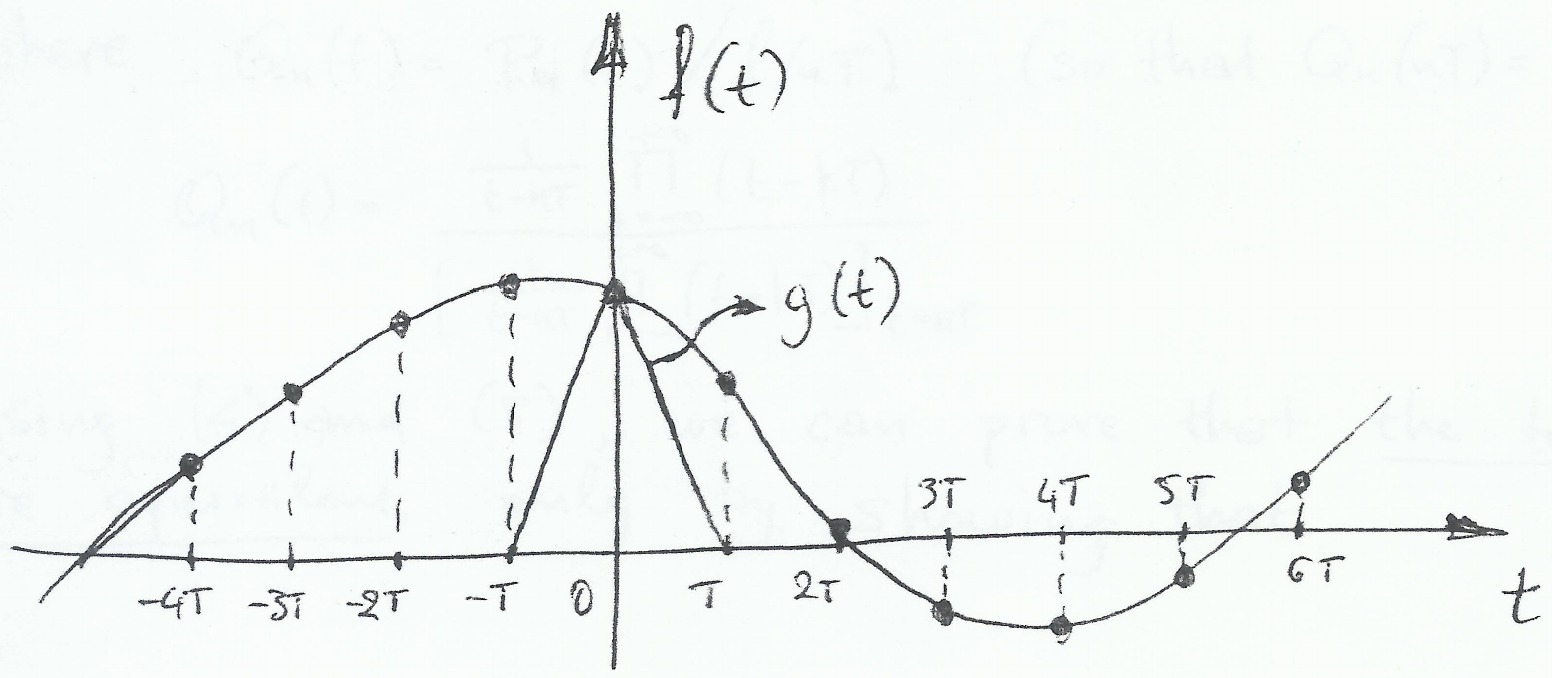
Reconstructing a continuous-time signal from a sampled sequence is usually done with a convolutional sum involving the sampling function. Another way of doing so is by Lagrange interpolation polynomials. The signal is then given by the sum of an infinite number of polynomials, each of which has roots for all integer multiples of the sampling period except for one, where it takes the value of the signal. Here we prove that the two methods are equivalent.
@unpublished{U1,
title = {Reconstruction of continuous-time signals from sampled, discrete-time sequences with Lagrange interpolation polynomials},
author = {Avrithis, Yannis},
note = {Unpublished},
month = {10},
year = {1993}
}